Document details
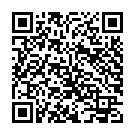
Abstract
The provision of many of the Space Surveillance and Tracking (SST) and Space Traffic Management (STM) services depends on how well characterized is the uncertainty of the orbits of the catalogued resident space objects (RSOs), what is commonly known as uncertainty realism. For instance, uncertainty misrepresentation, either in orientation or size, can lead to differences of more than an order of magnitude in the computation of the probability of collision between two RSOs.
Assuming the state of an RSO can be represented by Gaussian random variables, the estimation of uncertainty is described by the covariance matrix. One of the main sources of uncertainty in orbits of RSOs is the uncertainty of dynamical models used in the orbit determination process, which is not captured by the so-called noise-only covariance, as it only includes the measurement error contribution. Scaling techniques are sometimes used to scale the covariance for improved covariance realism, as in CNES’ Scaled Probability of Collision method or in CARA’s Probability of Collision Uncertainty method. However, these scaling approaches are not supported by physical interpretation or models. For instance, it is arguable whether it would make more sense to use a scale factor only in the along-track direction, instead of global one affecting the whole covariance matrix, provided that the main uncertainty comes from the atmospheric drag in the along-track direction.
The aim of this work is to present a methodology to improve the covariance realism of orbit determination processes through the classical theory of consider parameters in batch least-squares estimation. Consider parameters can be included in the underlying dynamical models, such as atmospheric force, solar radiation pressure force or measurement models, providing the means to account for additional uncertainty coming from errors in those dynamical and measurement models, while maintaining an unbiased