Document details
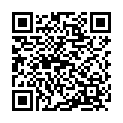
Abstract
The increasing density of objects in space has motivated improvements in the context of Space Situational Awareness (SSA) and a need for effective orbital traffic management using both ground and space-based sensor data. In this context, accurately characterizing resident space objects (RSOs) is essential for understanding their features and predicting their behaviour. Ground-based optical technologies offer a cost-effective means to identify and characterize RSOs by measuring variations in their brightness, known as “light curves,” which, when combined with positional data, provide an added value enhancing civil and military SSA applications.
Deimos owns and operates a network of ground-based optical sensors capable of producing large volumes of data every night in the form of light curves through high-frequency observations. The analysis of this photometric data collected under different visibility conditions allows the operator to characterize different features of an RSO, such as its shape, size, rotational rate, and attitude studying the variation of their brightness during the night. To automatically manage this extensive information efficiently and to analyze the nonlinear relationships that traditional analytical methods struggle to handle, machine learning (ML) techniques (in particular neural networks), have proven to be a powerful tool. For this reason, both classification and regression algorithms are used in this study to process and interpret photometric data and to finally predict RSO features.
Despite the large number of light curves potentially generated by sensors, their availability is usually limited due to weather, campaign plans, and other aspects. Thus, a high-fidelity light-curve simulator has been used to support the training of ML algorithms. The training dataset includes satellites with simple geometries such as spheres, boxes, boxes with panels, and cylinders, with different sizes and rotational status, observed across various orbital regimes. This data heterogeneity helps ML models to learn the relationships between brightness, object orientation, geometrical visibility, and other factors. The combination of simulated and real data allows the training process to be more efficient. While the former constitutes the backbone of the algorithm, the latter ensures that networks are adequately learning from real cases. Raw data is subjected to preprocessing steps to eliminate noise and anomalous measurements before being ingested or analyzed by the trained neural networks. For each characteristic, two Neural