Document details
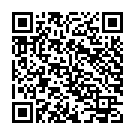
Abstract
In recent years a combination of technology maturation and space accessibility has allowed a proliferation of payloads. A result is an increase in the number of launches, which continues to exacerbate the problem of maintaining and increasing Space Situational Awareness (SSA) for safe space operation. A critical objective of SSA activities is the generation and maintenance of the Resident Space Objects (RSOs) catalog. An observation strategy and the characterization of tracklets from the collection of images are among a set of initial key elements that contribute to the continued accuracy of a catalog. In this paper, a sensor tasking optimization algorithm by Frueh et al. (2018) is used to determine observation schedules that maximize object coverage for tracking objects in the Geosynchronous (GEO) orbital region. The detections from collected images with Purdue Optical Ground Station (POGS) are processed with a multi-layer probability hypothesis Density (PHD) filter developed by Frueh et al. (2021) with improved data-driven birth.
To generate observation schedules for POGS, the sensor tasking algorithm considers several visibility conditions and prioritizes unobserved objects to obtain the optimal viewing directions. The algorithm utilizes prior knowledge of cataloged objects, which is obtained from the United States Strategic Command's Two-Line Element (TLE) catalog to initialize viewing directions. A set of observation schedules is generated, which details information on observation epochs, viewing directions, tracking rates, and potential cataloged objects to be observed. To increase the reliability and effectiveness of the observations, several visibility conditions are included. The conditions are categorized into, celestial, terrestrial, and sensor-specific constraints. The images taken accordingly by the sensor are processed and analyzed, by comparing observation results to the schedule sent to the sensor. The POGS sensor pointing and timing accuracy are evaluated against the expected values derived from calibrations. Additionally, a comparison is drawn between constant and variable sensor repositioning time, by characterizing the number of observations over time and computational efficiency.
The multi-layer PHD filter is composed of two steps, where the first step forms tracklets from a collection of image sets with a PHD filter in the orthogonal image plane. The formed tracklets from image sets are validated independently with a Mahalanobis filter, which associates detections in each image separately. The second step of the multi-layer PHD filter utilizes a