Document details
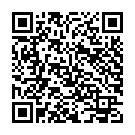
Abstract
Space debris are routinely tracked and followed for ultimately providing services of re-entry and conjunction analysis, among others. Estimation and pre-diction of the state of the space debris is at the core of such space surveillance and tracking operations. Performance of the estimation process can be mea-sured in terms of the correct characterisation of the state uncertainty, which is, in turn, necessary for the proper operation of the space tracking system. When tracking space objects, observations are not in abundance, and, therefore, propagation of the uncertainty from the epoch of the last observation update is key for the adequate performance of tracking algorithms.The work presented in this paper explores different methods of uncertainty propagation to meet the needs of state-of-the-art debris tracking systems. The work has been developed in the framework of the ESA contract of the same name. An assortment of approaches are detailed, defined and explored, to cover an extensive variety of methodologies. Among the uncertainty propagation (UP) methods, the focus is in the non-linear methods, i.e., those in which the non-linearity of the dynamics is explicitly taken into account. Non-linear UP methods can be classified in three broad groups: 1) probabilistic UP methods, that propagate the probability distribution of the state without regards to the specifics of the dynamic equation. This category actually includes most of the schemes: unscented transform (Julier and Uhlmann (2004)) and cubature methods in general (Arasaratnam and Haykin(2009)), or Gaussian mixture models (DeMars et al. (2013, 2014)); 2) Dynamics-based UP methods, include essentially three types of techniques, state transition tensors (STTs) (Fujimoto et al. (2012)), differential algebra (DA) propagators (Wittig et al. (2015)) and polynomial chaos expansions (Jones et al. (2013)); and 3) Hybrid UP methods, that aim at drawing from the advantages of different schemes (probabilistic or dynamics-based) by combining them, e.g., Gaussian mixture models combined with STTs or with a polynomial chaos expansion. In addition, stochastic and deterministic dynamical models, including a stochastic description of the atmospheric density, has been tested for assessing their influence on the overall performance of the UP methods. A series of study cases are selected to compare all the methods, taking into account both their computational cost and their accuracy (which is assessed by comparing with “brute force” Monte Carlo propagation). From the preliminary results obtained in the study, a selection of suitable UP methods is made. They are then used in two prominent applications: satellite collision risk assessment and re-entry analysis. For these two problems, the mapping of uncertainties into the quantities of interest, i.e., collision probability or re-entry time and location uncertainty, is also explored. Different techniques are proposed and specific metrics are defined for their performance assessment. Finally, a series of test cases are studied for both collision avoidance and re-entry analysis. From the work done, we expect to increase the awareness of the space debris community on the relevance of uncertainty propagation and help it in selecting the suitable approach for each of the applications, based on the results of the present study.
References
Arasaratnam, I. and Haykin, S. (2009). Cubature kalman filters.IEEE Trans-actions on automatic control, 54(6):1254–1269.
Crisan, D. and Ortiz-Latorre, S. (2013). A Kusuoka–Lyons–Victoir particle filter. Proceedings of the Royal Society A: Mathematical, Physical and Engineering Sciences, 469(2156):20130076.
DeMars, K. J., Bishop, R. H., and Jah, M. K. (2013). Entropy-based approach for uncertainty propagation of nonlinear dynamical systems.Journal of Guidance, Control, and Dynamics, 36(4):1047–1057.
DeMars, K. J., Cheng, Y., and Jah, M. K. (2014). Collision probability withGaussian mixture orbit uncertainty.Journal of Guidance, Control, and Dynamics, 37(3):979–985.
Fujimoto, K., Scheeres, D. J., and Alfriend, K. T. (2012). Analytical nonlinear propagation of uncertainty in the two-body problem. Journal of Guidance,Control, and Dynamics, 35(2):497–509.
Jones, B. A., Doostan, A., and Born, G. H. (2013). Nonlinear propagation of orbit uncertainty using non-intrusive polynomial chaos.Journal of Guidance,Control, and Dynamics, 36(2):430–444.
Julier, S. J. and Uhlmann, J. K. (2004). Unscented filtering and non linear estimation. Proceedings of the IEEE, 92(3):401–422.
Wittig, A., Di Lizia, P., Armellin, R., Makino, K., Bernelli-Zazzera, F., and Berz, M. (2015). Propagation of large uncertainty sets in orbital dynamics by automatic domain splitting.Celestial Mechanics and Dynamical Astronomy,122(3):239–261.
