Document details
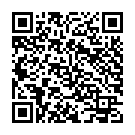
Abstract
Position and velocity covariances are critical for assessing collision probabilities in space operations; however, they are often unavailable or questionably estimated by flight dynamics systems. Spacecraft operators frequently encounter “black box” systems that do not readily output covariance matrices, or they may receive covariances tied to the Orbit Determination (OD) epoch lacking time history or customization options regarding reference frames and parameters. In addition, organizations providing Space Situational Awareness (SSA) information are hesitant to disclose positional accuracy metrics. As a result, reliable covariance information is scarce.
To address these limitations, we embrace the concept of synthetic covariances, computed directly from a given time series of position and velocity. Traditional approaches for constructing synthetic covariances are mainly based on standard overlap comparisons. In this regard, there are methods based on predefined error functions and covariance matrix eigenvector reference frames [1]. Recently, a novel digital statistics method for 6x6 covariance uncertainty modeling was presented drawing on statistics from time and argument-of-latitude bins on an object-by-object basis [1].
Alternatively, we proposed to pose as an estimation problem where the state vector is used in a way analogous to an observation. The solution approach consists of an initial filtering of the available ephemeris with a priori noise information, that is adaptively adjusted for the residuals to be statistically consistent. The estimation of noise covariance matrices in the optimal filtering problem has been the subject of research in the last 50 years [2], mainly in the context of KF and linear invariant systems. However, some of them have been extended to nonlinear problems. In general, the methods can be classified into four groups according to the approach [3]: Bayesian methods, correlation methods, maximum likelihood methods and covariance matching methods. In this work, we will review some of the possible approximations [4] applied to this specific problem. In this way, it is possible to generate a state estimate, consisting of the expected state and its uncertainty represented by the covariance, compatible with the dynamic model and the filtering method used, solely from the time series of the state vector.
