Document details
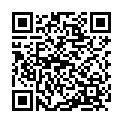
Abstract
One of the most important modalities for the observation and cataloguing of space debris is radar technology. From a radar perspective, space debris observation is particularly challenging due to the long distances between the objects of interest and the observer on the one hand and the small size of many objects on the other. One method to increase the performance of a space debris sensing system is to use a network of radars in a multi-static setup. Local coherent radar networks, whose nodes are typically not further than a few hundred metres apart, show a quasi-monostatic behaviour since the distance between the nodes is negligibly small compared to the distance to the observed objects in Low-Earth Orbit (LEO). Thus, we model the antennas of a coherent local network as one big antenna array. However, if each node of the network is equipped with an antenna array, the joint array will become sparse. The elements of each individual array are usually densely spaced, i.e. neighbouring elements are less than half a wavelength apart, exhibiting low sidelobes in their spatial/angular response. Elements of different subarrays in the joint sparse array are much further apart, which leads to high sidelobes in the joint response. In particular, the estimated Direction of Arrival (DoA) is distributed according to a network-specific function that depends on the antenna architecture as well as the relative location of the nodes. Usually, the DoA estimation error is assumed to be Gaussian distributed within a single mode around the true value. In a sparse array, this distribution becomes multi-modal, with the highest peak centred on the true DoA of the object surrounded by several secondary