Document details
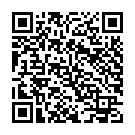
Abstract
Space weather events significantly impact satellite trajectories in low Earth orbit, introducing uncertainties from rapid variations in thermospheric density. Traditional approaches, reliant on thermospheric density models, often fall short due to inaccuracies and limited scalability. To address these challenges, we developed a Bayesian Neural Network (BNN) framework to predict orbital perturbations directly from space weather data, bypassing atmospheric models and incorporating uncertainties in space weather drivers and ballistic coefficients.
Building on this, we are iterating toward a Graph Neural Network (GNN) framework enhanced by Graph Bayesian Aggregation (GBA) to better model spatial correlations and interdependencies across orbital populations. The approach uses a two-step process: debris, with simpler and more predictable orbital behaviors, is first modeled using the BNN as a high-frequency subset of data. These predictions are then integrated into the GNN, which represents how debris covariates with other objects in orbit, enabling predictions of how other objects’ trajectories evolve based on these relationships. This allows for real-time updates of object states across the tracked catalog, propagating debris predictions to satellites with more complex dynamics, including those performing intentional maneuvers. Additionally, by analyzing changes in adjacency relationships (covariance and correlation) over time, the GNN framework can detect anomalies or maneuvers, improving situational awareness and collision avoidance.
The framework further extends to thermospheric density inversion, where debris orbital decay patterns are used to estimate density distributions as a data-driven alternative to traditional models. This iterative work aims to develop scalable, uncertainty-aware solutions for satellite and debris trajectory management, addressing the growing challenges of orbital congestion and sustainability.
Preview
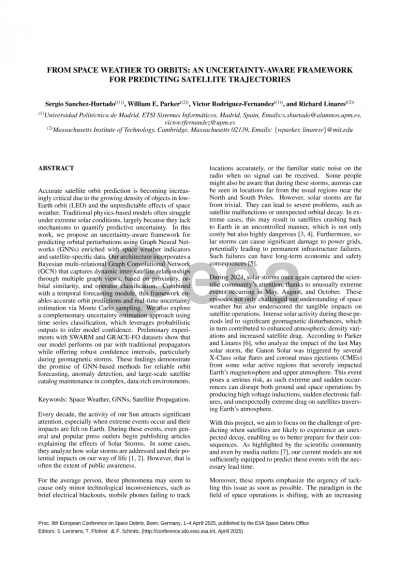
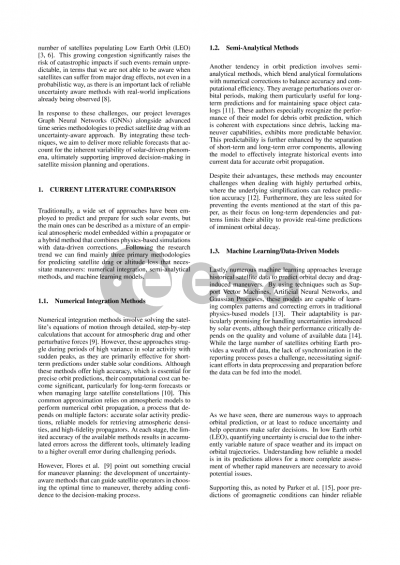
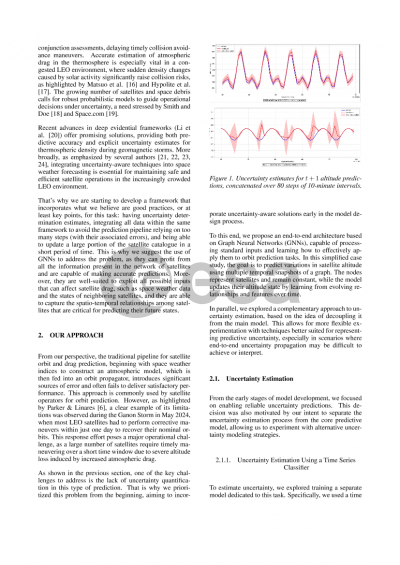
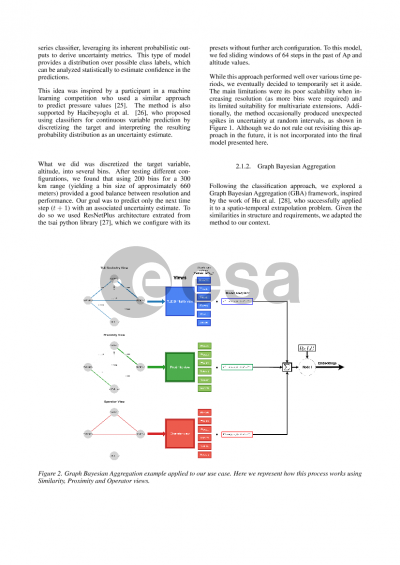
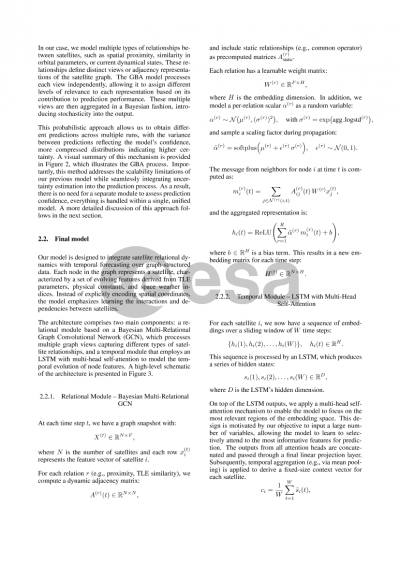
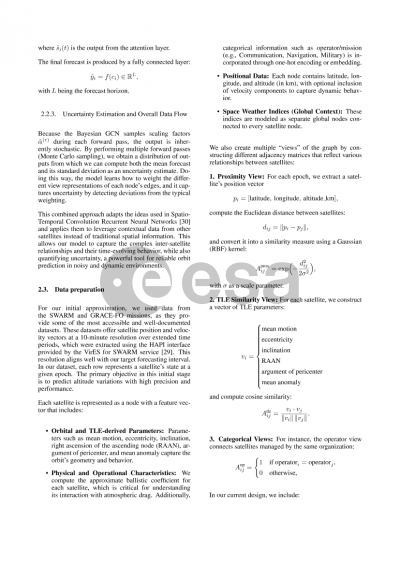
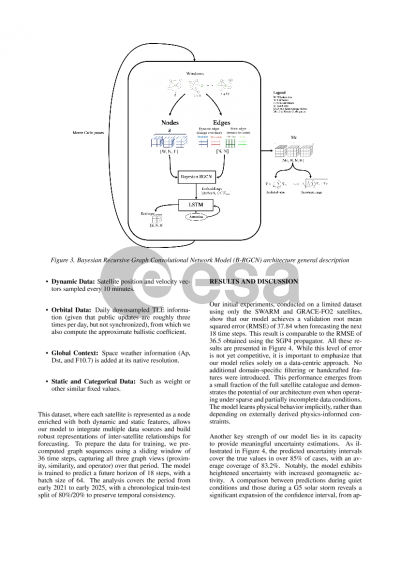
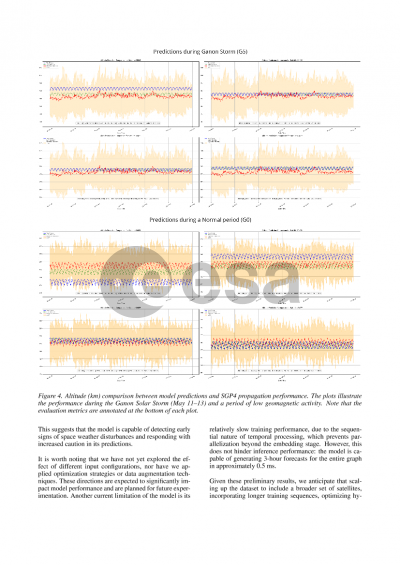
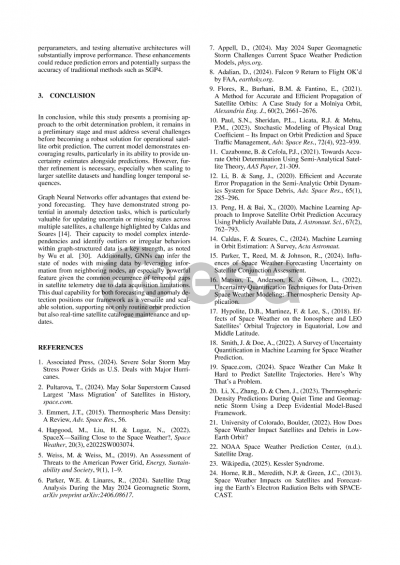
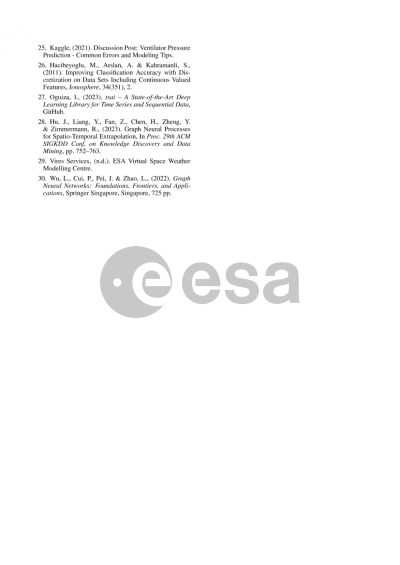