Document details
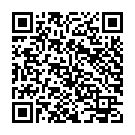
Abstract
The exponential increase in satellites launched into Earth’s orbits over the past decade has led to a significant rise in space debris within critical orbital regions used for commercial and scientific missions. This growing congestion in space traffic demands immediate action and has become a critical focus within the fields of Space Surveillance and Tracking (SST) and Space Environment Preservation (SEP). In this context, merely determining the orbits of inactive resident space objects (RSOs), such as debris fragments or decommissioned satellites, is insufficient to safeguard operational satellites and ensure the long-term sustainability of the space environment. This work focuses on estimating the attitude of inactive RSOs using photometric measurements (light curves) from ground-based optical sensors. Accurate knowledge of an object's attitude is essential for the efficient planning of Close-Proximity Operations (CPO), such as Active Debris Removal (ADR) and In-Orbit Servicing (IOS) missions. Moreover, attitude information improves the accuracy of orbit determination, refines the estimation of collision probability, and facilitates the verification of a satellite's successful transition into contingency mode.
More specifically, the present study addresses the estimation of the attitude of inactive RSOs with known geometric and optical properties. The light curve inversion problem for attitude estimation presents two main challenges: the highly non-linear nature of the measurement model and the intrinsic ambiguity in the measurements, notably the potential existence of multiple attitude solutions for a given light curve. To overcome these challenges, an advanced light curve inversion method based on particle filtering is introduced. The particle filter is an approximate Bayesian estimator that represents probability densities through a weighted set of samples, enabling the effective handling of multimodal probability density functions (PDFs). Therefore, it can overcome the limitations found in other estimation methods, such as the Least Squares Method (LSM) and the Unscented Kalman Filter (UKF). Photometric observations are simulated using GMV's Grial tool, a state-of-the-art high-fidelity simulator that utilises OpenGL to compute the contributions of reflected light from each illuminated and visible pixel on the three-dimensional model of the RSO, while accurately accounting for shading interactions among its various components.
To develop a particle filtering method suitable for real operational scenarios, efforts are directed towards minimising computation time while preserving accuracy in attitude estimation. To this end, the Adaptive Pseudo-Batch Particle Filter (APB-PF) is introduced. This method relies on adaptive particle allocation in regions of high probability density using optimisation methods, combined with Kalman filtering and clustering techniques, to enhance both accuracy and computational efficiency. Simulated scenarios are developed to demonstrate the advantages of the proposed approach over traditional sequential particle filtering methods. The results of these analyses show excellent estimation accuracy for light curves simulated under perfect knowledge of the RSO’s geometric and optical properties. The robustness of the APB-PF is further assessed by estimating the attitude under uncertain knowledge of the RSO characteristics —an essential step before analysing real light curves. In this case, the results indicate the method's promising capability to handle real light curves in operational scenarios.