We will evaluate the performance of this new technique in a similar way to previous work [1]. Two-Line Elements (TLEs) and high-accuracy numerically integrated ephemerides will be used for comparison. This comparison will use high-quality reference orbits, including those from calibration spheres, Wide Area Augmentation System (WAAS), and Global Navigation Satellite System (GNSS) satellites.
References:
1. Gist, R.G., Oltrogge, D.L., and Alfano, S. Synthetic Covariance Production Using a New Digital Approach, 4TH International Conference on Space Situational Awareness (ICSSA) Daytona Beach, FL, USA. IAA-ICSSA-24-0000
2. Zhang, Lingyi, David Sidoti, Adam Bienkowski, Krishna R. Pattipati, Yaakov Bar-Shalom, and David L. Kleinman. “On the Identification of Noise Covariances and Adaptive Kalman Filtering: A New Look at a 50 Year-Old Problem.” IEEE Access 8 (2020): 59362–88. https://doi.org/10.1109/ACCESS.2020.2982407.
3. Duník, Jindřich, Ondřej Straka, Oliver Kost, and Jindřich Havlík. “Noise Covariance Matrices in State-Space Models: A Survey and Comparison of Estimation Methods—Part I.” International Journal of Adaptive Control and Signal Processing 31, no. 11 (2017): 1505–43. https://doi.org/10.1002/acs.2783.
4. Dunik, Jindrich, Oliver Kost, Ondřej Straka, and Erik Blasch. “Covariance Estimation and Gaussianity Assessment for State and Measurement Noise.” Journal of Guidance, Control, and Dynamics 43 (November 7, 2019): 1–8. https://doi.org/10.2514/1.G004348.
Preview
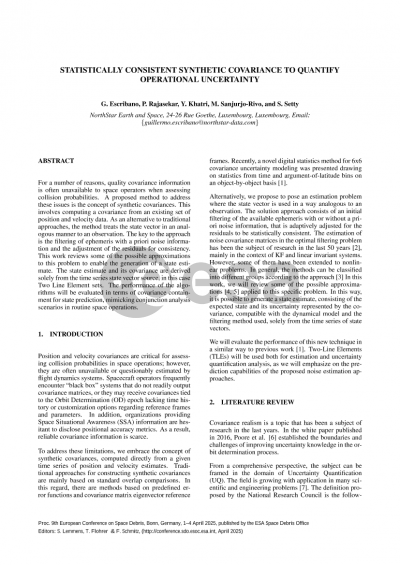
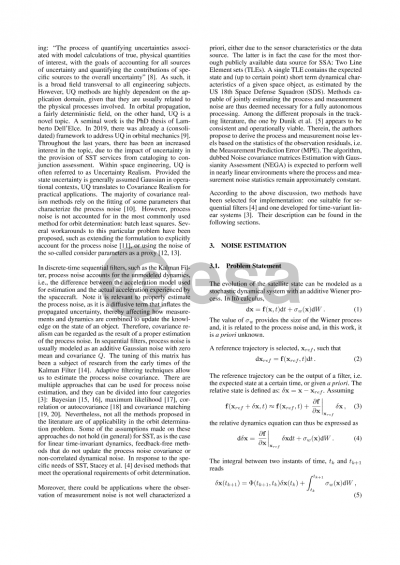
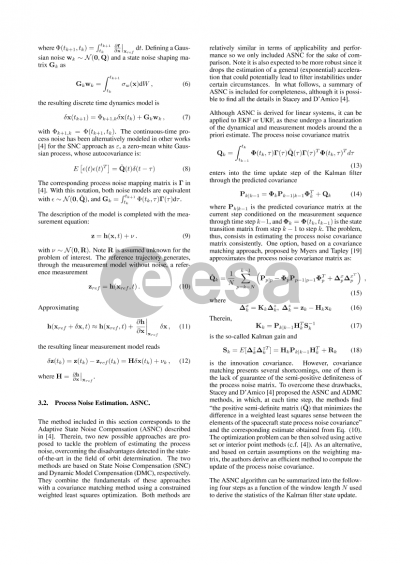
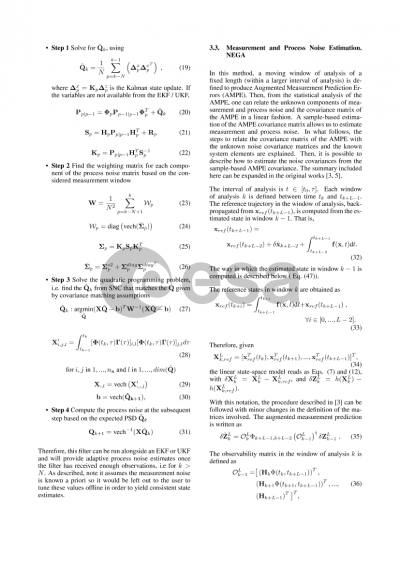
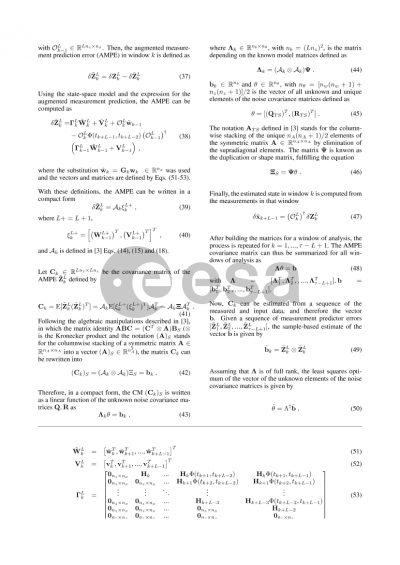
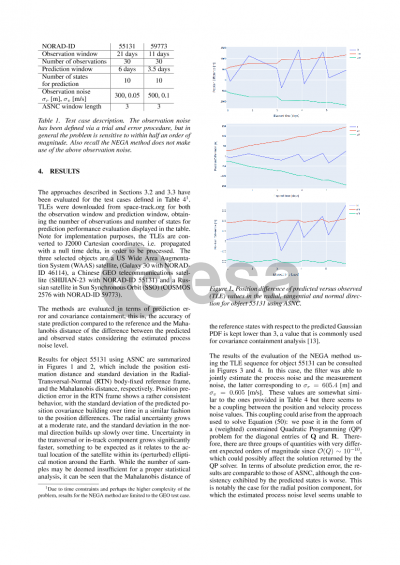
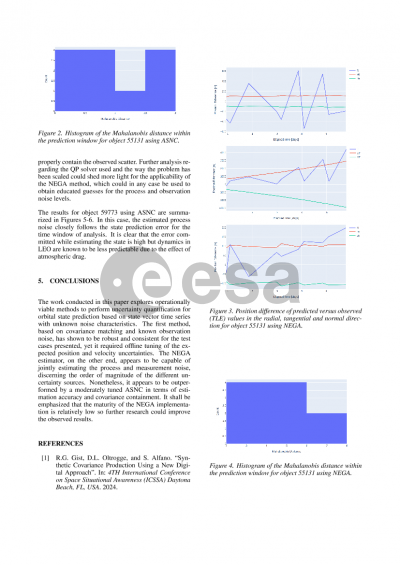
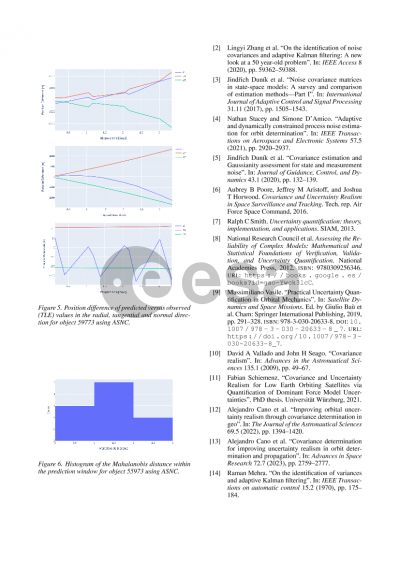
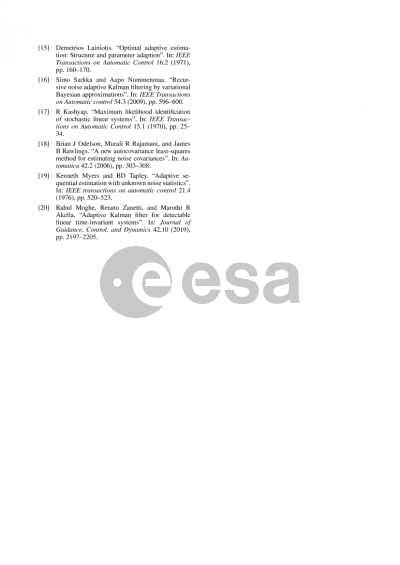