Document details
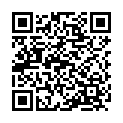
Abstract
Accurate knowledge of the orbits of all Earth-orbiting objects is required to enable space traffic management for safe space operations. However, due to the limited accuracy of dynamical models and orbital tracking data, orbit estimates are often not sufficiently accurate to avoid the problem of probability dilution in satellite conjunction analysis.
Atmospheric drag is the largest source of error in orbit determination and prediction for low Earth orbits. Most of the uncertainty stems from inaccurate knowledge of atmospheric density [1]. The uncertainties in the density are due to errors in both the model and the input, such as space weather indices. For orbit determination and short-term orbit prediction (<2 days) errors due to model inaccuracy tend to dominate, whereas errors due to inputs become dominant for longer orbit prediction when predicted space weather data are used. Therefore, the accuracy of orbit determination can be improved by reducing the error of density estimates. This can be achieved by calibrating density models or by estimating thermospheric densities directly using orbital tracking data. Such approaches can significantly reduce the bias and uncertainty of atmospheric densities.
Thermospheric density corrections have been computed in the past using two-line element (TLE) data by comparing observed and estimated ballistic coefficients [2] or comparing observed and estimated changes in the orbital mean motion [3]. Recently, a new technique for density estimation and prediction was developed by deriving and applying reduced-order models for the thermospheric density [4,5]. The developed reduced-order models have been used with Kalman filters to accurately estimate the global thermospheric density through data assimilation of density and orbital data [6,7,8]. Density estimation was demonstrated by assimilating accelerometer-derived density measurements [6], TLE data [7] and radar and GPS tracking data [8]. In particular, it was shown that density estimates based on TLE data are more accurate than the JB2008 and NRLMSISE-00 models when compared with CHAMP and GRACE accelerometer-derived and SWARM GPS-derived densities [7,8]. Moreover, the technique allows for uncertainty quantification of the density estimates as well as prediction of densities and their uncertainty [9].
In this paper, we assess the improvement of orbit determination accuracy due to the use of estimated densities obtained using reduced-order models. First, we estimate thermospheric densities using TLE data and quantify the uncertainty in the estimated density by comparison against accurate density data. Then the densities are used for orbit determination and the resulting orbit solution is compared against accurate ephemeris and against solutions obtained by applying empirical density models. In addition, we compare the statistics of series of estimated ballistic coefficients obtained using estimated and modelled densities to analyze the effect of density calibration on estimated ballistic coefficients. Finally, the accuracy of orbit predictions is assessed and their use for conjunction assessment considering density uncertainties is demonstrated.
References:
1. National Research Council (2012), Continuing Kepler’s Quest: Assessing Air Force Space Command’s Astrodynamics Standards, National Academies Press, Washington, D.C., 2012.
2. Cefola, P. J., Proulx, R. J., Nazarenko, A. I., & Yurasov, V. S. (2004). Atmo- spheric density correction using two line element sets as the observation data. Advances in the Astronautical Sciences, 116, 1953–1978.
3. Picone, J., Emmert, J., & Lean, J. (2005). Thermospheric densities derived from spacecraft orbits: Accurate processing of two-line element sets. Journal of Geo- physical Research: Space Physics, 110(A3).
4. Mehta, P. M., & Linares, R. (2017). A methodology for reduced order modeling and calibration of the upper atmosphere. Space Weather, 15(10), 1270–1287.
5. Mehta, P. M., Linares, R., & Sutton, E. K. (2018). A quasi-physical dynamic reduced order model for thermospheric mass density via hermitian space- dynamic mode decomposition. Space Weather , 16 (5), 569–588.
6. Mehta, P. M., & Linares, R. (2018). A new transformative framework for data as- similation and calibration of physical ionosphere-thermosphere models. Space Weather, 16(8), 1086-1100.
7. Gondelach, D. J., & Linares, R. (2020). Real-time thermospheric density estimation via two-line element data assimilation. Space Weather, 18(2), e2019SW002356.