Preview
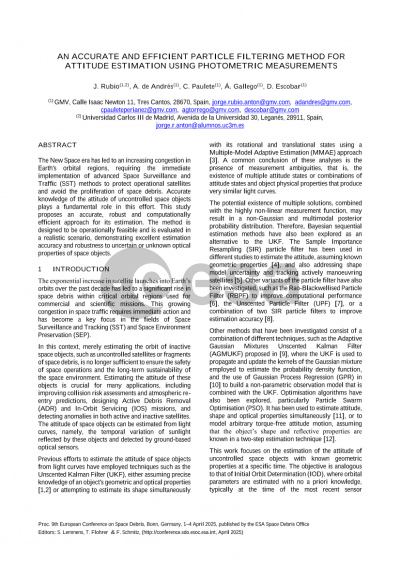
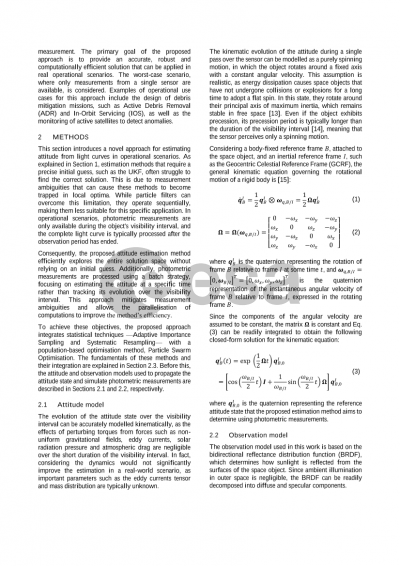
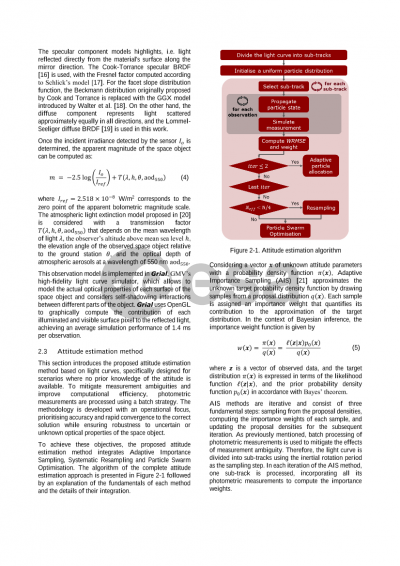
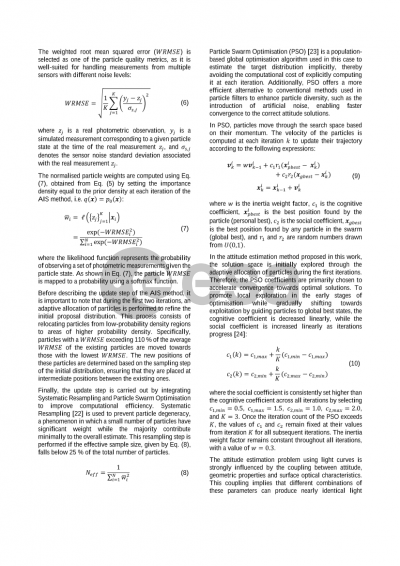
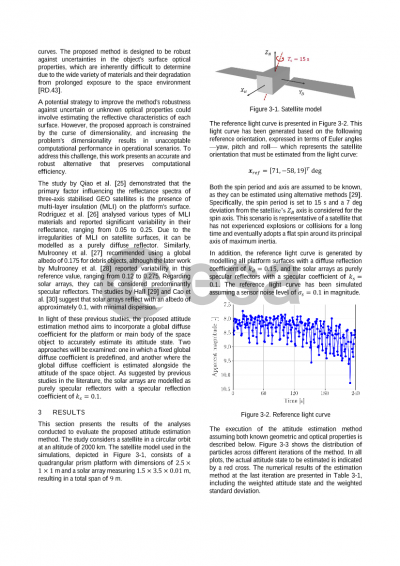
