Document details
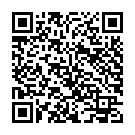
Abstract
Space Debris Surveillance and Tracking has become a necessity to ensure the safety of current and future space missions. Ground based infrastructure has seen large development in the past years and agencies are now considering space based capabilities to support identification and classification of Space Debris. LIFT ME OFF (LMO) has been contracted by the UK Space Agency to develop techniques for automatic detection and Identification in space of Space Resident Objects (SRO) using Machine Learning models in the frame of the UKSA grant call for “Advancing Research into Space Surveillance and Tracking (SST)”. The work was concerned with the detection, classification and monitoring of satellites and space debris.
The purpose of SST is to provide warnings to satellite operators concerned that a malfunctioning satellite or space debris could impact upon the safety of their own space assets. This paper will focus on how algorithms can be used for in-orbit detection and classification of satellites and space debris. The proposed solutions research the use of combining thermal infrared and visual sensors with Artificial Intelligence (Machine Learning and Deep Learning models) to create a space based SST solution.
The models were developed with emphasis on experimental data, for which a test facility was built in order to generate representative images for training the algorithms. The project also included a prototype test bed with sensors, electronics and algorithms to build some foundation techniques which can be later scaled up to an end-to-end autonomous algorithm for detection of anomalous behaviour that can raise warnings based on live information.
This paper presents a description of the experimental setup used for generation of training and validation images, the test cases selected for this development, the algorithm used and the performance achieved, as well as the lessons learnt to be implemented for future work.
Preview
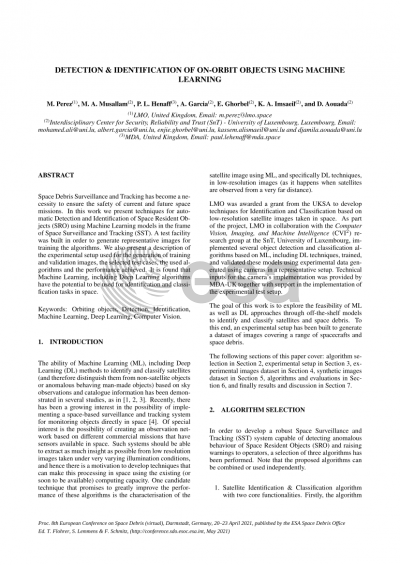
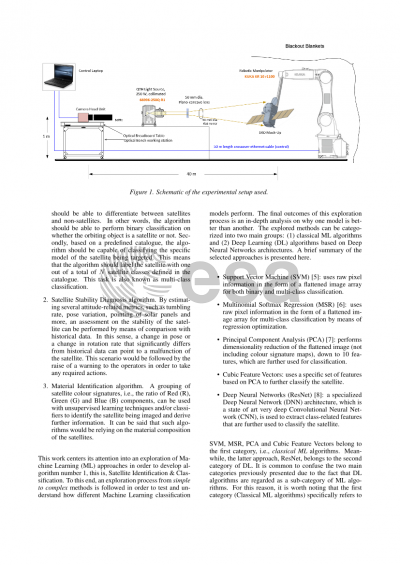
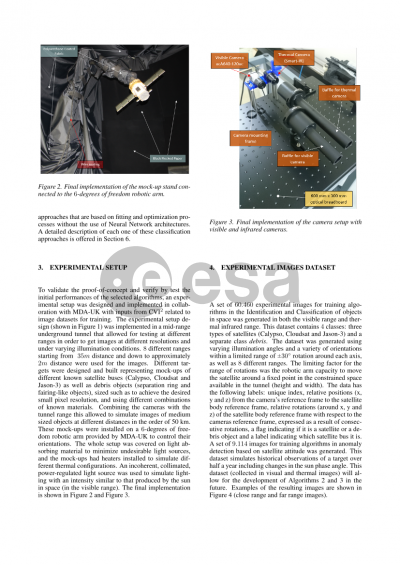
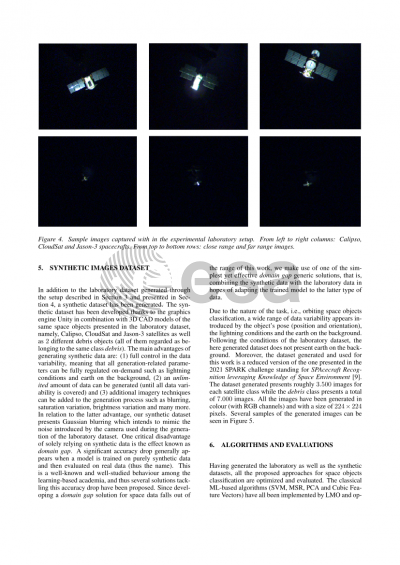
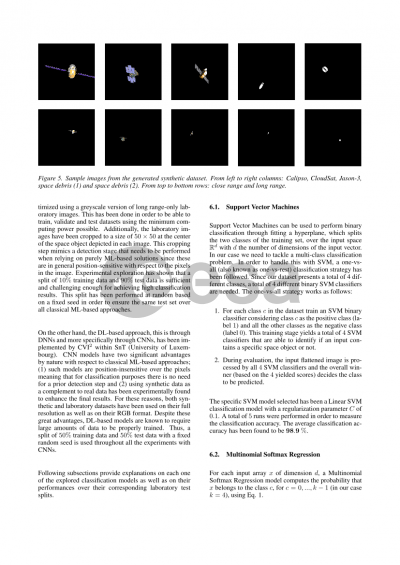
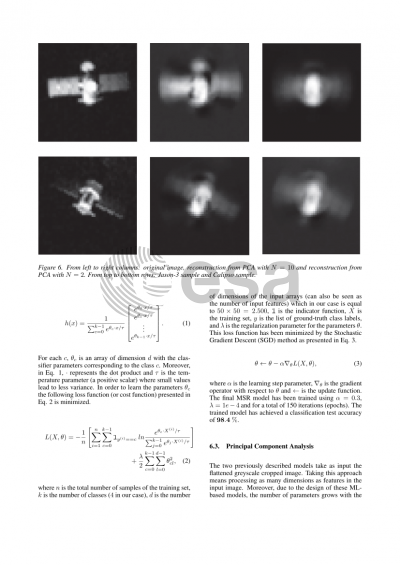
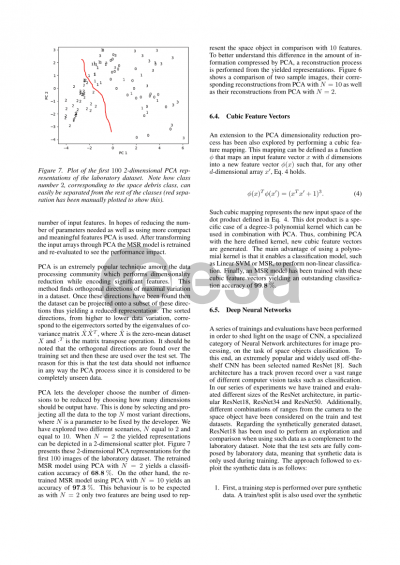
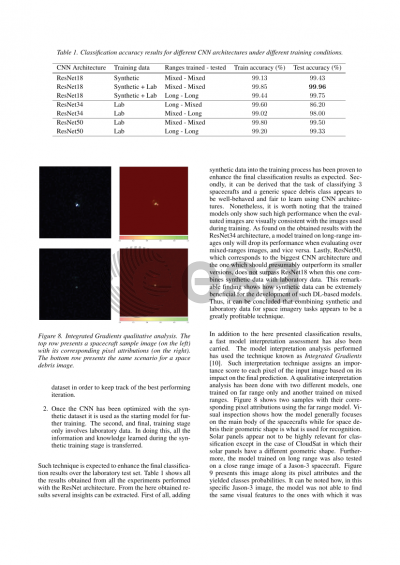
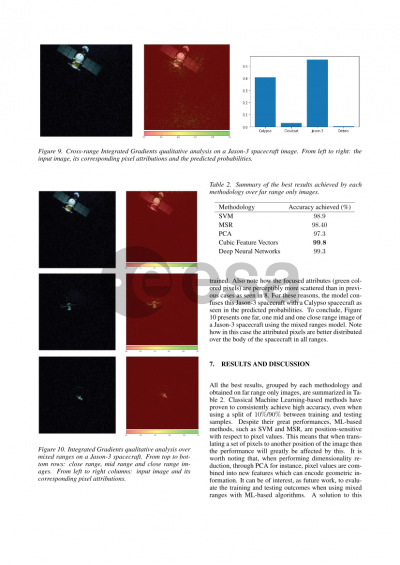
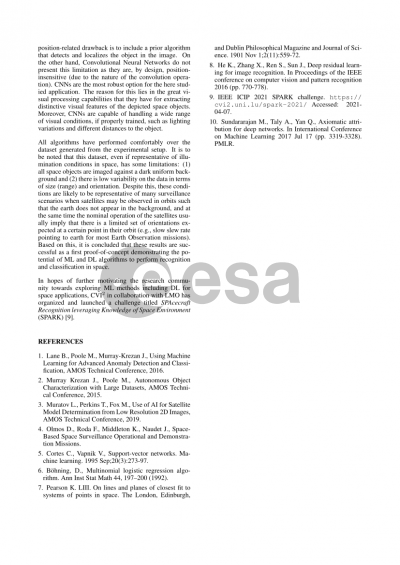