Preview
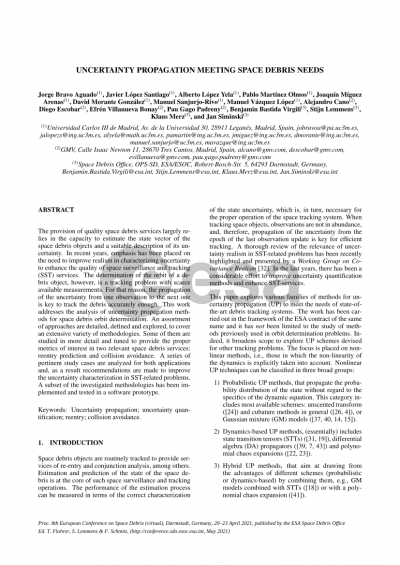
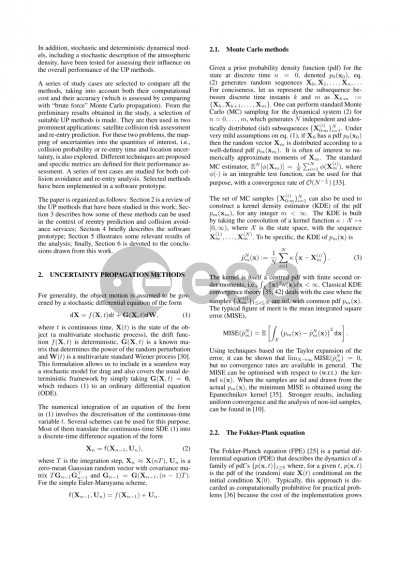
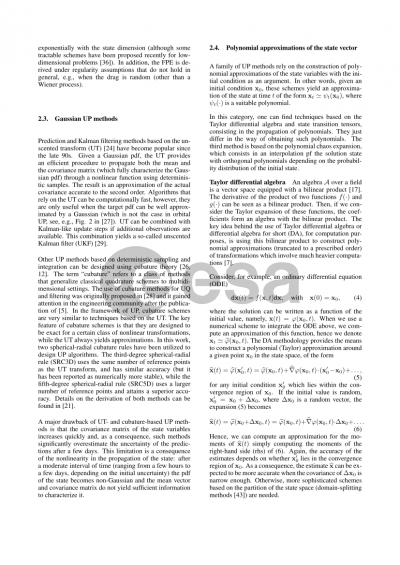
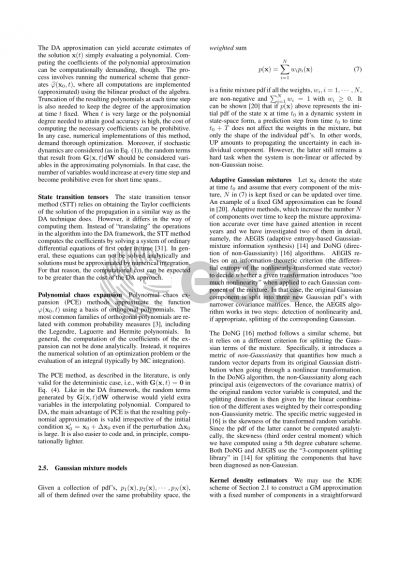
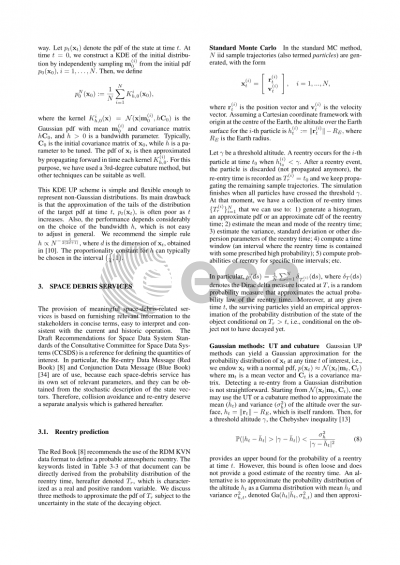