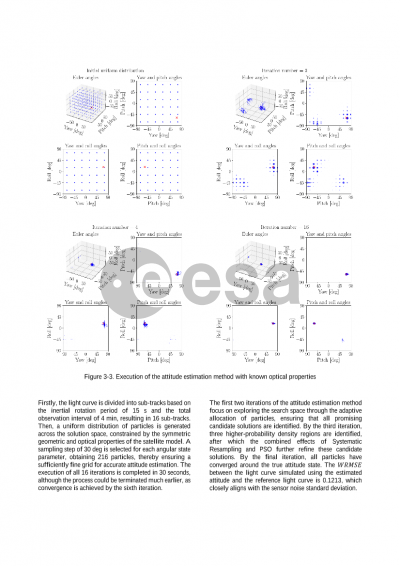
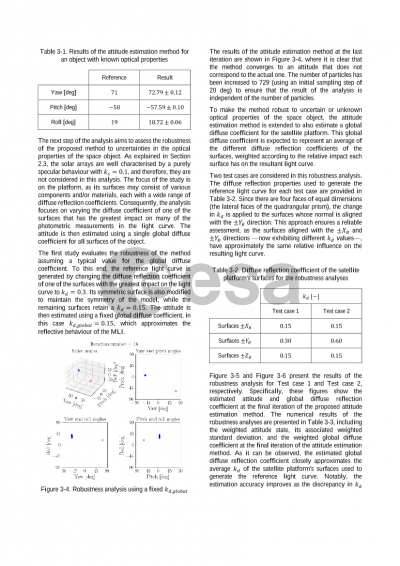
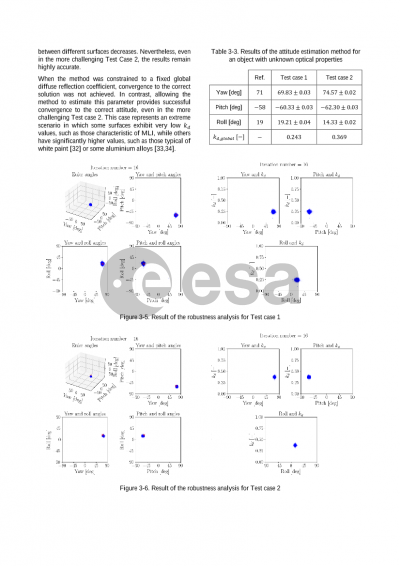
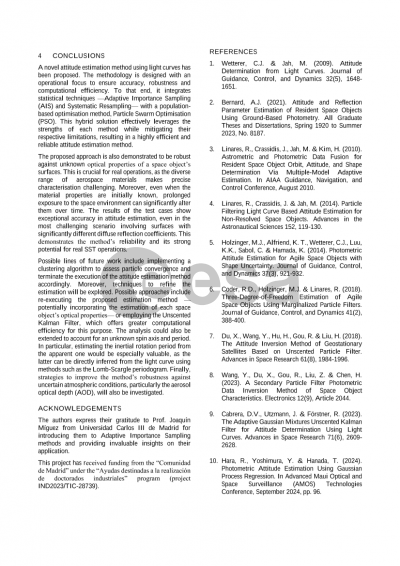
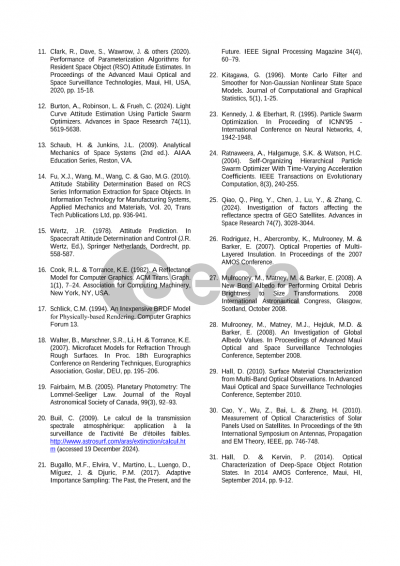
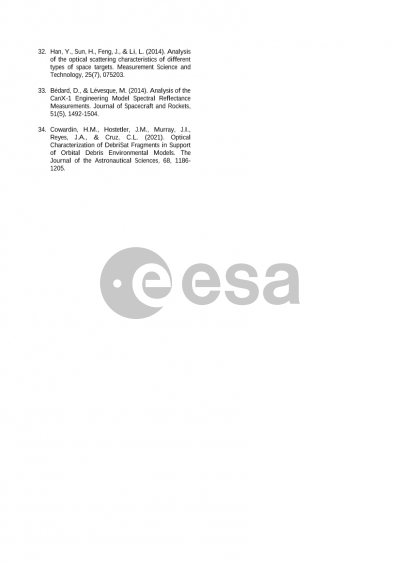