Document details
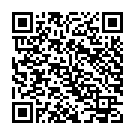
Abstract
Catalogue build-up and maintenance are fundamental to Space Situational Awareness (SSA) services, ensuring updated databases of all observed space objects, including operational satellites or space debris, with their current and predicted orbit estimates. The accuracy and reliability of the catalogue is crucial for the provision of other SSA services such as conjunction assessment, fragmentation detections, re-entry predictions, and manoeuvre detection, all essential for the safety and sustainability of the space environment.
The current space context, driven by the democratisation of space and the rise of commercial activities, heightens the challenges of catalogue build-up and maintenance. Associating new observations with existing objects becomes ambiguous in overly congested regions, which can lead to poor track association performance. This strains the reliability of the subsequent processes of orbit determination and propagation. Poor catalogue estimation and prediction further deteriorate data association performance, aggravating data scarcity and ultimately impacting SSA services. Therefore, it is needed to analyse and develop robust and reliable methodologies for orbit determination and data association to address current and future challenges of the space environment sustainability.
The European Space Agency’s (ESA) funded project “Robust Orbit Determination for Space Debris”, led by GMV in collaboration with the University of Liverpool (UoL) and the University Carlos III de Madrid (UC3M), aims to tackle these challenges. This paper focuses on some of the most relevant results of the project.
On one hand, it showcases results from modern extensions to state-of-the-art batch least-squares estimation algorithms designed to improve robustness. These include the Huber penalty function for least-squares estimation to enhance convergence, time-dependent measurement weight to achieve smoother Lengths of Update Intervals (LUPI), and uncertainty quantification techniques for covariance realism improvement, including Stochastic Consider Parameters and alternative state representations for uncertainty assessment.
On the other hand, we evaluate the performance of sequential and particle filters in space tracking environments. Regarding sequential algorithms, we explore the family of methods that can be spawned by tuning the hyperparameters of the Iterated Posterior Linearization Filter (IPLF), going one step beyond EKF or sigma-point Kalman filters, and taking advantage of the knowledge of the value of the measurement and statistical linear regression for an improved update. Furthermore, the benefit of introducing a smoother (IPLS), defining a sliding time window to refine the estimate, is assessed. For particle filters, the standard Particle Filter (PF), Ensemble Kalman Filter (EnKF) and Nested Particle Filter (NPF) are selected for analysis in the very same scenarios representing operational environments.
