Document details
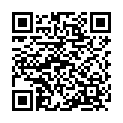
Abstract
The classification and characterisation of objects in geosynchronous orbit (GEO) is an important goal in Space Situational Awareness (SSA). Optical observations can be used to detect and identify GEO objects, while radar is generally only used to observe Low Earth Orbit due to the range limitations. Temporal variations of apparent magnitude, called a light curve, are captured by optical telescopes. This optical signature is usually processed with various estimation algorithms to get insightful information on the state of the object. Light curves contain information on features such as attitude, size, shape and materials useful for the characterisation of the object. Analysing light curves can allow the determination of normal patterns of life, and hence the detection of anomalous behaviour during the orbit such as manoeuvres.
The main objective of this study is to develop a high-fidelity simulator for generating photometric data and light curves to be further processed and used to train the machine learning algorithm for extrapolating common features of GEO objects. The simulated observations are intended to reproduce images taken by Deimos Sky Survey (DeSS) system, located in Puertollano (Spain). This paper details the development of the simulator, and the development and initial results of the machine learning algorithm.
Ephemeris from the selected object are collected in order to allow the simulator to perform a visibility check with respect to the observer location to ensure good quality observations. Satellite, Sun and Moon elevation, and Earth shadow are the main geometrical constraints for good image quality. A geometric model of the selected object is given to the rendering software PovRay, together with its attitude and material properties, in order to generate photo-realistic