Document details
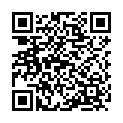
Abstract
Due to the increasing and frequent launch activities in the last decades, the number of artificial space objects has been growing steadily, and most of them are space debris. This threatens the safety operation of human space assets and the sustainable development of the space industry. A new concern sparked in Space Situational Awareness (SSA) is the reliability of collision assessment based on a single data source. False warning messages coming from a single input have not been uncommon in recent years. This may lead to an unnecessary maneuver operation that shortens the mission lifetime, disrupts scientific research, and even increases the risk of collision with other objects.
Global efforts are required to minimize the impact of unreliable collision assessment. As more surveillance sensors come on-line, an abundance of data becomes available to support SSA research. However, it is not surprising to receive conflicting collision warning messages from different data-providers, such that decision-makers are apt to get bogged down in confusion. Further, the statistical information of some data sources may be incomplete or totally scarce, e.g., two-line elements data, leading to a restriction in the capability to provide accurate uncertainty representations, which subsequently limits the probabilistic collision analysis that can be carried out and the results that can be derived.
These concerns raise the demand for a consistent utilization of various data sets and the capability to properly handle the imperfect uncertain information. This multi-source data fusion problem can be addressed using a credibility consensus strategy based on the Outer Probability Measure (OPM) theory. OPM is an extrinsic description of uncertainty, it provides a more faithful representation of epistemic uncertainty to account for the limited or imperfect information we possess of the system. Closed-form stochastic