data-driven approach for the full orbital PHD filter. For both steps of the multi-layer PHD, a Gaussian mixture implementation is used. The data-driven approach in the second step inherently contains both persistent and birth PHD in the measurement update step, where birth is seeded by a measurement correlation optimization process. The optimization is done on a boundary value problem, where current and previous measurements are correlated. Successful correlations are characterized by a correlation likelihood function, which forms the birth instantiation hypotheses. A test of the improved multi-layer PHD filter is conducted over a set of collections for a night, where the results are compared to the corresponding TLE objects in the GEO orbital region.
Preview
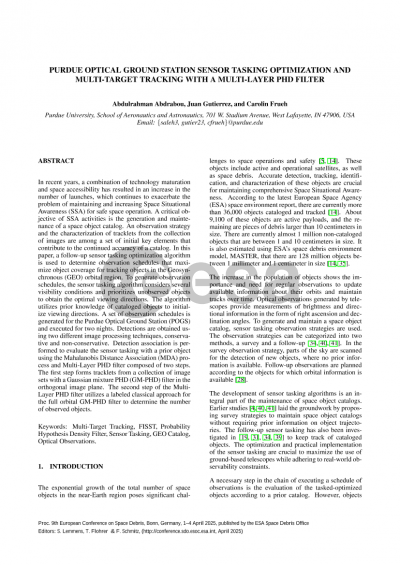
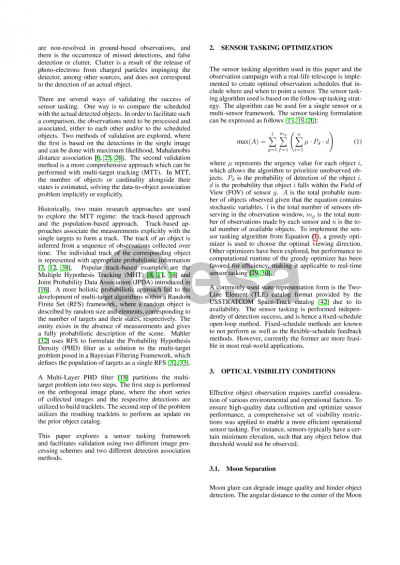
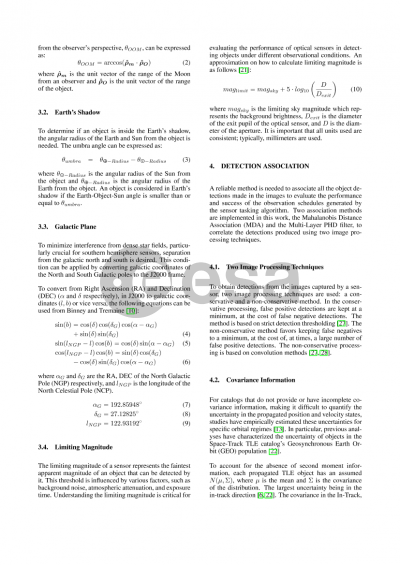
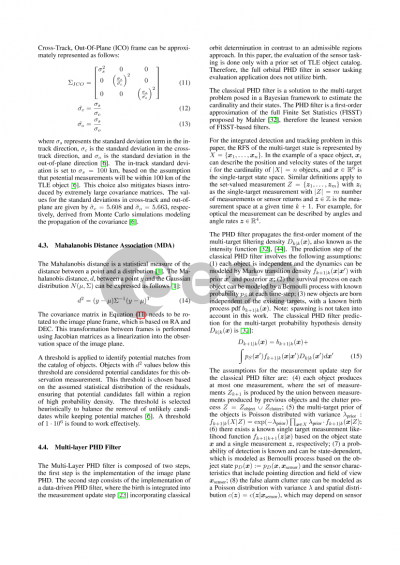
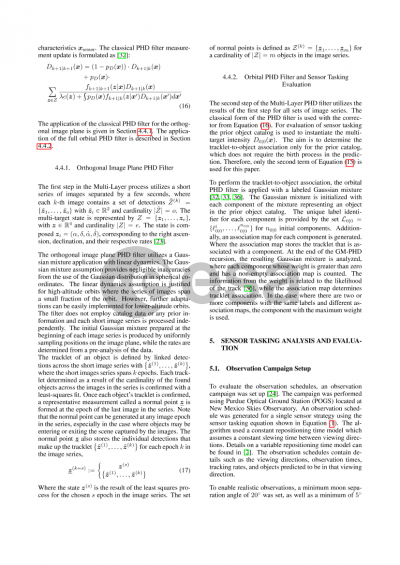
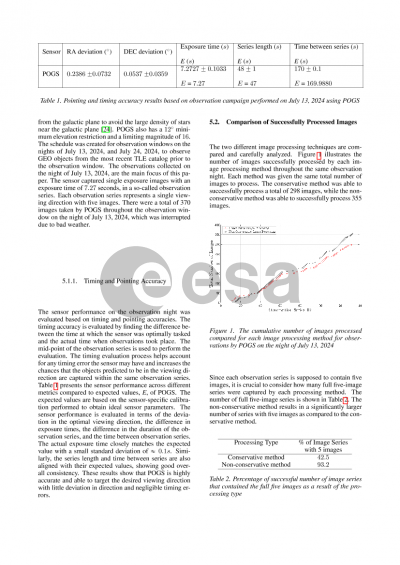
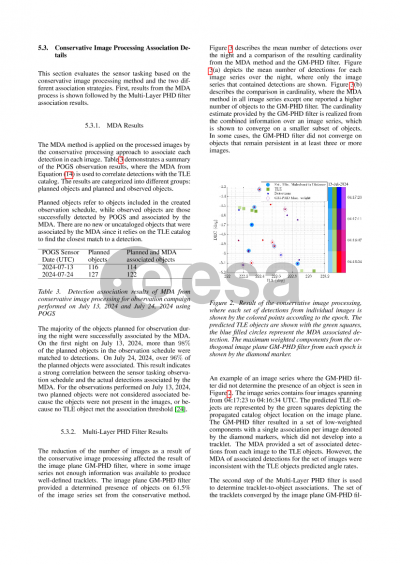
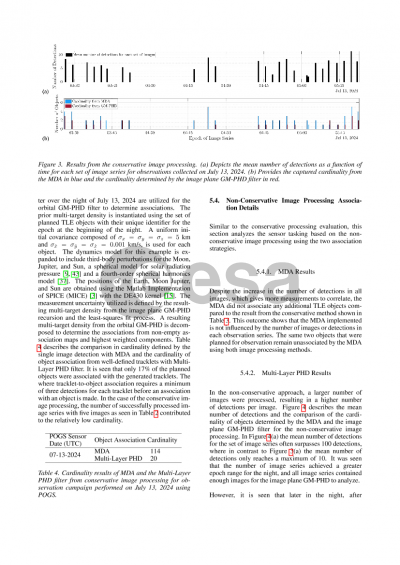
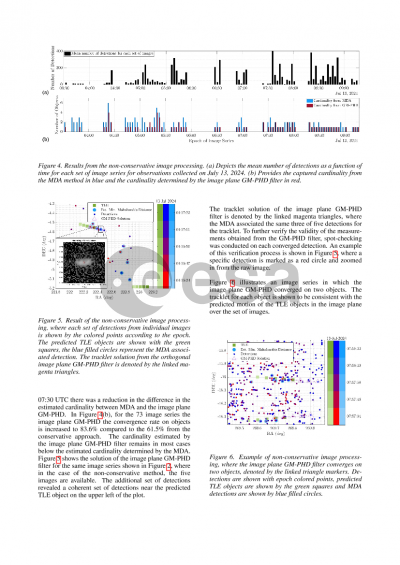
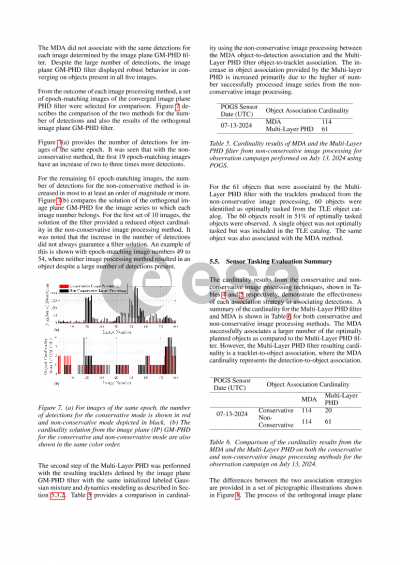
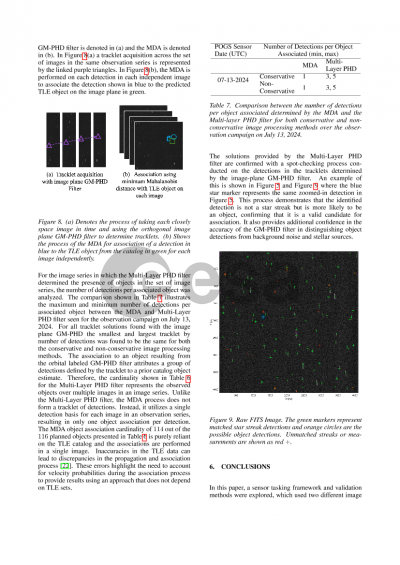
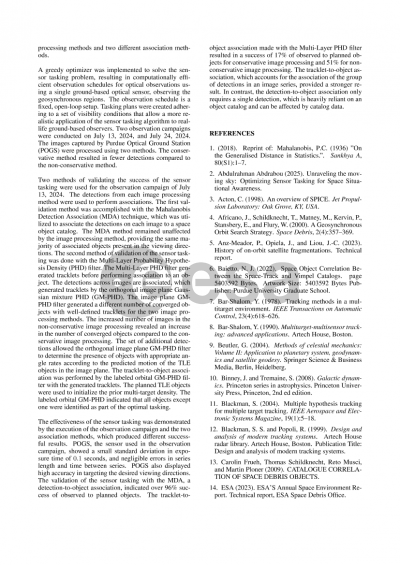
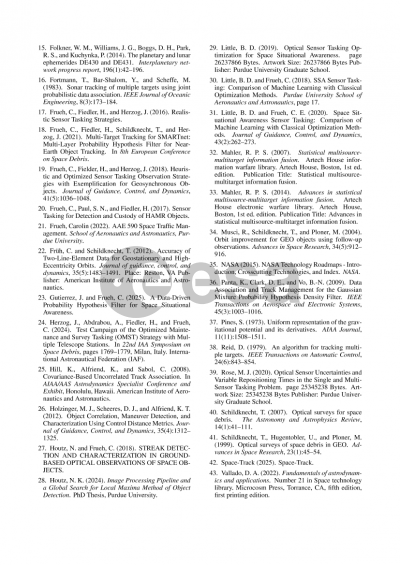
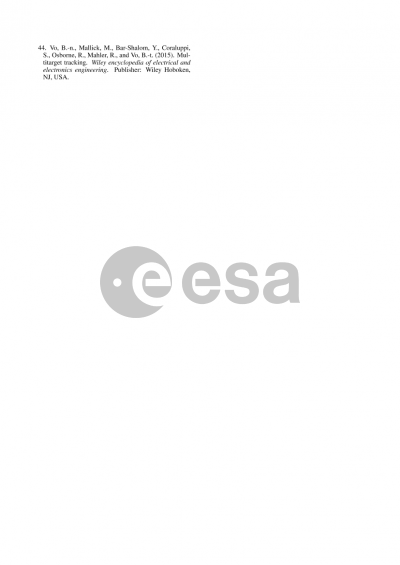