images from the perspective of the ground-based observer through the use of the ray tracing technique. After a series of images has been rendered, they are processed and calibrated to determine the apparent magnitude and extract light curves. In order to assess the quality of the results and validate the simulator, simulated light curves were compared with the real ones generated from real observations taken by DeSS.
After the acquisition of simulated and real light curve data, an artificial neural network has been trained to identify characteristics of spacecrafts in GEO. Depending on the amount and the quality of input data available the algorithm can extract a range of features from the light curve. Initially, the algorithm looks at pattern of life, through analysis of rotation rates, then it considers geometry and materials. Assuming the geometry is known, the attitude of the spacecraft can then be derived. This is the first step in automatic characterisation of objects. The simulator can be used to generate a full night light curve for an orbiting object. By varying the simulator inputs, many different nights, under different conditions and for different objects can be simulated. A wide dataset will help the algorithm to recognise a broad range of satellite types, attitudes and many other characteristics. Full validation of the algorithm is ongoing, using real data gathered by DeSS.
Preview
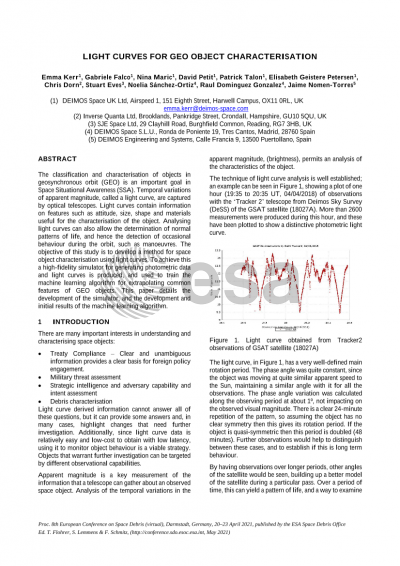
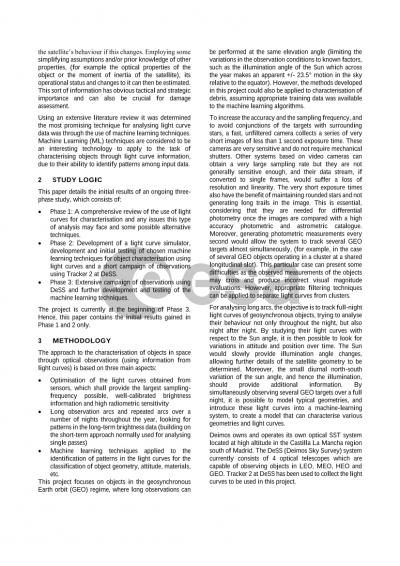
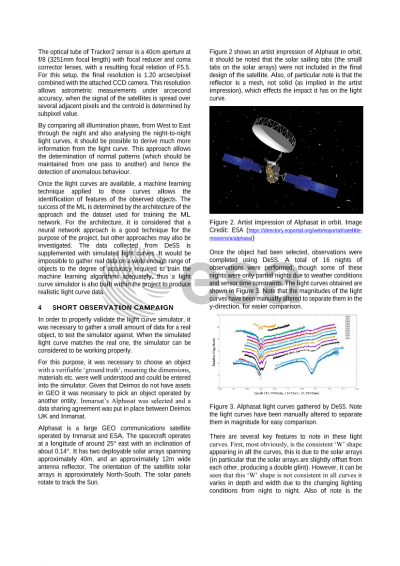
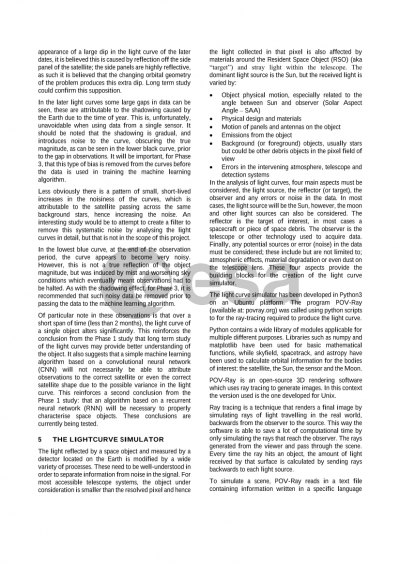
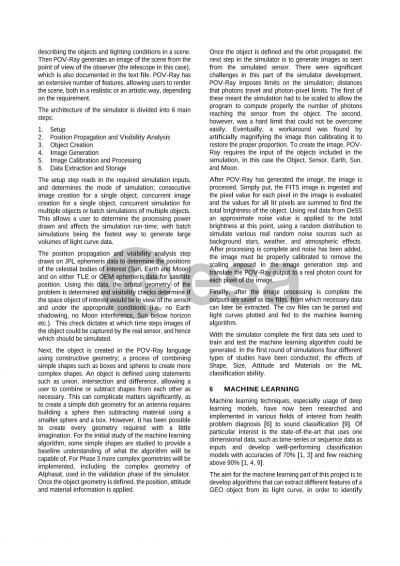
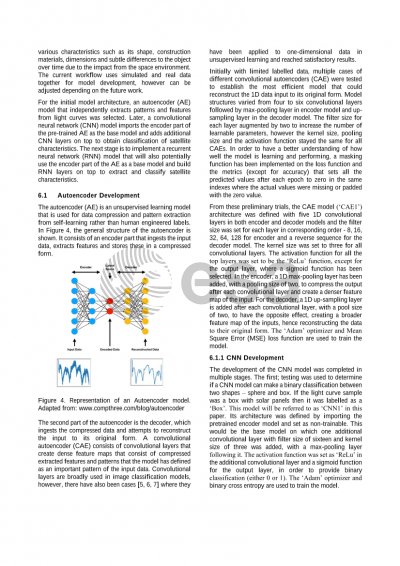
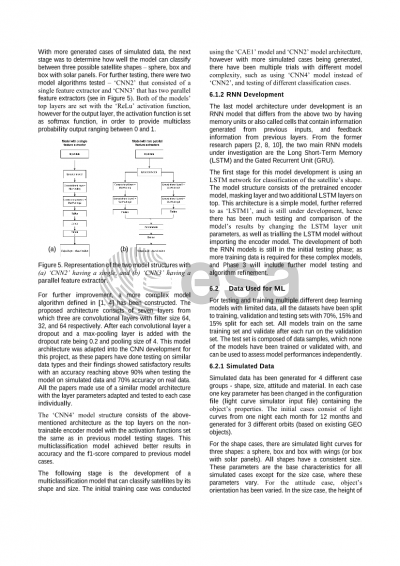
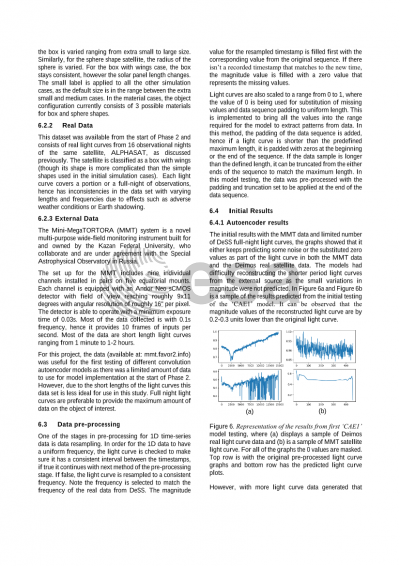
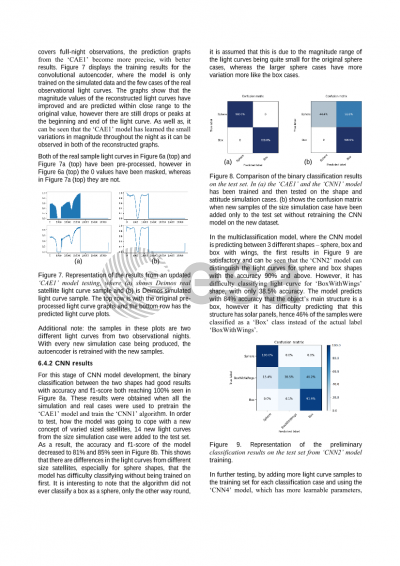
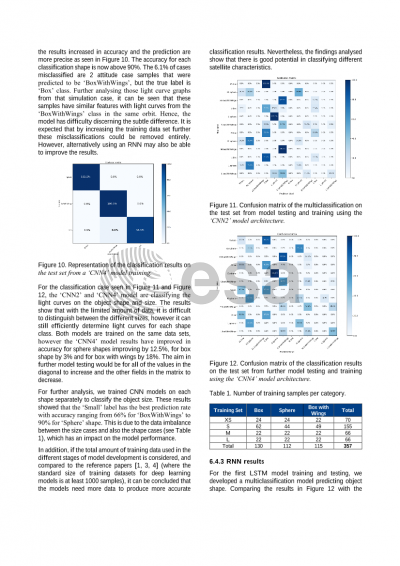
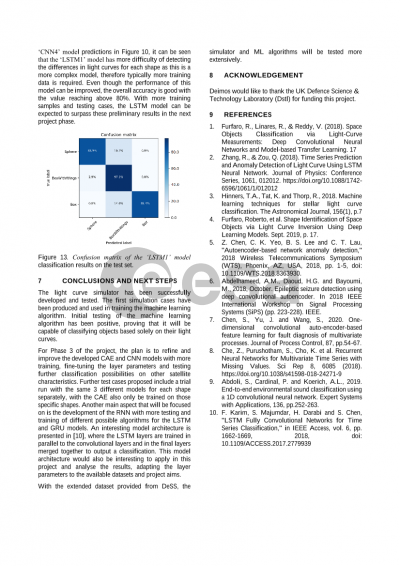