8. Gondelach, D. J., & Linares, R. (2020). Real-time thermospheric density estimation via radar and GPS tracking data assimilation. In Proceedings of the AAS/AIAA Astrodynamics Specialist Conference, South Lake Tahoe, CA, August 9-13, 2020.
9. Gondelach, D. J., & Linares, R. (2020). Atmospheric density uncertainty quantification for satellite conjunction assessment. In 30th AIAA/AAS Space Flight Mechanics Meeting, Orlando, FL, January 6-10, 2020.
Preview
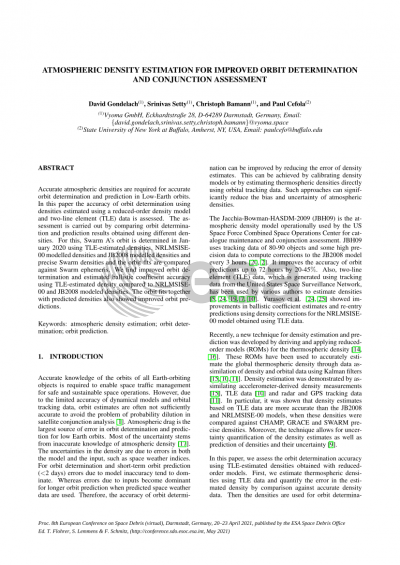
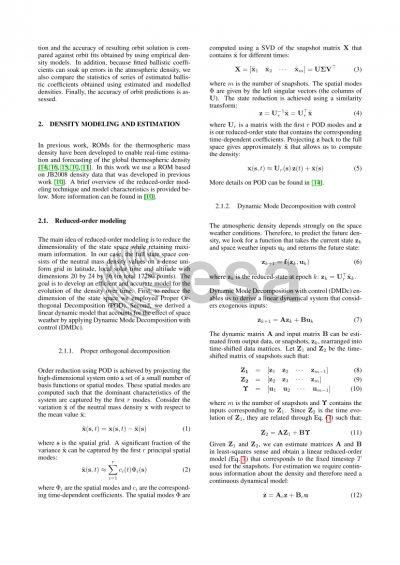
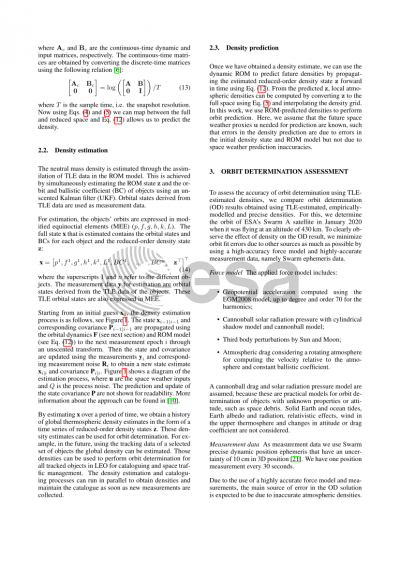
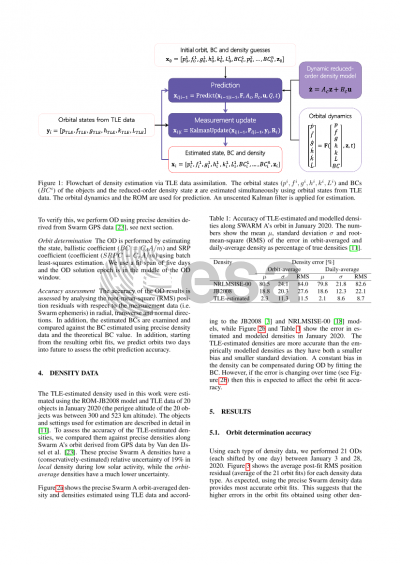
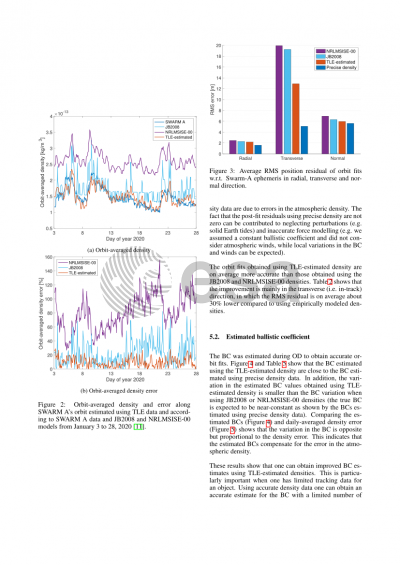
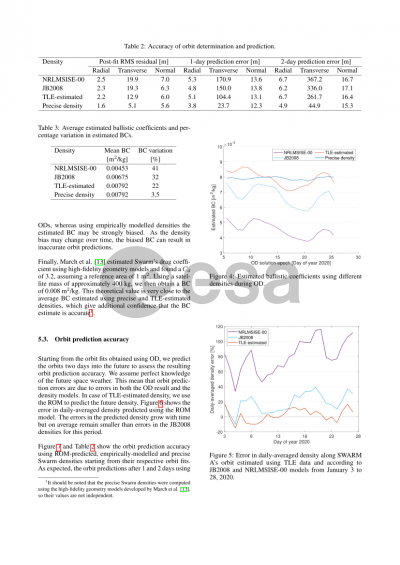
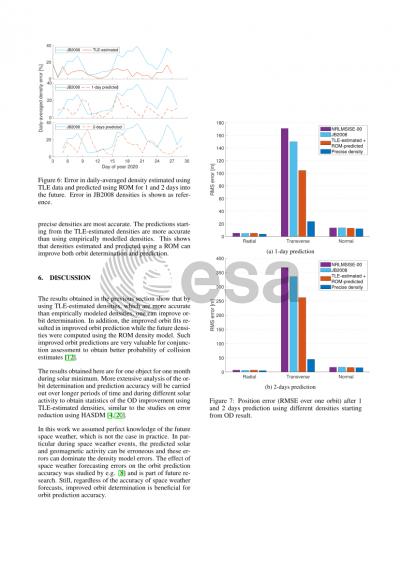
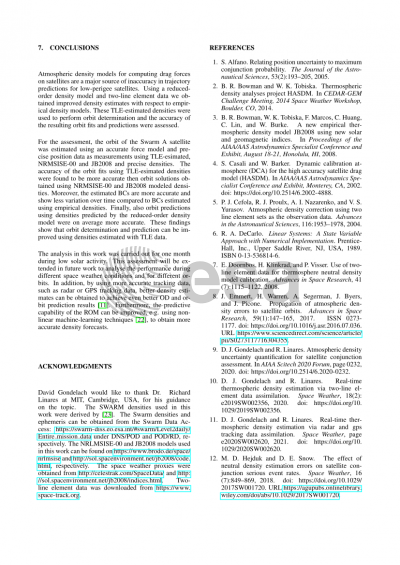
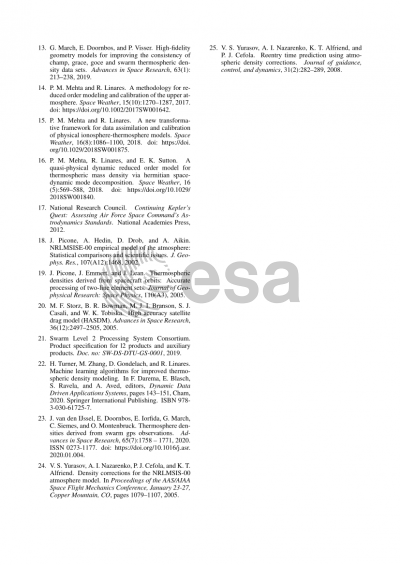