modes with significant amplitude, whose number and location depend on the configuration of the network. Not considering the multi-modal nature of the error can have a significant impact on the evaluation of estimation results as well as any subsequent data processing such as tracking to improve the estimates.
This paper proposes to use a Bayesian approach to process the described multi-modal DoA estimates over time. In particular, the sensor model is approximated as a mixture of Gaussians corresponding to the underlying probability distribution stemming from the architecture of the considered radar network. In the filtering recursion, the current state is first predicted according to a suitable motion model (e.g. Kepler dynamics or a local near-constant velocity approximation) and then corrected with the incoming measurement using the characteristic sensor model of the network. This procedure is applied in simulation to different examples of network configurations as a proof of concept, including a monostatic baseline case, a triangular network with three nodes, and a cross-shaped network with five nodes. The simulations show that the applied filtering is effective in resolving the modes, resulting in a DoA error that is closer to a single Gaussian distribution. The improved DoA accuracy due to the increased aperture size of the coherent network is retained, resulting in a smaller error covariance of a tracked target in comparison to the monostatic scenario.
Preview
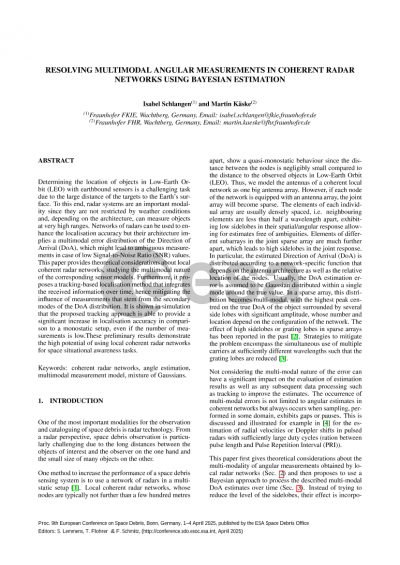
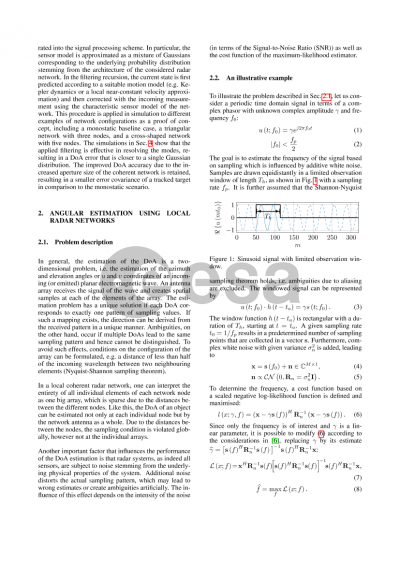
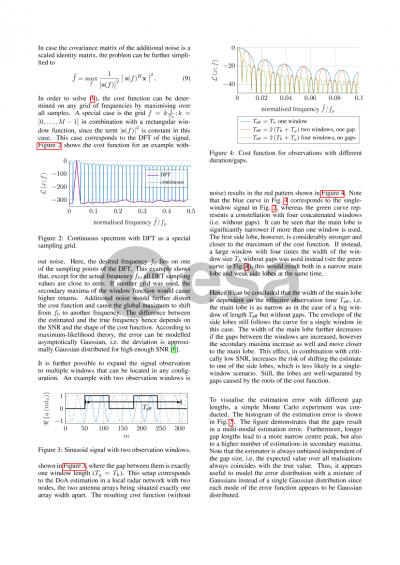
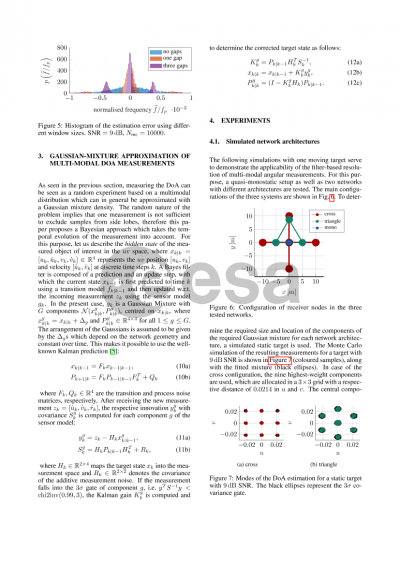
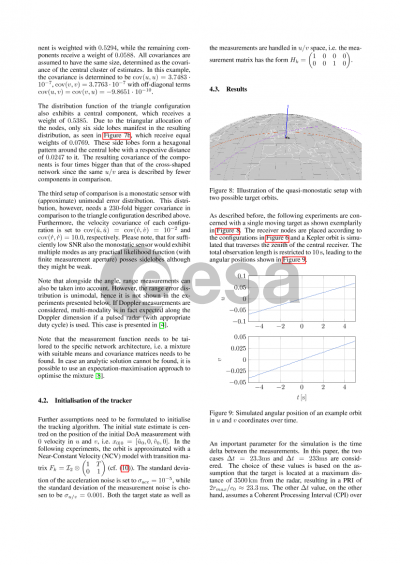
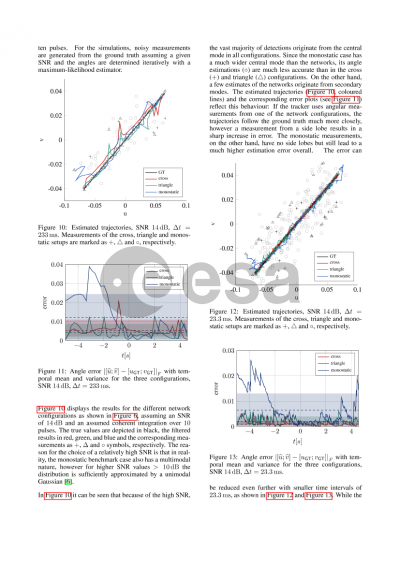
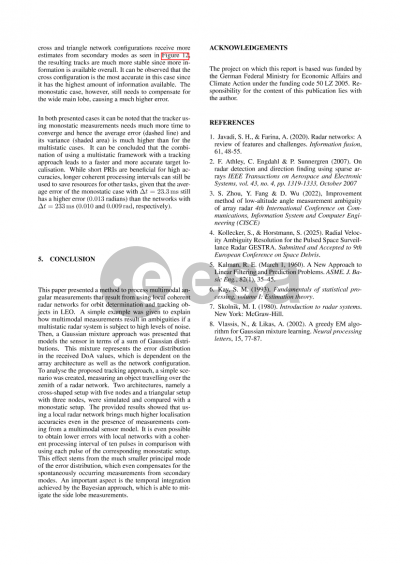