Document details
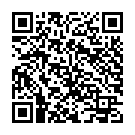
Abstract
The problem revolving around the status of the near-Earth environment is complex and ever-changing, requiring a collective effort to characterise, model, and monitor the evolution of the resident space object population from both institutions and industry. Reaching a comprehensive Space Situational Awareness has thus become of utmost importance, due to the immediate effects on every phase of a space mission, from its design to the operations foreseen after its deployment.
A fundamental aspect involved in the surveillance and tracking of space objects is taking operational ones into account in terms of their manoeuvring capabilities. Actively controlled objects can, in fact, hamper the correlation of new tracks, leading to false or missed associations, and reduce orbit determination accuracy, introducing unmodeled contributions to the assumed dynamics. Given the rapidly increasing subset of manoeuvring targets in catalogued populations (e.g., large satellite constellations performing collision avoidance manoeuvres), this concept is becoming key to improving the robustness of measurement processing pipelines.
One way of addressing the problem consists in characterising the pattern of life of operative objects in terms of manoeuvres in order to understand how likely it is for a target to be actively controlled within a specific time frame. The main showstopper identified in the literature is the shortage of manoeuvre histories used to generate labels for supervised learning algorithms. This severely reduces a method’s generalization capability.
The current work aims to overcome this limitation by resorting to unsupervised learning techniques commonly used for anomaly detection purposes to identify manoeuvres with no prior information on the target’s control history. An autoencoder built from Long Short-Term Memory cells is proposed, feeding on fixed-size sequences of orbital elements extracted from public Two-Line Element files. With this kind of architecture, the objective of the training phase is to fit a distribution representing a target’s nominal behaviour, so that a high anomaly score is returned by the model in case an out-of-distribution sample is provided as input. The proposed application considers ballistic motion as the ‘normal behaviour’ while identifying manoeuvring arcs as out-of-nominal samples. To accomplish this, for every active object under analysis, a set of debris with similar orbit geometry is selected and used to train the network. The inference phase is therefore performed on the reference active object and sequences of anomaly scores are retrieved. A k-means clustering step is used to identify clusters of anomaly score values, distinguishing between a background value, linked to the orbital differences between the debris population and the target, and peak values correlated to manoeuvres. A threshold is finally computed based on the cluster with lower anomaly scores, enabling the detection of manoeuvring events.
The technique has been tested on active targets from the International Laser Ranging System database, with publicly available orbital data history and past manoeuvre events. The outcome of this performance assessment shows promising and consistent results on the whole set of objects, proving that the approach represents a convenient first step to characterise a satellite’s pattern of life.
Preview
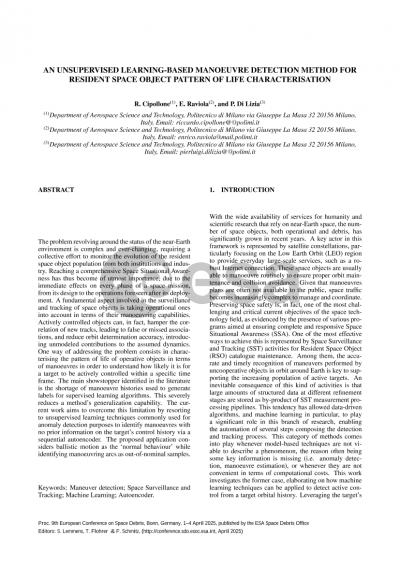
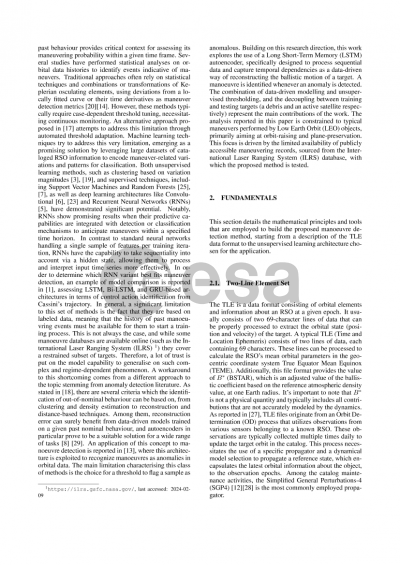
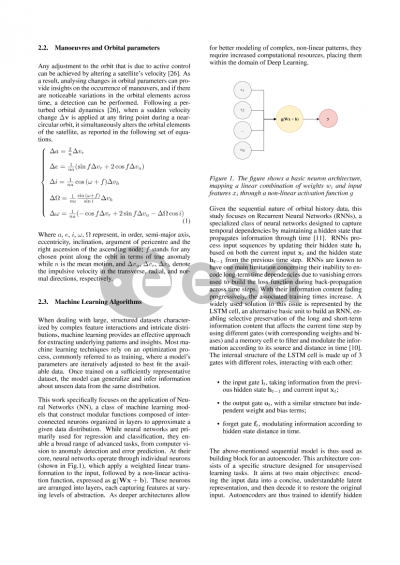
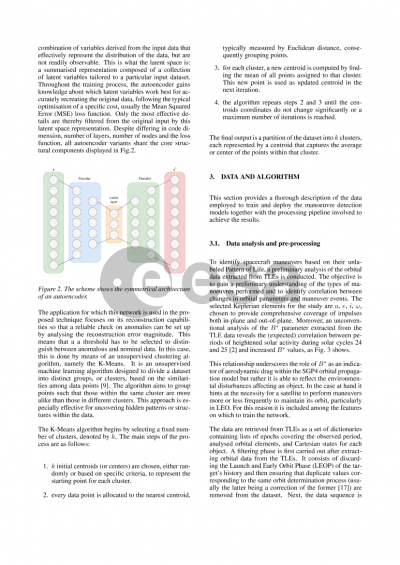
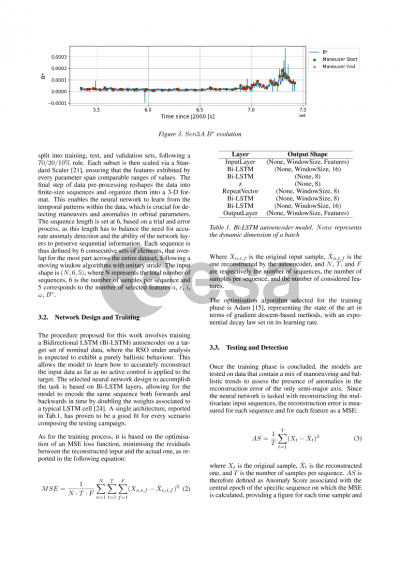
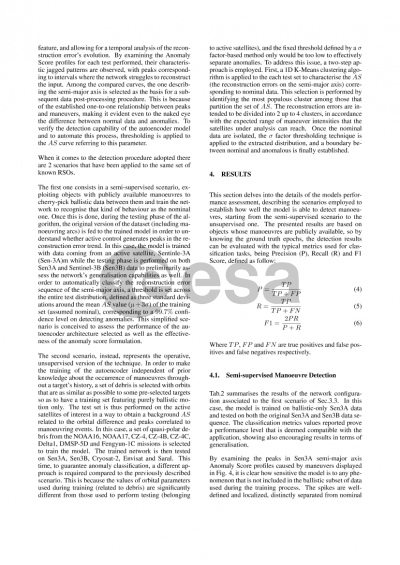
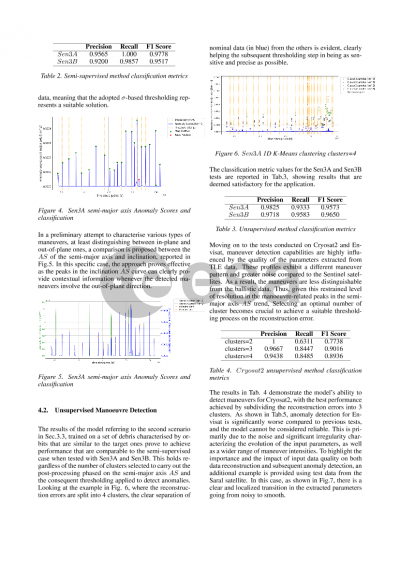
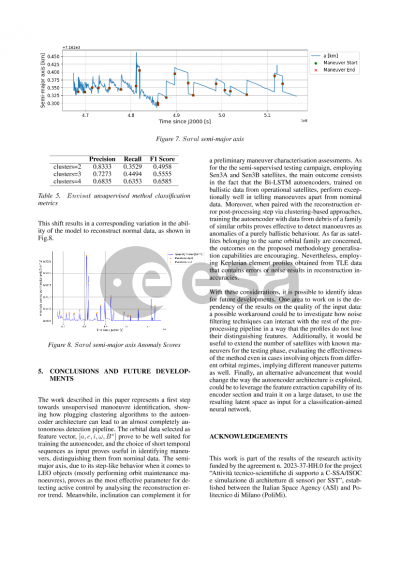
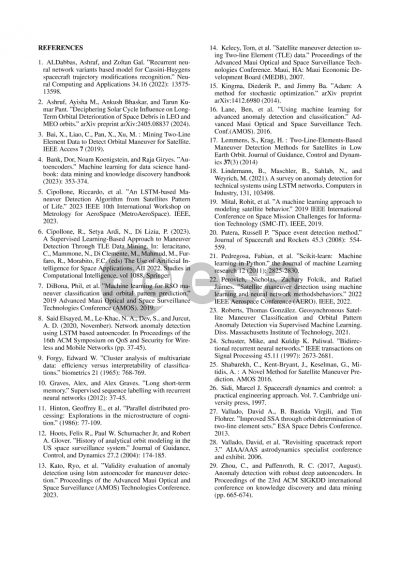