Document details
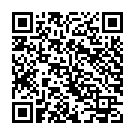
Abstract
The growing density of resident space objects in orbit is resulting in an increase in conjunction events. Consequently, collision avoidance has become an essential component of satellite operations, with many organizations relying on on-call satellite operators to initiate avoidance maneuvers to mitigate collision risks. Given the anticipated rise in conjunction events in the coming decade, it is essential to automate this process to reduce operator workload and enable scalability. This study aims to identify and compare the decision-making processes used by satellite operators across five major organizations – German Aerospace Center (DLR), European Space Agency (ESA), GMV, Korea Aerospace Research Institute (KARI), and HawkEye 360 – to inform the development of automated decision-making systems. Outcomes from the comparative study are used to devise deterministic models and refine a previously developed Long Short-Term Memory (LSTM) model, which are subsequently assessed on their ability to make reliable go/no-go avoidance decisions.
A set of Conjunction Data Messages (CDMs) pertaining to 30 high-risk conjunction events was provided to a decision-making analyst from each organization. The analysts were tasked with assigning a go/no-go maneuver decision to each event based on their perceived criticality of the conjunction. Additionally, an accompanying survey was provided to the analysts to gain deeper insights into the decision-making process. For each event, the analysts stated their rationale behind the decision made, specifically highlighting the feature(s) that contributed most to their decisions. Feature importance from the survey is processed to obtain normalized weights for each feature. Feature values are normalized to capture their relative criticalities, using pre-defined thresholds. Additionally, qualitative mentions of other influential factors, such as the presence of unreliable trends in certain features, are used to develop new features to quantify such characteristics. The feature criticalities and weights obtained from each analyst across the 30 events are used to formulate weighed sum models, which output a deterministic go/no-go decision for each event. These are supplemented with conditional statements, to include select 'rules' analysts are found to abide by: for instance, assigning a no-go to a high-risk event, if the time to closest approach is sufficiently large. 5 distinct deterministic models, one for each analyst, are formulated in total and assessed on their ability to match the decisions made by the analysts.
