Document details
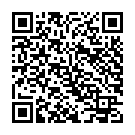
Abstract
In recent years, the number of active and defunct Resident Space Objects (RSOs) have been increasing. Additional artificial space objects orbiting Earth is becoming an issue for space situational awareness (SSA). Accurate identification of RSOs through ground-based photometric light curve measurements can aid in characterizing collision-prone objects to our orbital assets, planning for commercial use of space, and possibly understand the intent of foreign objects. Optical measurements for space object tracking are sensitive to shape, attitude, angular velocity, and surface parameters. Current state-of-the-art in RSO characterization relies heavily on nonlinear state estimation theory, multiple models, and full light curve inversion which is computationally expensive. A data-driven approach for improved accuracy with a large volume of objects employs the use of deep neural networks. Given an unresolved object’s light curve, in low earth orbit (LEO), we can characterize it by shape and spin rate using Recurrent neural networks and a hidden Markov model. This hybrid approach improves the accuracy of time series classification by executing a secondary screening accounting for the temporal relations in the data. Redundancy in the classifiers from multiple layers of training and prediction can be eliminated using confusion matrices. This integrated model has been developed to identify tumbling and stabilized objects by testing for aliasing, periodicity (using Fourier and phase-folding methods), and feature extraction. The performance of this approach for RSO classification is demonstrated via simulations. The model is designed to train and validate using synthetic light curve measurements of objects spanning ellipsoid, rocket upper-stage, CubeSat, torus, probe, and box-wing satellite, among others. Variations from different viewing geometries, altering shape/size dimensions and surface properties have populated the training dataset. The simulated measurements are evaluated using function integration (Lebedev quadrature) at varying angular rotational speeds and interpolated surface reflectance properties across the facets. Real light curves have also been integrated to adjust for noisy data. The fidelity of these simulations ensures it is both realistic and accurate to within 10% error, and the computation speed is faster with flexibility to deal with a larger amount of data.
Preview
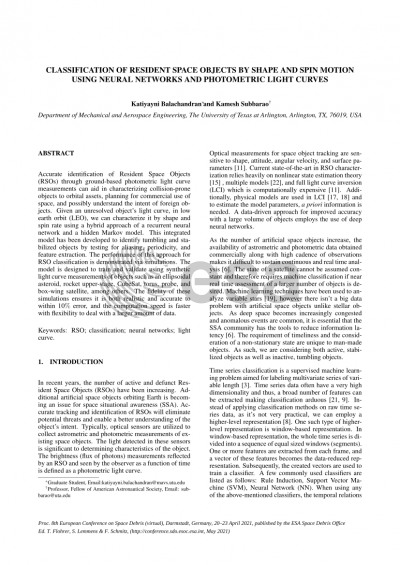
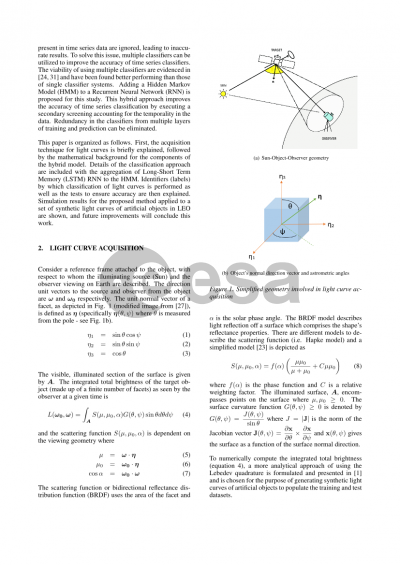
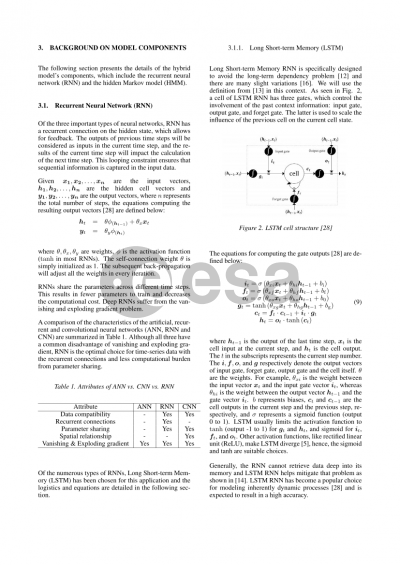
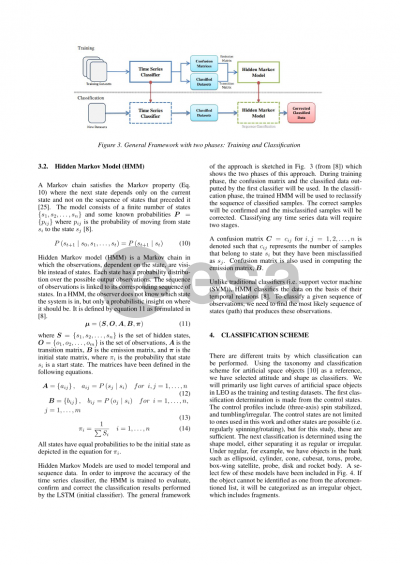
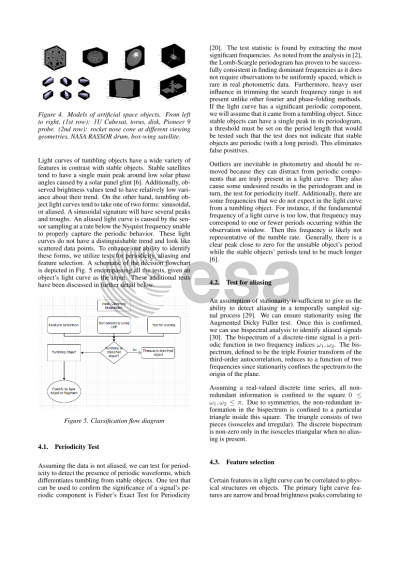
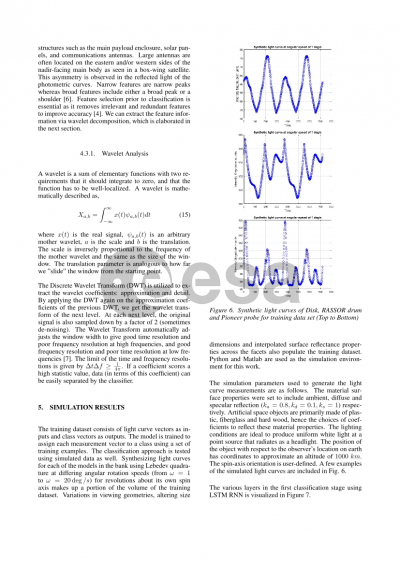
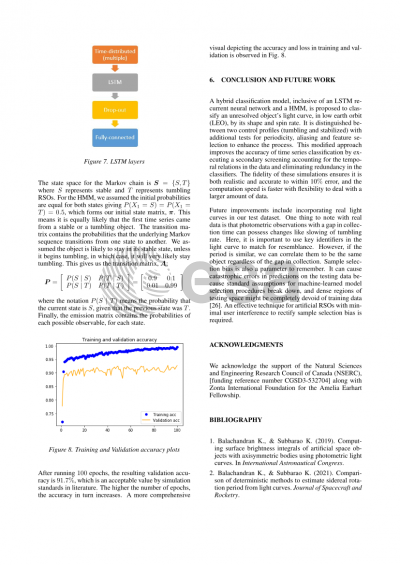
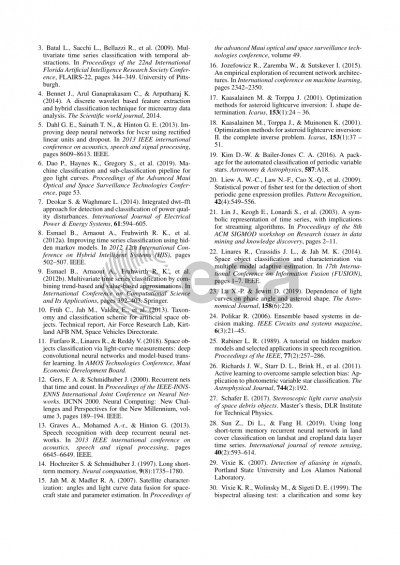
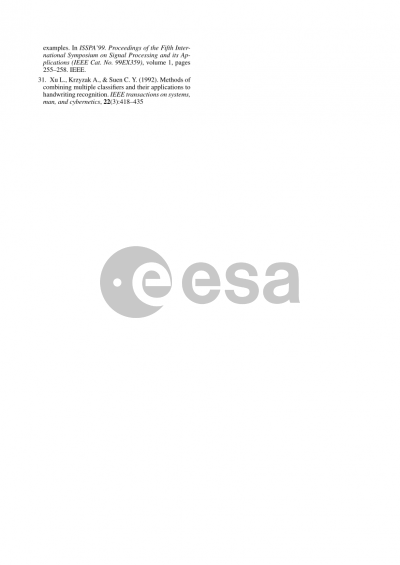