filtering solutions based on OPMs, dubbed credibility filter, have been derived for a recursive uninformative prior Bayesian estimation. The Sequential Monte Carlo (SMC) implementation of the credibility filter, namely credibility particle filter, has been successfully applied to space object tracking.
In this paper, a Credibility Consensus Particle Filter (CCPF) is developed. To our knowledge, the proposed method is the first distributed fusion algorithm that is fully derived based on the credibility framework. Compared to typical probabilistic consensus filters, the credibility consensus method can provide improved robustness since it accounts for the absence of statistical information of uncertain components in the system. Moreover, instead of taking the average of local estimates, the weighted consensus method adaptively tunes the fusion weight according to the credibility information gain of local posteriors. It is expected that the information-driven weighting strategy can achieve enhanced accuracy than that of typical average consensus algorithms.
The developed method is validated using both simulated and real space object tracking case studies. The simulated scenario considers a fusion task using a hybrid sensor network including optical and radar sensors. A more practical scenario further considers the fusion of real orbit determination results of a Flock satellite using four datasets, i.e., PlanetLabs, LeoLabs, JSpOC, and ASTRIA. Test results demonstrated the efficacy and accuracy of the CCPF method.
Preview
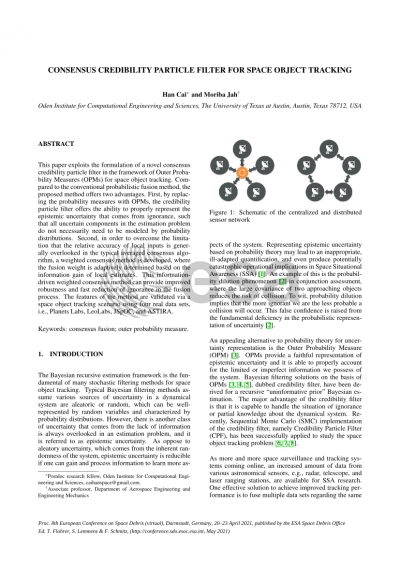
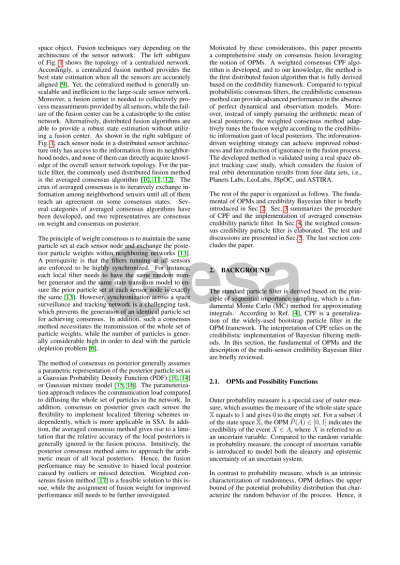
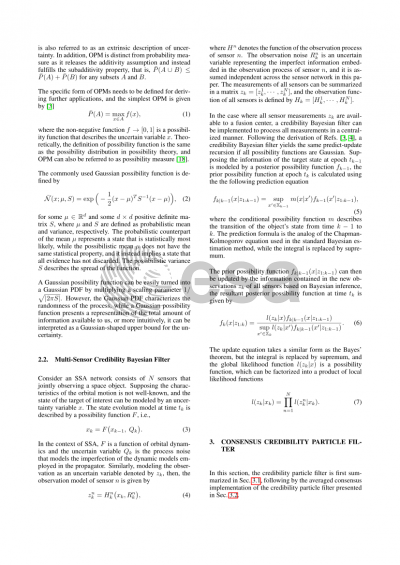
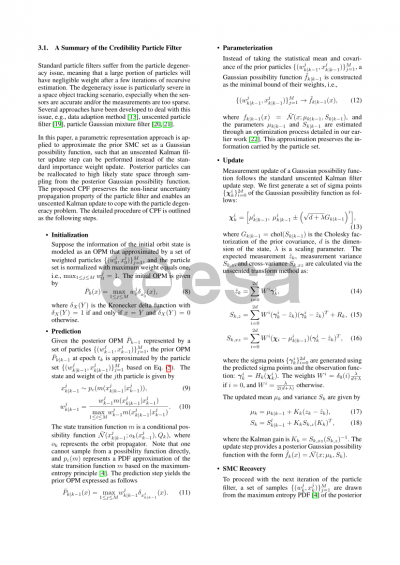
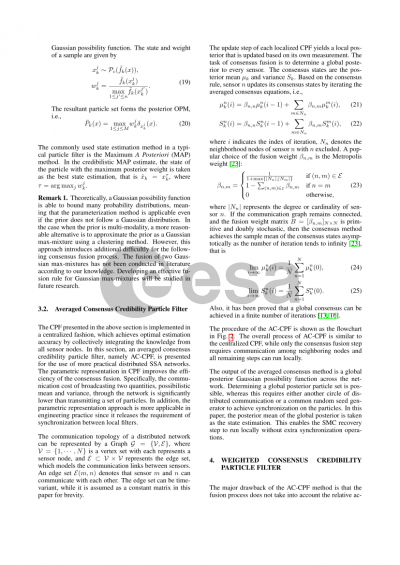
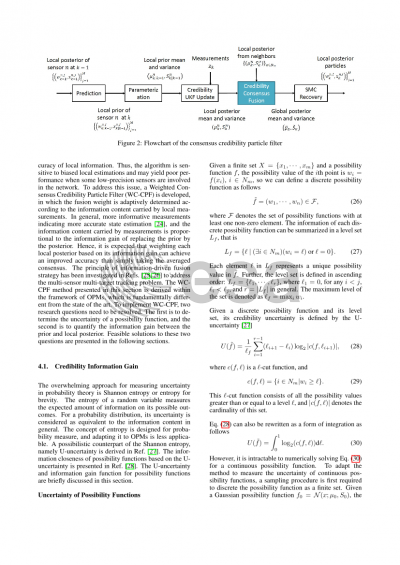
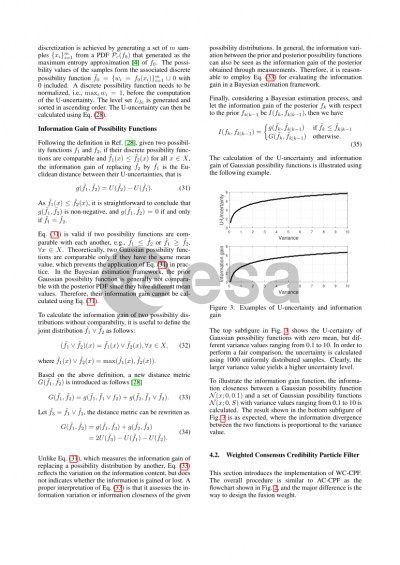
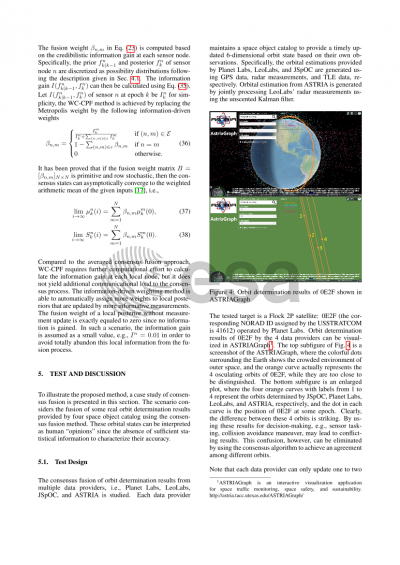
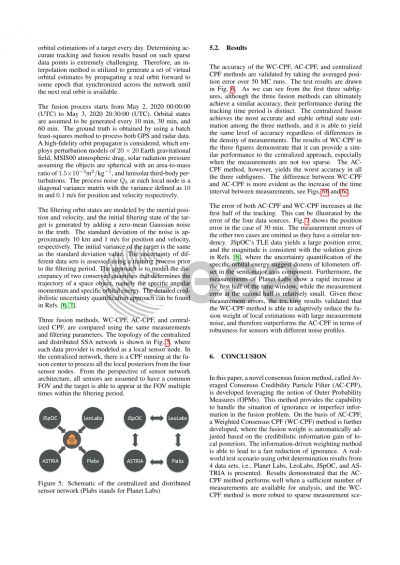
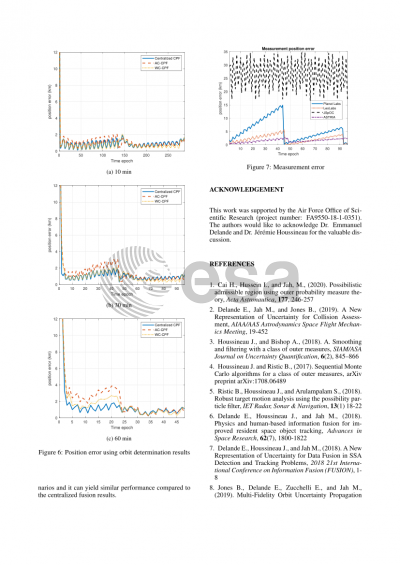
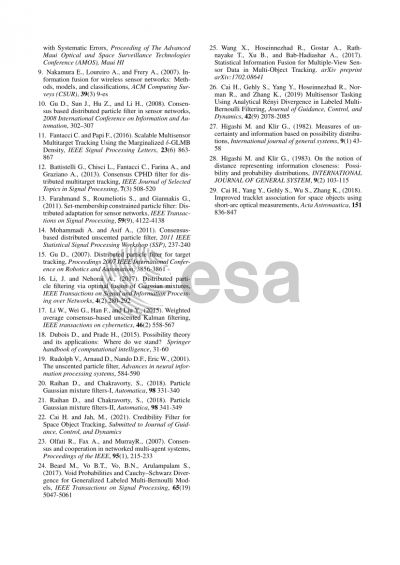