Document details
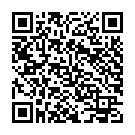
Abstract
Modeling orbits in Low Earth Orbit (LEO) is a cornerstone of modern space operations but presents significant challenges due to the inability to reliably maintain Gaussian assumptions during numerical propagation. The research presented here is the result of observations made during our project to develop the next-generation drag modeling framework that accurately characterizes atmospheric density uncertainty due to space weather through a physics- and data-driven approach. Supported by the Intelligence Advanced Research Projects Activity (IARPA) Space Debris Identification and Tracking (SINTRA) program and the Office of Space Commerce (OSC), we are developing the stochastic Unscented Transform (SUT), a mathematical formulation designed to capture the joint statistics of probabilistic atmospheric density models and their probabilistic drivers or inputs. During the development process, we observed an intriguing trend: the 2-norm of the position standard deviation exhibited an unexpected dip when a LEO orbit was numerically propagated beyond three days. This prompted a deeper investigation into the numerical stability and Gaussian assumptions underlying the propagation framework.
It has been observed that the non-physical trend emerges when the state covariance is orthogonalized and sigma points are recomputed at fixed cadence, indicating the limitations of the underlying Gaussian assumption. This setup has been employed to exploit the numerical instability such that the half-life in atmospheric density can be modeled. The presence of non-physical trends in position and velocity uncertainties during propagation provides a basis to investigate deviations from Gaussian assumptions, specifically the divergence of sigma points from the underlying Gaussian distribution.
Firstly, normalization techniques are applied to improve numerical conditioning. Position and time units are scaled with respect to the Earth’s radius and the orbital period of the given LEO orbit, respectively. While this adjustment leads to measurable improvements in the conditioning of the covariance matrix, it does not fully eliminate the instability. Consequently, the standard Cholesky decomposition, used in the covariance orthogonalization process, was replaced with Singular Value Decomposition (SVD). The use of SVD significantly enhances numerical stability, allowing for an increase in the covariance-orthogonalization update cadence from 5 minutes to 20 minutes, without introducing excessive instability.
However, the analysis revealed that for update cadences exceeding 20 minutes, residual non-physical trends persist. To further understand these trends, 2000 Monte Carlo simulations were run to replicate the propagation scenarios and evaluate the impact of non-Gaussian deviations in the covariance. The Monte Carlo results confirmed that the two-sigma point configuration results in failing Gaussian assumptions as the sigma points diverged. This divergence causes the covariance propagation to become increasingly unstable, leading to the observed anomalies in the position and velocity standard deviations.
This comprehensive investigation underscores the challenges of maintaining numerical stability and preserving Gaussian assumptions in the complex dynamics of LEO modeling. The findings highlight the need for robust techniques to address the divergence of sigma points and mitigate its impact on covariance propagation. By improving covariance realism, this research enhances the accuracy of orbit prediction models, which is crucial for applications such as long-term propagation, collision avoidance, and high-precision orbit determination.