In the context of the multi-target data association problem, novel approaches making use of sequential filters together with Global Nearest Neighbour and Joint Probabilistic Data Association Filter associators are investigated, and its behaviour contrasted with track-to-track correlation methods, which are already applied in this domain.
The project concludes with the development of a prototype, “Robust Orbit Determination and Data Association for Space Debris” (RODDAS), which implements promising pre-prototyped methodologies using ESA’s GODOT as the underlying astrodynamics library and Stone Soup’s library as the foundation for the development of the selected target tracking and state estimation algorithms.
Preview
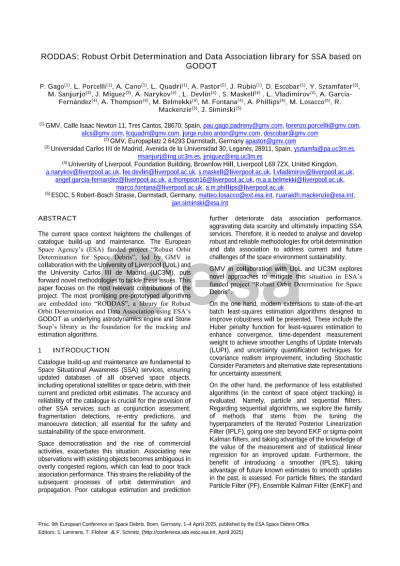
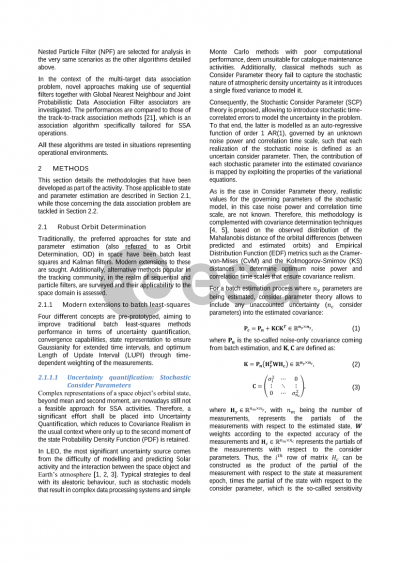
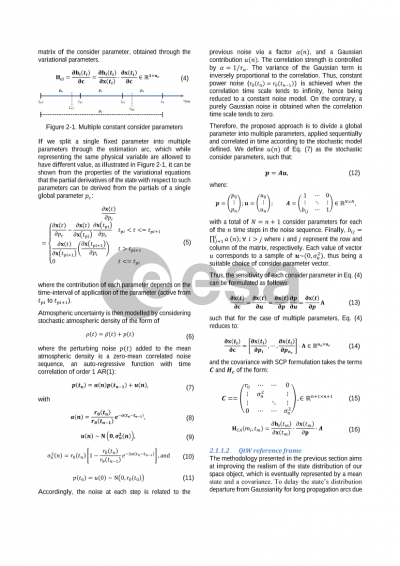
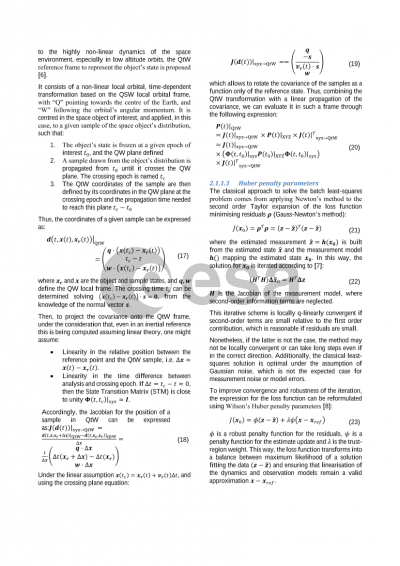
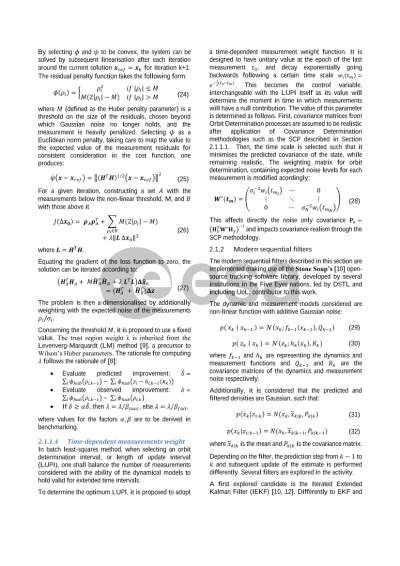
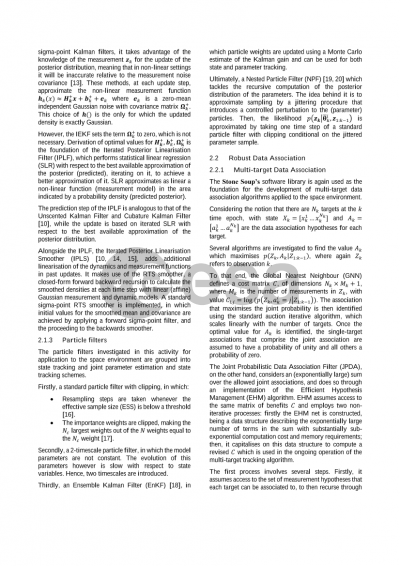
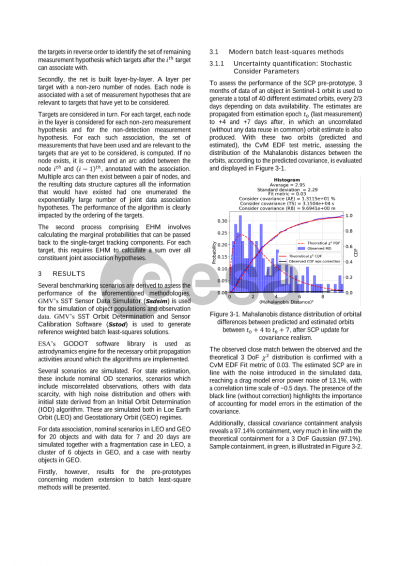
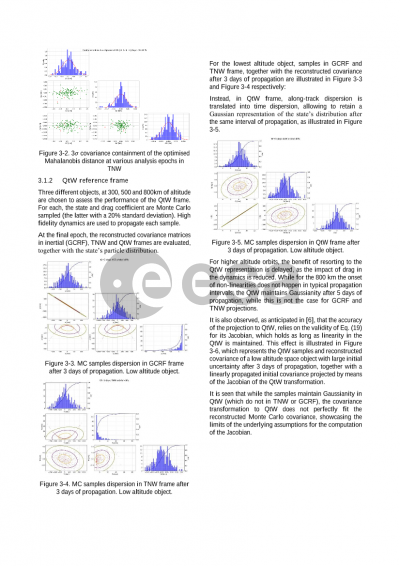
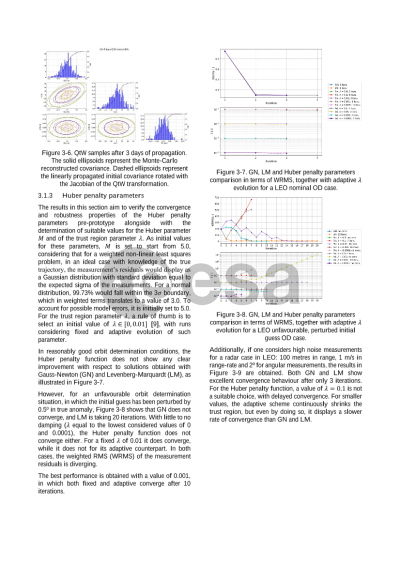
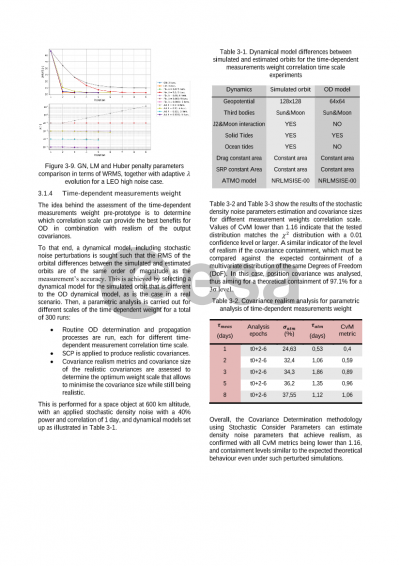
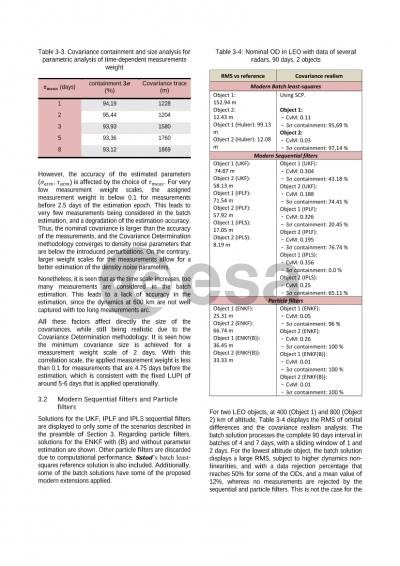
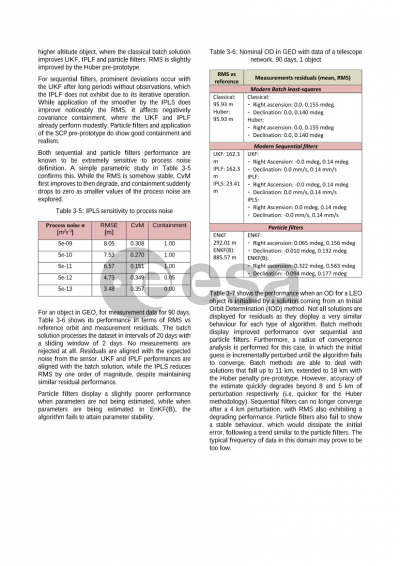
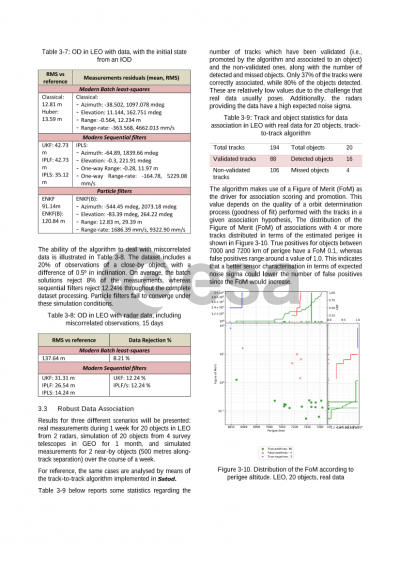
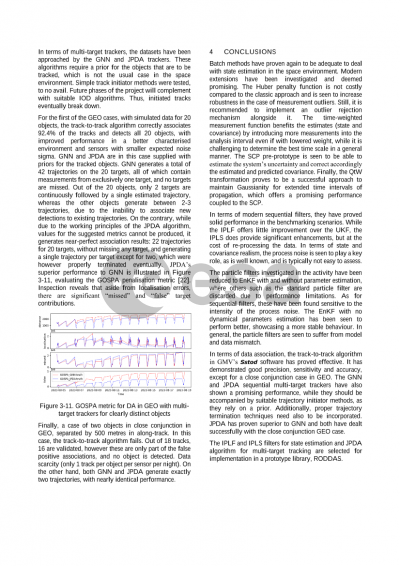
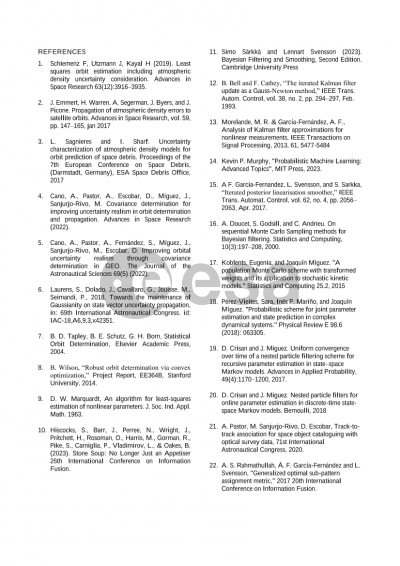