estimation. However, realistic variances of these consider parameters are not known. To solve this issue, a methodology is proposed to estimate the variances of consider parameters considering an objective function closely related to covariance realism: under a Gaussian assumption the square of the Mahalanobis distance of orbital differences must follow a Chi-square distribution. This property can be used to estimate the variance of the consider parameters in a parameter estimation process by forcing the observed Mahalanobis distance distribution fit the expected theoretical distribution. Error models used in this case consist in a constant relative error model, but other error models are also allowed in the formulation, such as a time and space correlated error model.
In this work, the methodology is applied to the atmospheric drag force and range bias model errors in Low Earth Orbit (LEO) using Sentinel-3A and one year of real radar data as an example of use. Results show that important improvements are achieved in terms of covariance realism. This is verified through containment tests, as well as analysis of Mahalanobis distances during the first few days (from 0 to 10 days) of propagation of orbit and covariance. Additionally, it is also shown that the variances of consider parameters included affect the covariances in two different ways. The variance of the range bias acts as a global scale factor on the whole covariance, while the variance of the atmospheric density error acts as a scale factor only in the along-track direction.
Preview
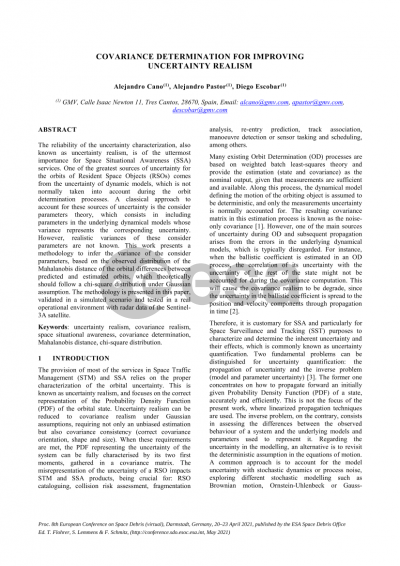
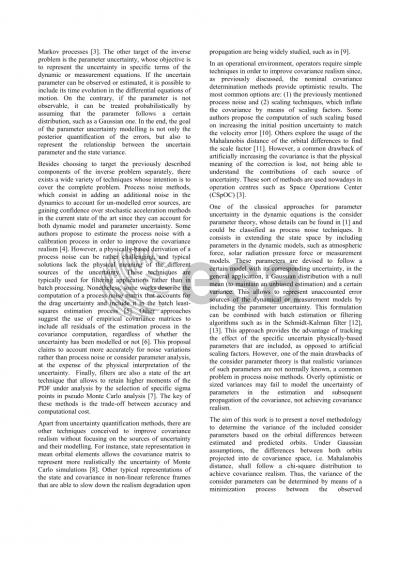
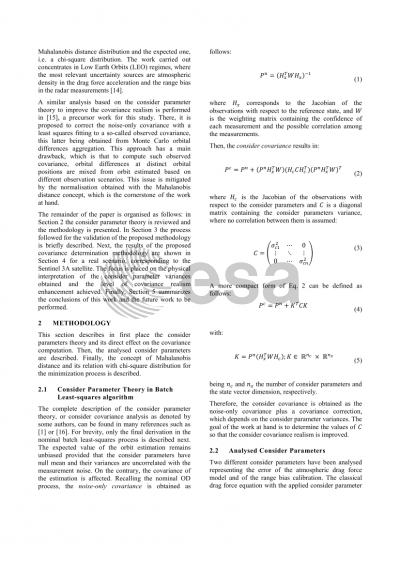
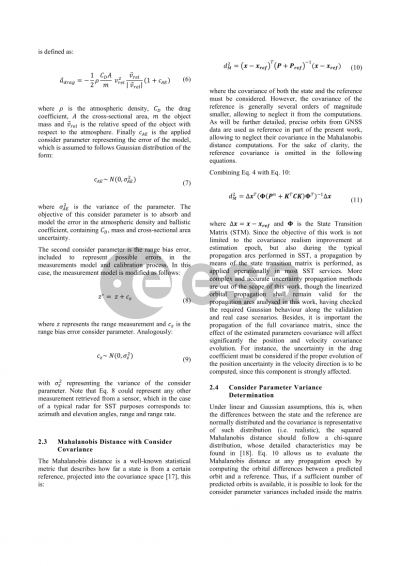
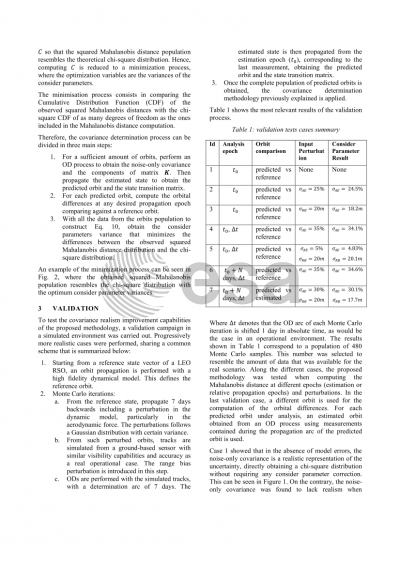
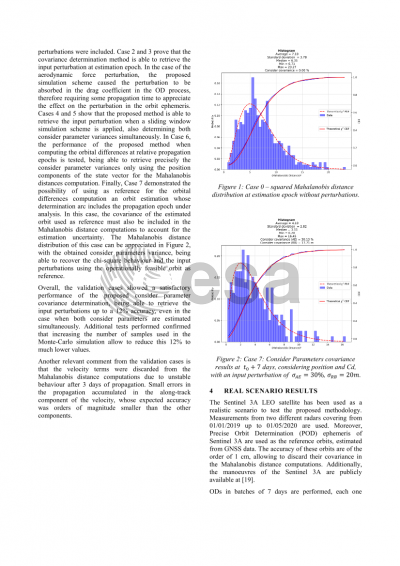
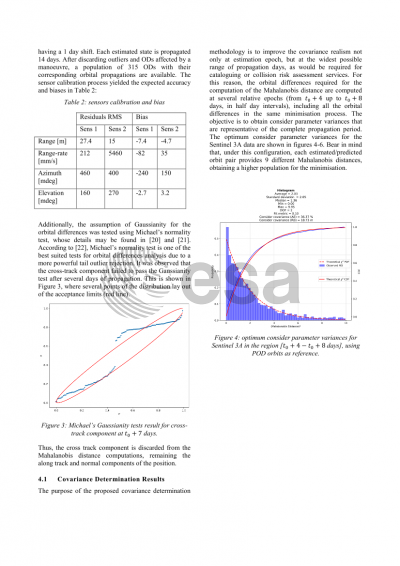
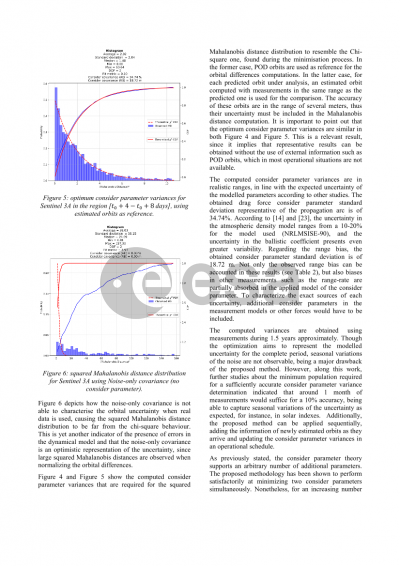
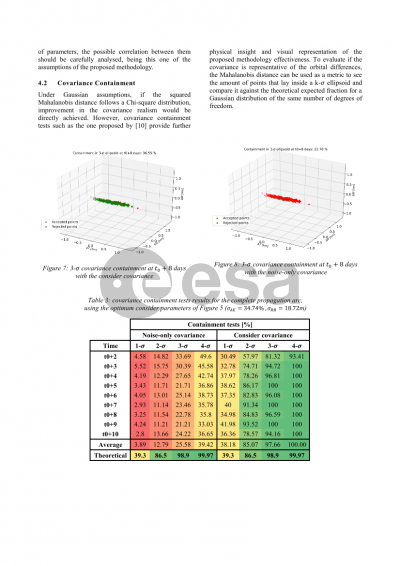
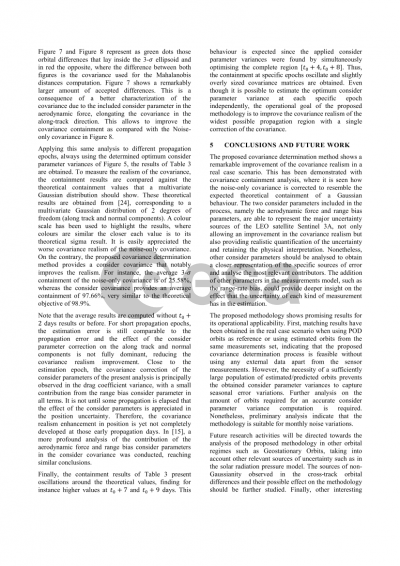
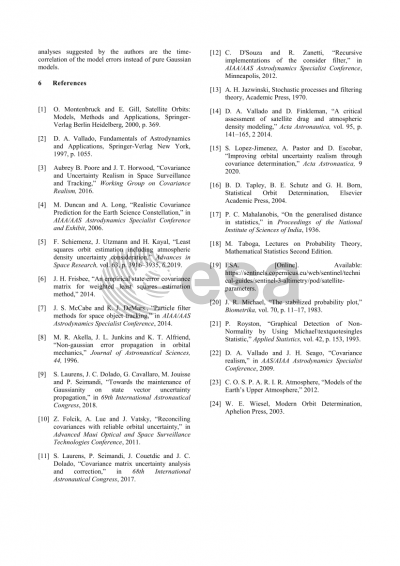