Preview
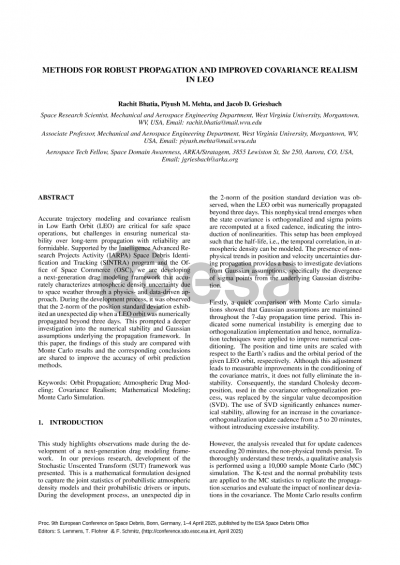
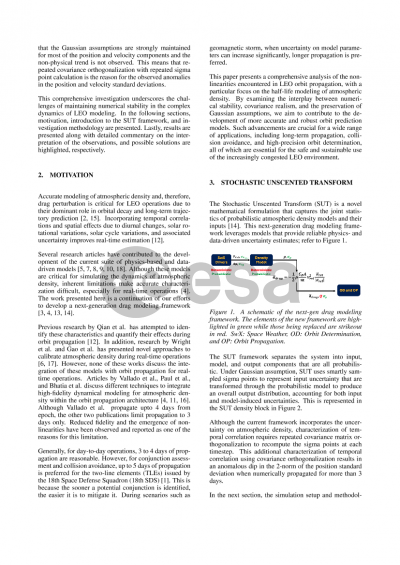
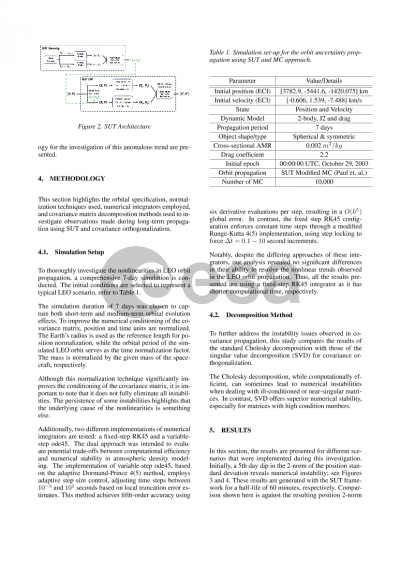
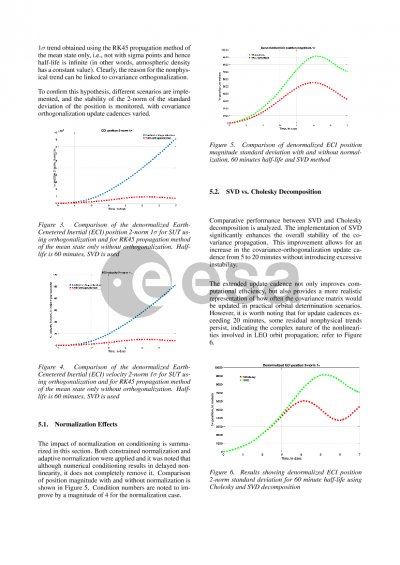
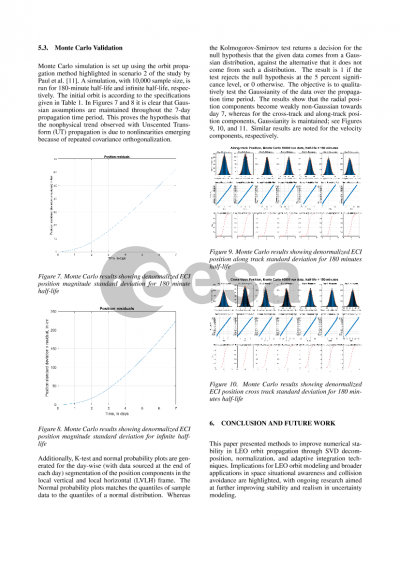
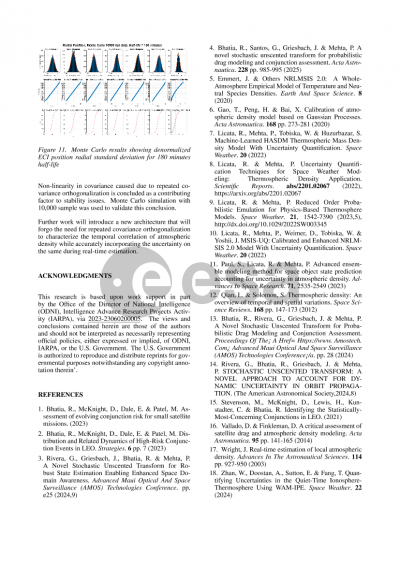