Document details
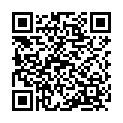
Abstract
The dynamics of space objects in Low Earth Orbit (LEO) is strongly determined by the effects of atmospheric drag: this complex interaction, dependent on the physical properties of the atmosphere, comprises several of the primary sources of orbit uncertainty in LEO.
Moreover, accurate calculation of the collision probability or re-entry time of a given object requires both an accurate prediction of the orbit, as well as an accurate estimation of the associated uncertainty.
However, most atmospheric density models, both empirical and semi-empirical, do not explicitly provide an estimate of the uncertainty.
In this work, we propose a Deep Learning technique based on the use of autoencoders (Champion et al., 2019), a family of artificial Neural Networks, to construct a grey-box model of the dynamics of the thermosphere density field. This gray-box model allows for a nearly real-time quantification of the uncertainty in the dynamics using satellite tracking data (Gondelach and Linares 2020).
Building on the DTM-2013 density model (Bruinsma 2015), we develop a reduced-order dynamical model of the thermosphere density: autoencoders can be used to construct a nonlinear embedding of the high-dimensional, chaotic dynamics associated with the physical space, mapping it onto a low-dimensional manifold. This Deep Learning architecture provides a promising generalization to Proper Orthogonal Decomposition, in which the relation between the reduced-order state and the observed one is not necessarily linear.
The Neural Network can be structured following the encode-propagate-decode model so that the dynamics associated with the reduced-order model can be reconstructed in tandem with the model reduction and decomposition, as opposed to the sequential decomposition performed in previous works (e.g., Mehta and Linares 2018).
The dynamics is reconstructed via Deep Symbolic Regression (Manzi and Vasile 2020) and can include space weather effects (related to the F10.7 index) and geomagnetic activities (related to the a_p index).
As opposed to related works in the field, no linearity assumption is made here and the Deep Symbolic Regression yields a sparse, nonlinear term to be included in the differential equations governing the motion of the observed objects.