The LSTM model, which was trained using supervised learning with 200 DLR analyst decisions, is adapted to leverage the findings from this work. It is retrained using features highly weighted by analysts in this study, along with new features used in the deterministic models. The performances of the initial and modified LSTM models, and the deterministic models, are compared to evaluate each model's alignment with the analysts’ decisions. Feature importance analysis is applied to the LSTM models to aid transparency by identifying which features contributed most to the models’ predictions.
This study discusses the factors affecting go/no-go decisions made by analysts at different organizations, the development of numerical and AI models to reproduce such decisions, and the practicability of using an automated process to characterize collision risks.
Preview
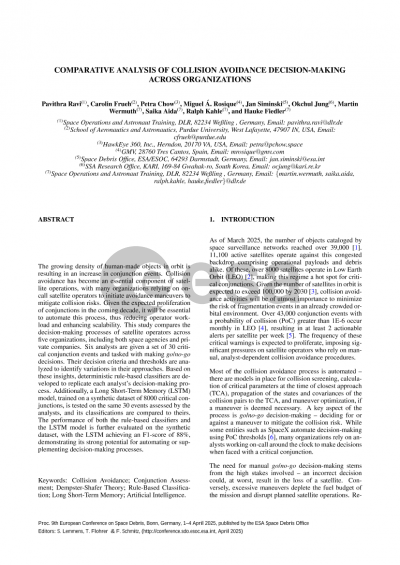
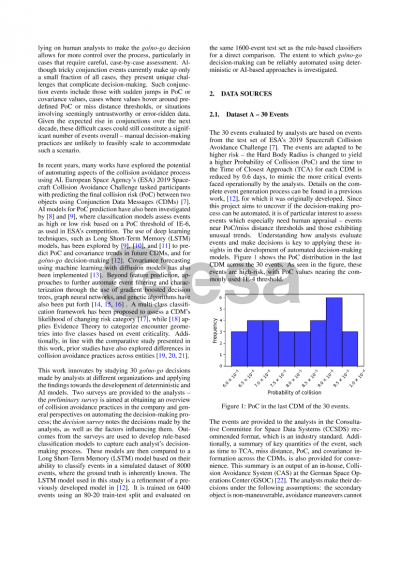
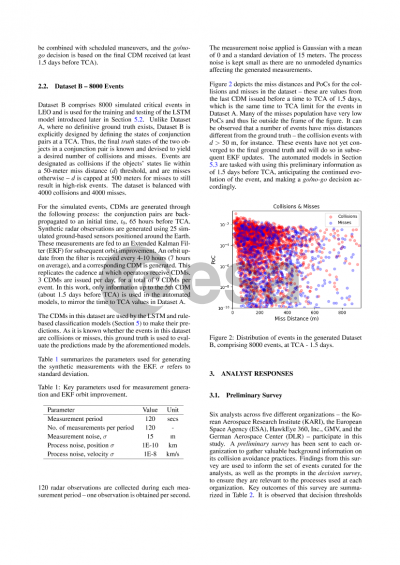
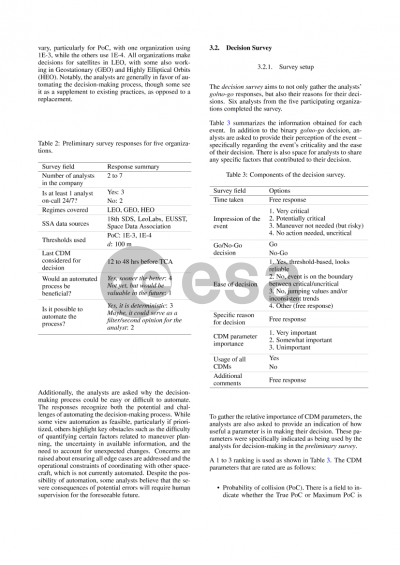
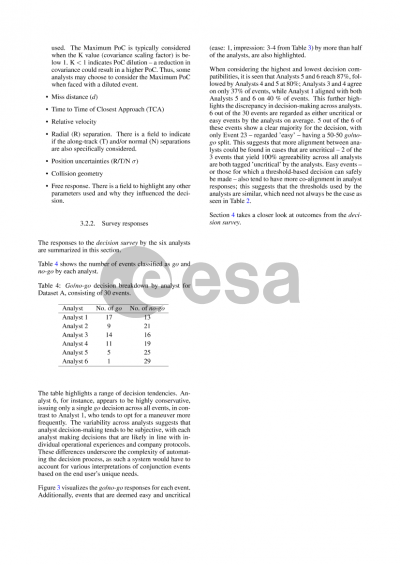
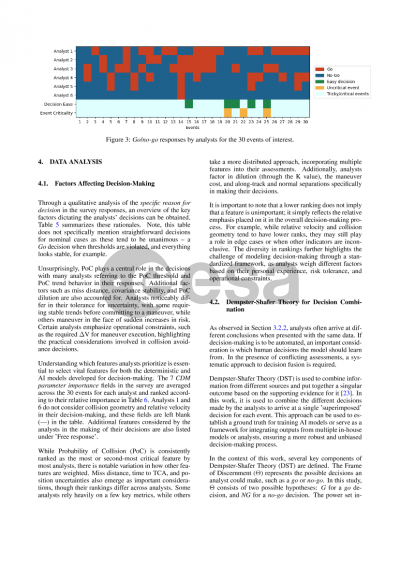
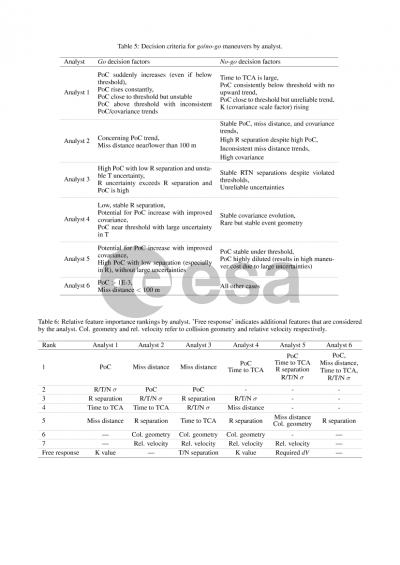
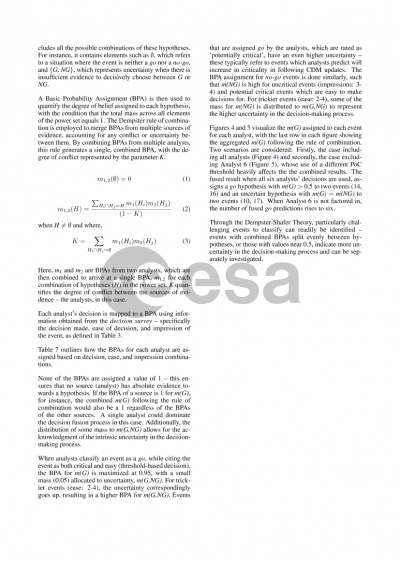
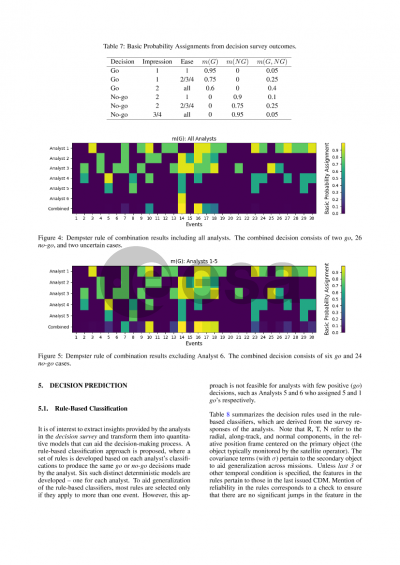
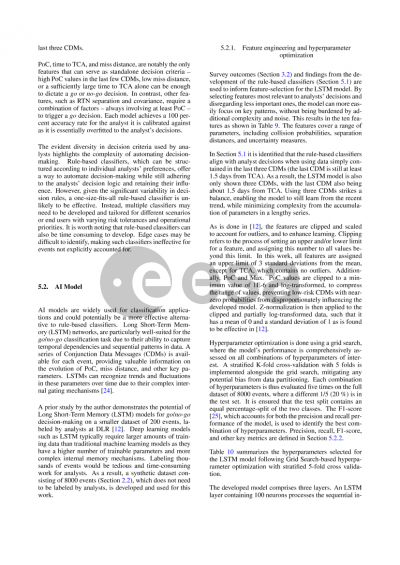
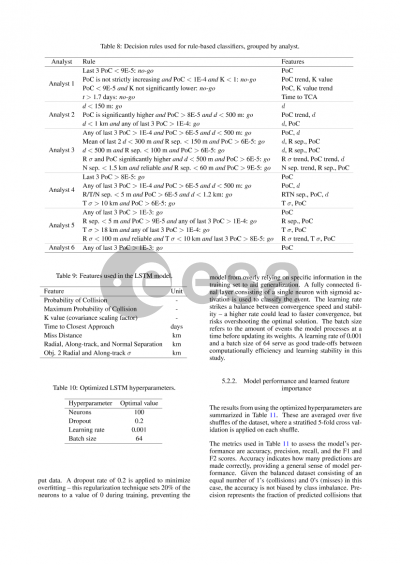
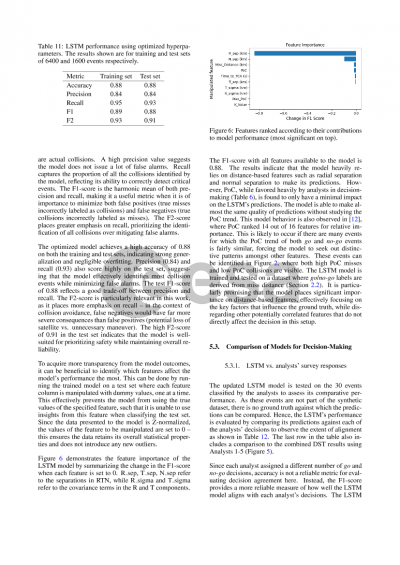
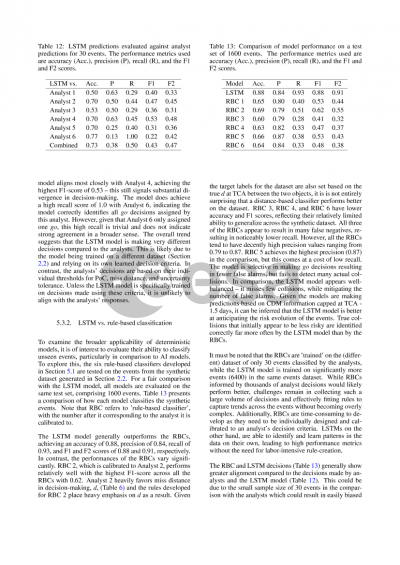
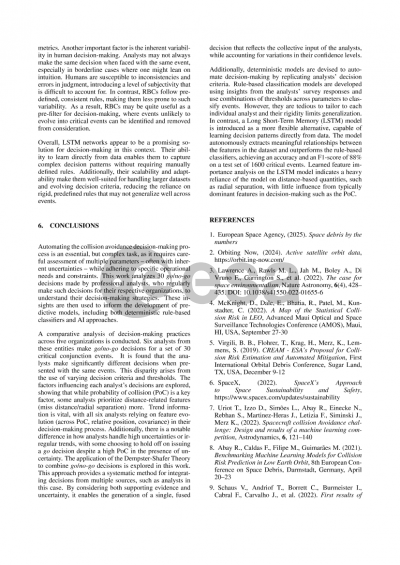
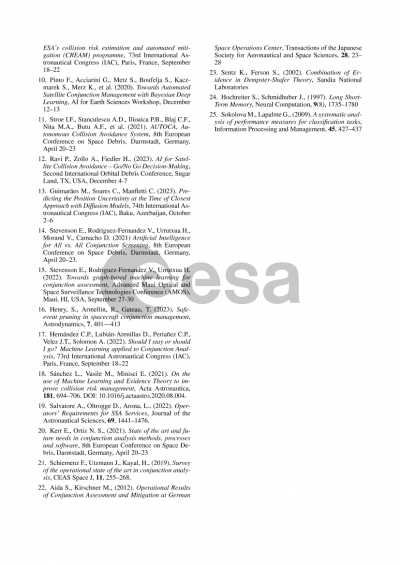