Document details
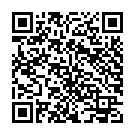
Abstract
In early 1990s, NASA and DoD collaborated on a laboratory hypervelocity impact (LHVI) test called SOCIT. These tests helped engineers and scientists to understand the effects of orbital debris impact and provided a statistical representation of the debris environment. As e technology advanced, some metallic components were replaced by carbon-fiber reinforced polymers (CFRP), Kevlar, and other high performance materials. This material composition in modern satellites have led to different expectations in fragmentation characteristics. Thus, the DebriSat project was developed and executed.
The DebriSat project was created by NASA, DoD, The Aerospace Corporation, and the University of Florida (UF) to provide characteristic data to improve orbital debris modeling capabilities. A test article representative of a modern-day LEO satellite was designed fabricated, tested, and subjected to a LHVI. The LHVI test was performed at a facility at Arnold Engineering Development Complex (AEDC) in Tennessee. The post-impact test system and fragments were collected and transported to UF for fragment identification and characterization. During characterization, fragments are individually assessed for material, shape, mass, and size (cross-sectional area, volume, and characteristic length).
The UF team established various dimensional analysis instruments for material assessment and interconnected these instruments within a database referred to as the Debris Categorization System (DCS). Fragments that are assessed for material classes to be metallic are labeled with a placeholder “METAL”. Fragment volumes are obtained after imaging and used to compute densities for identifying the METAL fragments as either aluminum (AL), titanium (TI) or stainless steel (SS). Around 2022, a