Networks are developed, one with data from LEO objects and one with GEO. After a thorough validation, the initial results demonstrated that light curves hide certain patterns that, if properly identified by the networks, can help predict RSO characteristics with high accuracy.
This work demonstrates the effectiveness of artificial intelligence (AI) in enhancing the characterization of Earth-orbiting objects through real photometric data analysis. Our findings indicate that AI not only improves the accuracy of object characterization but also enables the identification of previously unrecognized patterns in the data. This work paves the way for more efficient monitoring and management of the space domain, contributing to the broader field of space situational awareness and ensuring the safety of orbital operations.
Preview
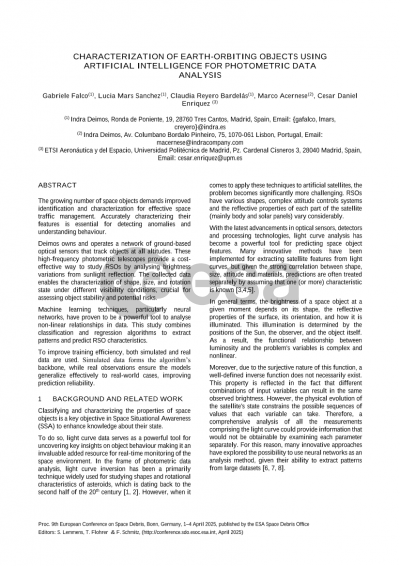
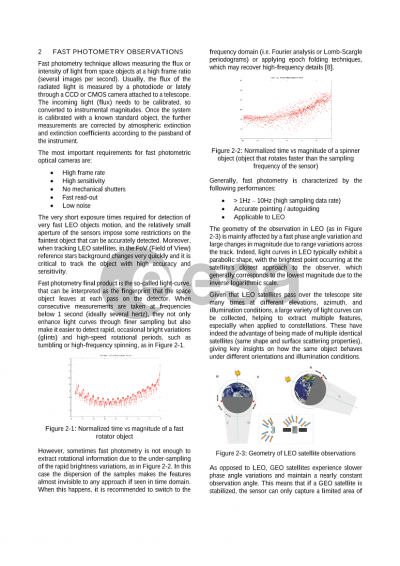
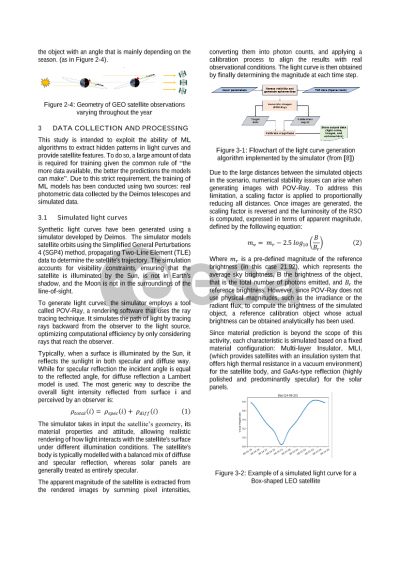
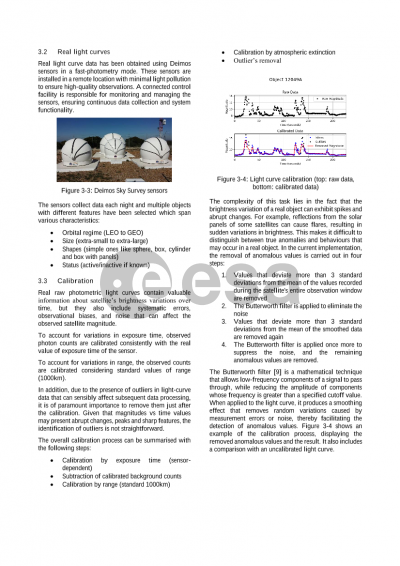
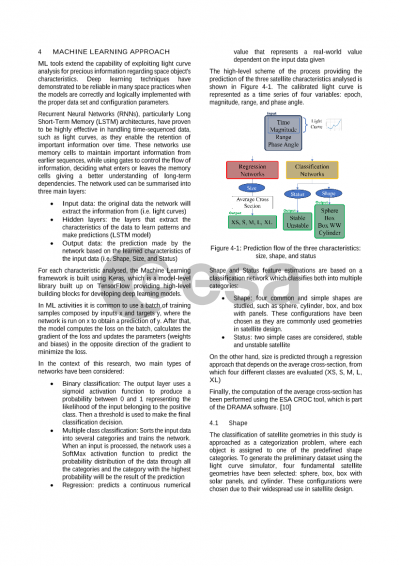
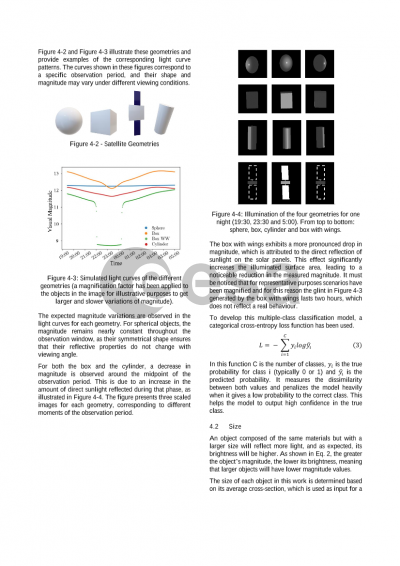
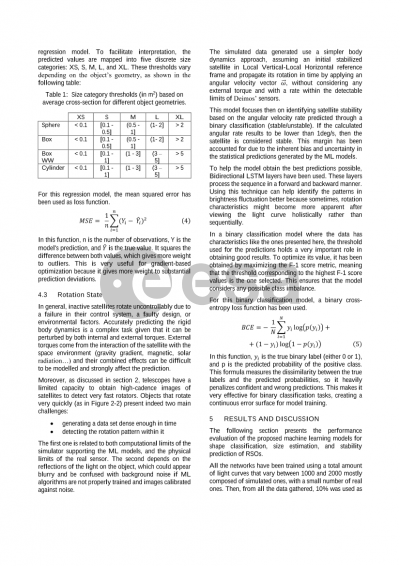
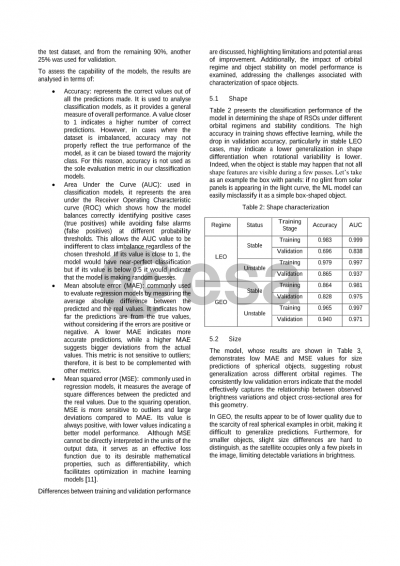
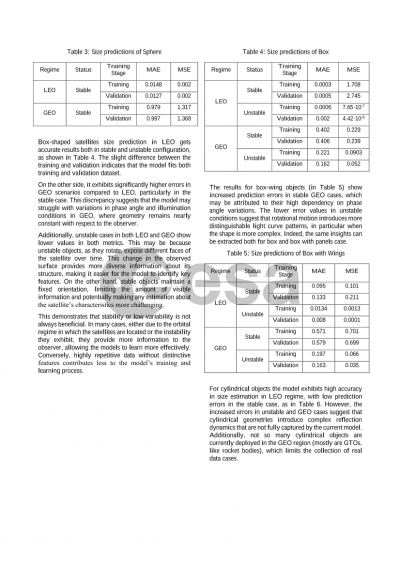
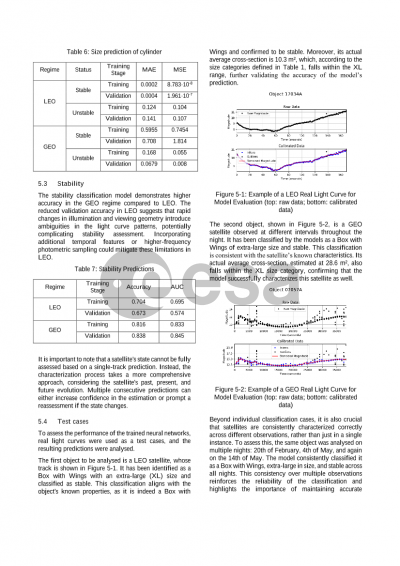
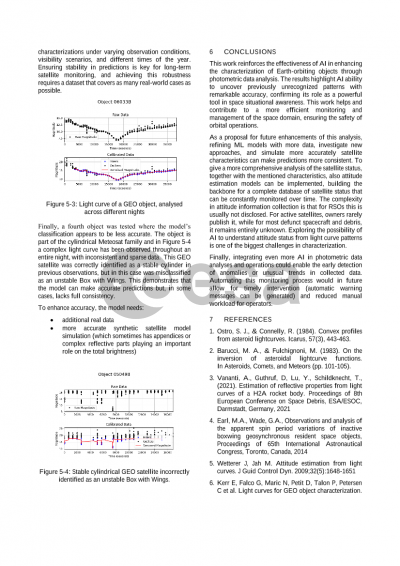
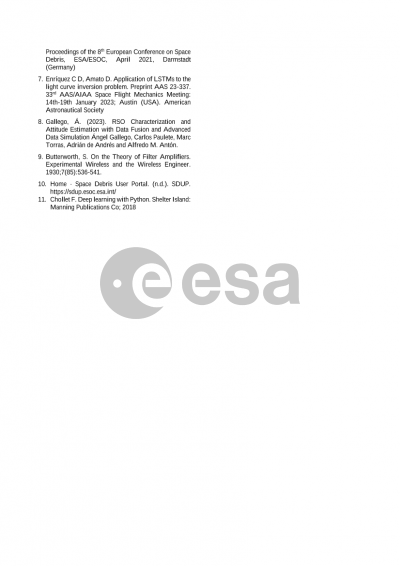