Making use of such a grey-box model, the estimation of the density field can be coupled with the dynamics of tracked objects, leading to real-time calibration to deal with both measurement and model uncertainties. The obtained model, because of its explicit differential formulation, enables one to take into account parametric uncertainties, leading to a more informed uncertainty quantification for a system of interest. This additional source of uncertainty can then be used to refine the computation of the probability of collision.
In the paper, we start by applying the proposed methodology to a simple, low-dimensional dynamical system whose underlying model is explicitly known. After that, the technique will be applied to model the dynamics of the thermosphere density field. The forecasting capabilities of the constructed model will be assessed, comparing its performance with traditional density models.
Preview
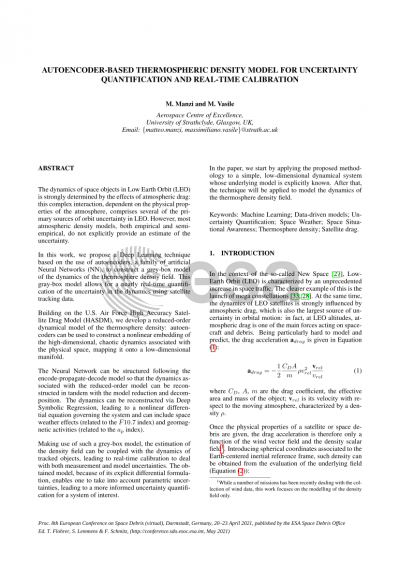
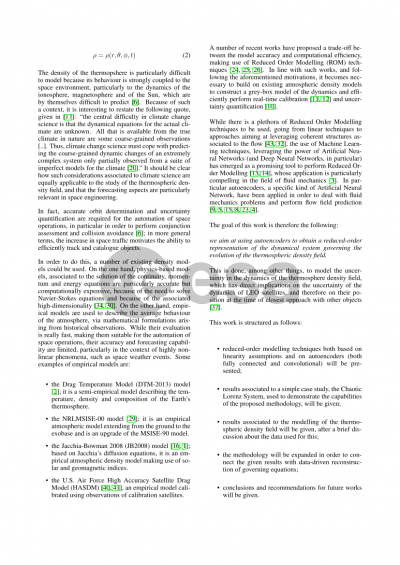
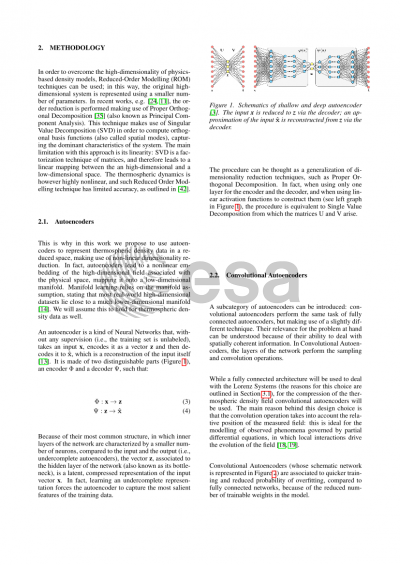
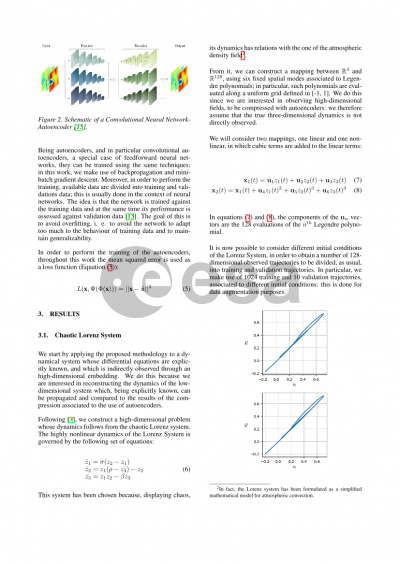
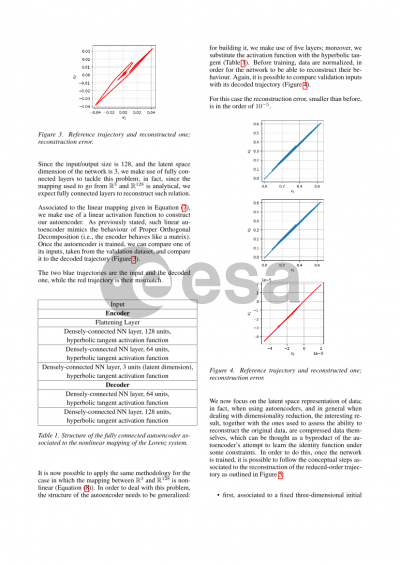
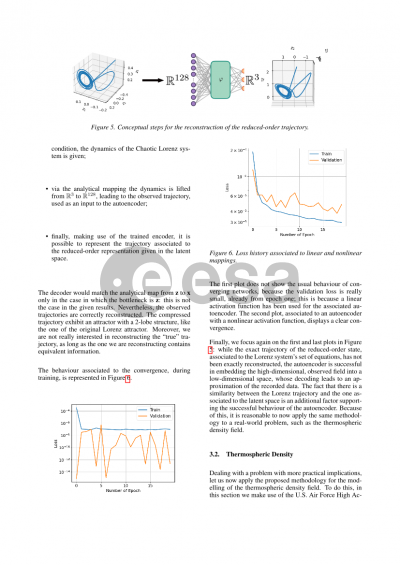
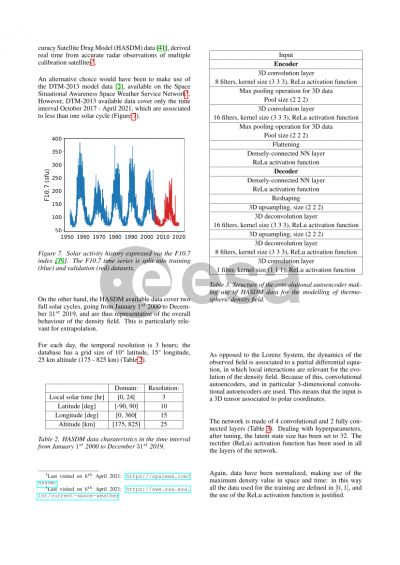
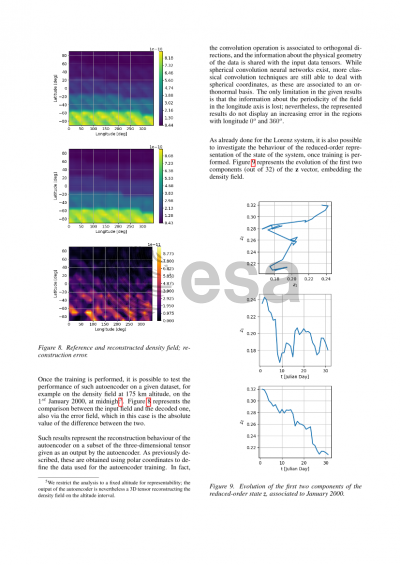
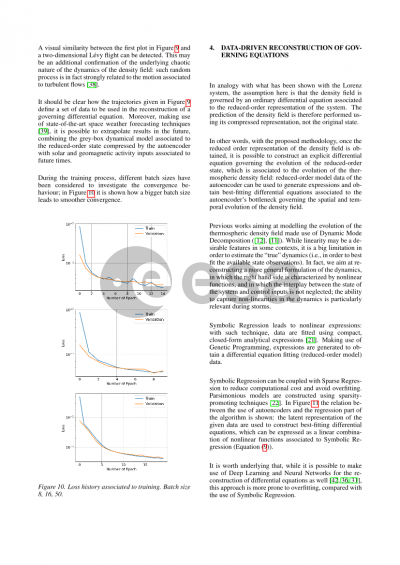
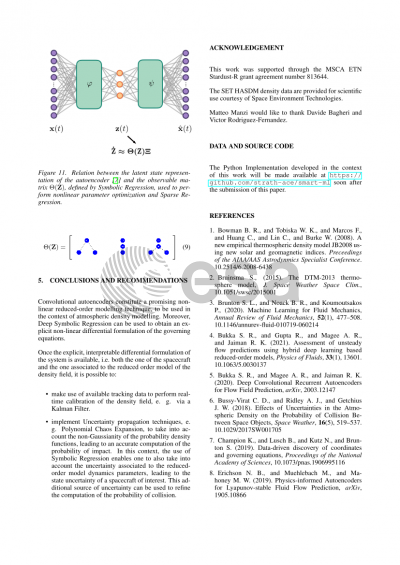
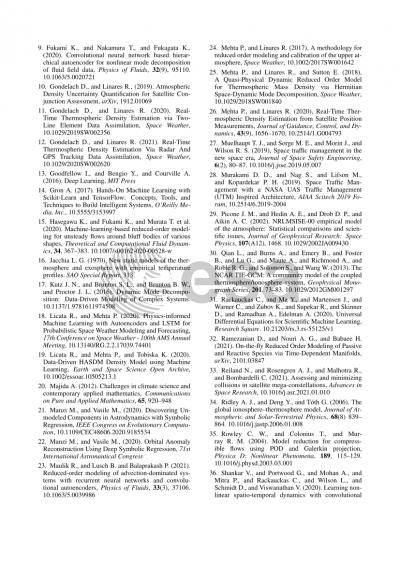
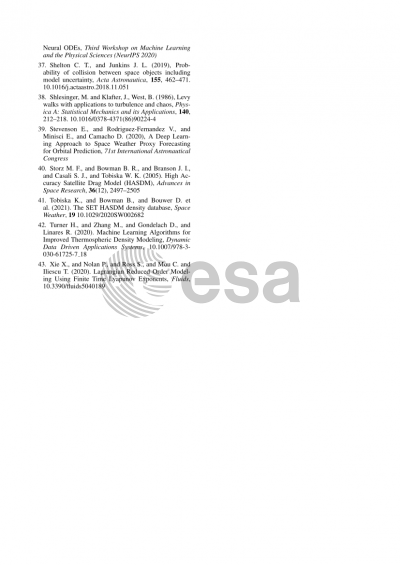