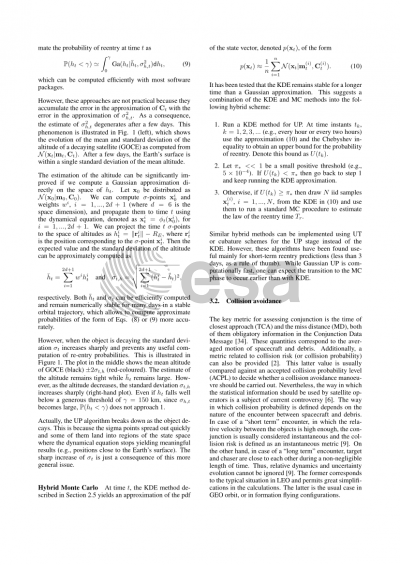
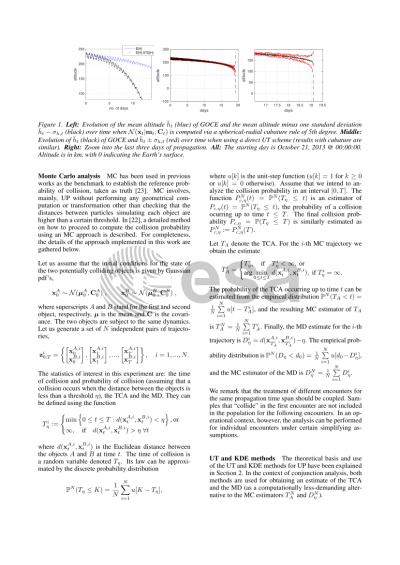
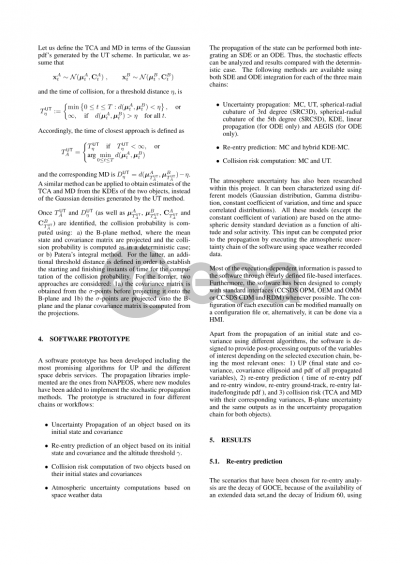
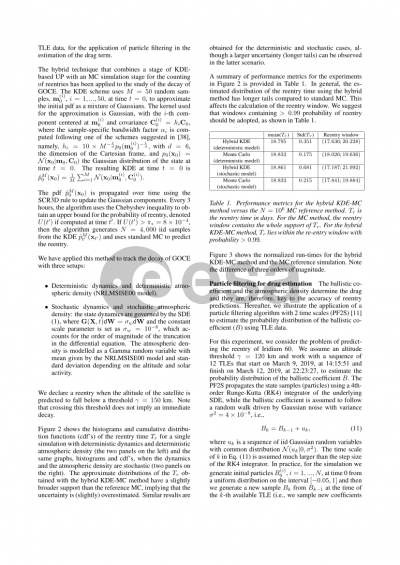
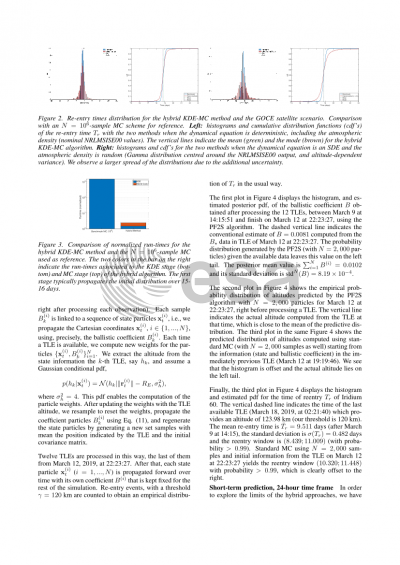
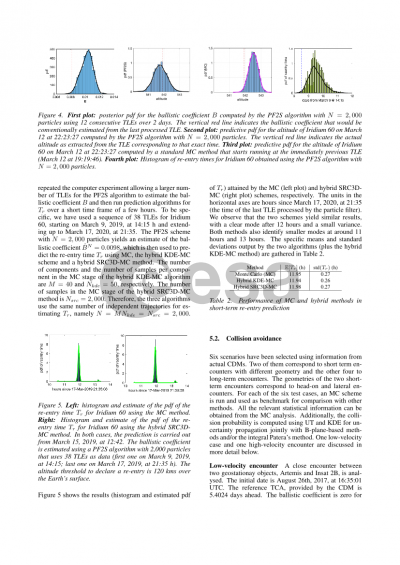