bias related to mislabeling of metal fragments was identified in the DCS – it appeared a set of stainless steel fragments were labeled as titanium. After a survey, it was determined that the bias arose from the thin fragment processing approach for volume computation. To resolve this bias, an algorithm was developed to compensate the overestimation of volume and utilized to determine the stainless steel and aluminum fragments in the metal dataset. Also, all TI fragments were identified and were no longer part of the METAL dataset.
To minimize bias in selecting metal labels (AL, TI and SS), a supervised machine learning (ML) model using Logistic Regression was trained on the newly defined material classes derived from the relabeling process. When the Logistic Regression Model (LRM) was applied to the uncategorized METAL fragments, it achieved over 90% accuracy in detecting SS fragments. However, it was unable to achieve comparable accuracy for AL labeled fragments. This was due to presence of other materials such as multi-layer insulation (MLI), glass, epoxy and CFRP in the lower density region of the METAL dataset. Consequently, a training set was constructed from a revised low density fragment (AL, MLI, glass, epoxy, CFRP) to train a ML model using a Decision Tree algorithm, aimed at detecting fragments that are biased with METAL label within the low-density region. This manuscript presents the results of a ML model that is capable of detecting a broad range of single-material fragments.
Preview
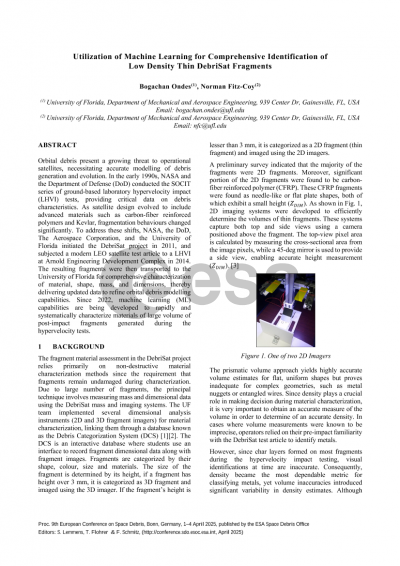
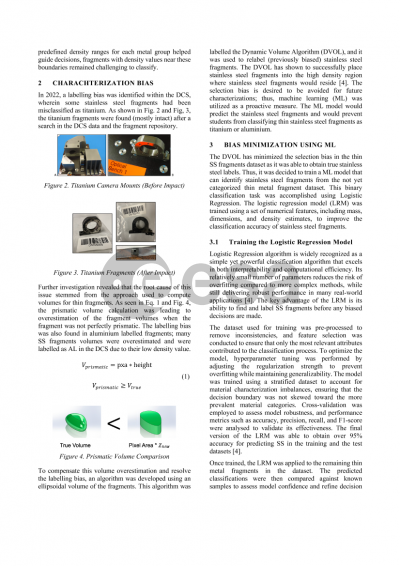
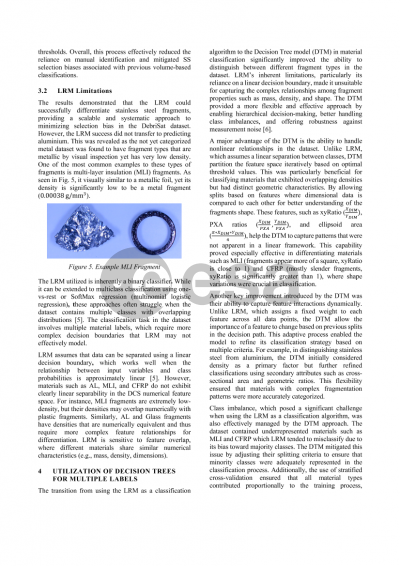
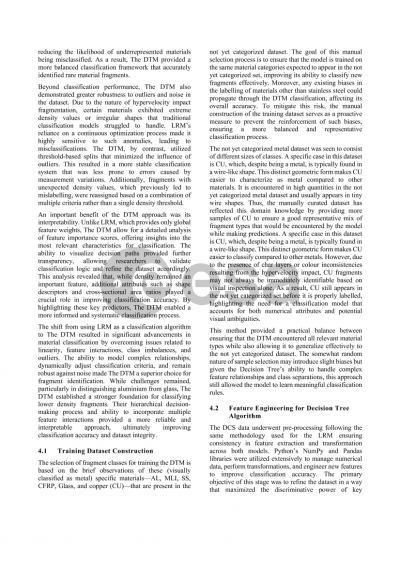
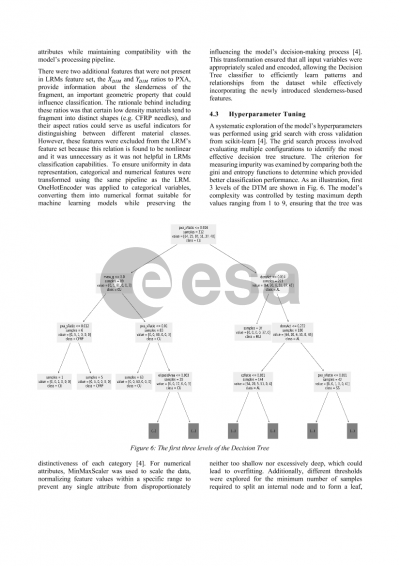
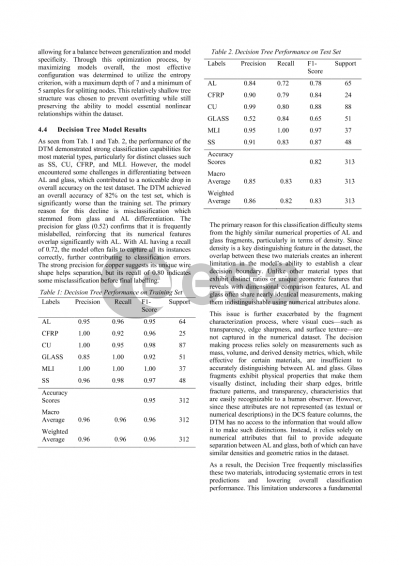
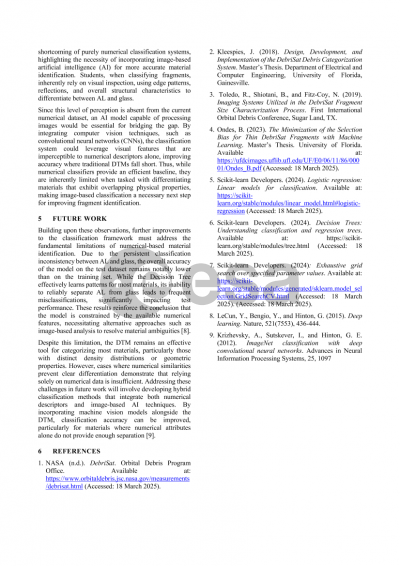
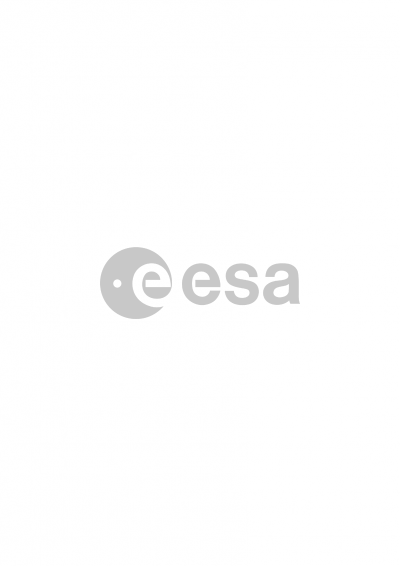