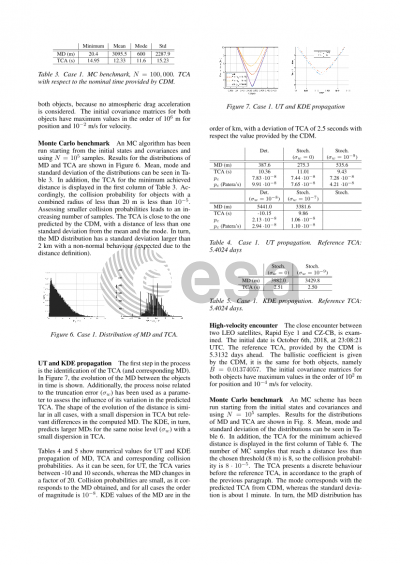
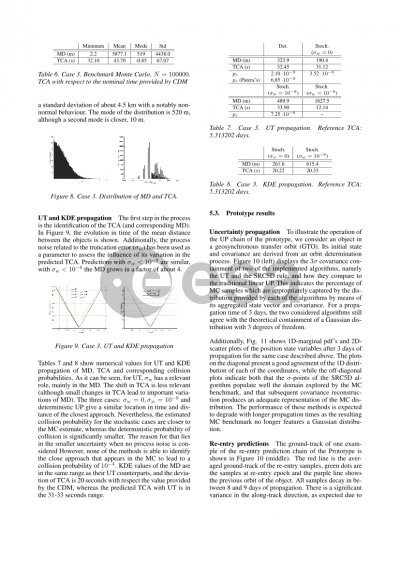
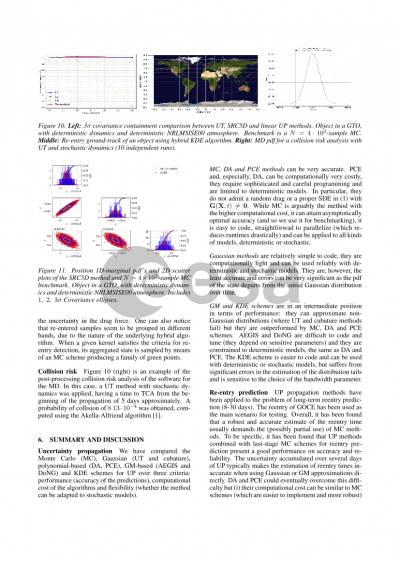
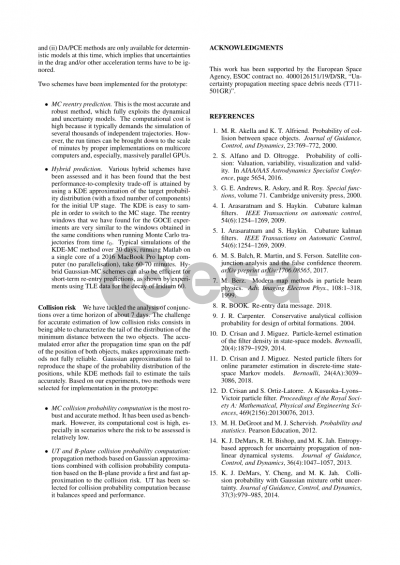
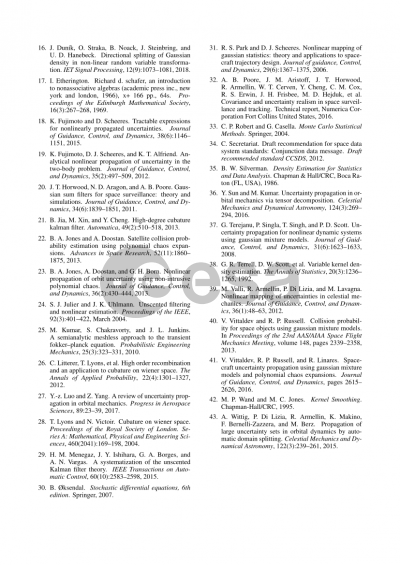