Document details
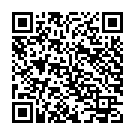
Abstract
The use of telescopes photometry for the identification and classification of resident space objects (RSOs) has been a standard practise since the advent of the satellite era, from photographic films to modern electronic CCD sensors. In the last few years, recording the photometry in time (lightcurves) is becoming more and more important as it can be utilised to determine detailed information on the operational status, attitude dynamics or platform configuration and surfaces characteristics. The algorithms traditionally applied to lightcurves have been mainly centred on determining the spin rate such as Fourier transforms, least squares spectral analysis, epoch folding, etc. The widespread growth of artificial intelligence (AI), in particular machine learning (ML) algorithms, opens up an alternative to the traditional methods and new possibilities of extracting the hidden information behind the lightcurves.
The power of machine learning on classification problems has been well documented for a variety of problems, from image classification to speech recognition or mood detection in texts, so its use in lightcurves seems to fit as a new field of applicability. Lightcurves can be used as fingerprints, identifying not only the attitude state, also the type of the platform, the attitude orientation, the geometry and elements (solar arrays and antennae), and even the failure on the deployment or orientation of those elements.
One of the main requirements for the success of a ML model is the availability of a large amount of input data for the training phase, usually, thousands of well-characterised samples per class. However, in the space domain, this volume of data generally represents an issue; either it is just impossible to obtain it or it is excessively expensive. Fortunately, light reflection models such as Phong model, extensively used in computer graphics, are well known and can be used to accurately simulate lightcurves by applying them to model designs representing space objects. This simulation can provide thousands of training samples with all possible variations (randomised) on the illumination conditions, attitude, configuration, geometry and surface characteristics, etc. Once the ML model is trained, it can be applied operationally to real lightcurves. The main advantage on training the ML model using simulated dataset is that there is not actual limitation on the features that can be trained, allowing, for example, training with faulty components, deployment or orientation, and new brand or even unseen platforms.
Initial tests performed, using purely simulated data, show that a fully connected dense neural network is able to identify platforms and attitude state from lightcurves with an accuracy of 95% with a small confusion between spinning satellites or very similar 3-axis stabilised platforms. These results fulfil a first part of the study objectives, demonstrating that the use of the lightcurves as fingerprints to recognise the platform and the attitude state is perfectly suitable. However, continuing these tests using actual data as a validation dataset for the trained algorithm has highlighted some defects on the training data that must be corrected, such as absence of data gaps and signal noise high enough in the training dataset. Once these differences on the modelling are overcome, the ML model trained using simulated data is expected to reach a similar performance using real lightcurves.
Preview
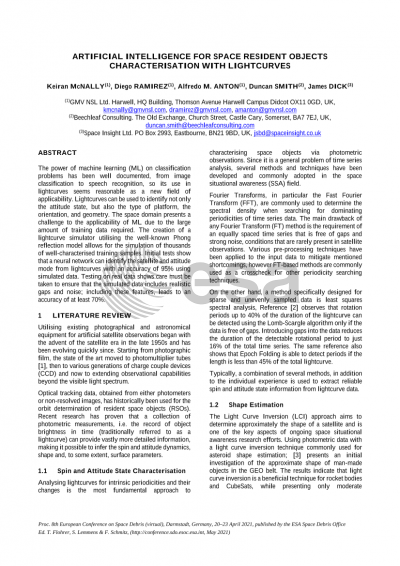
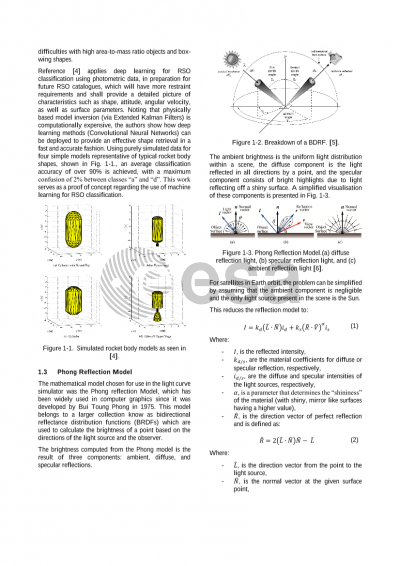
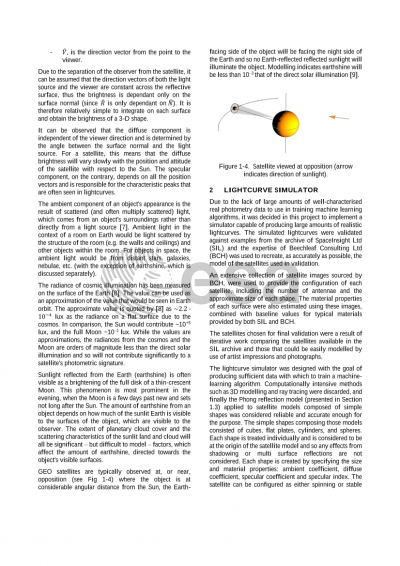
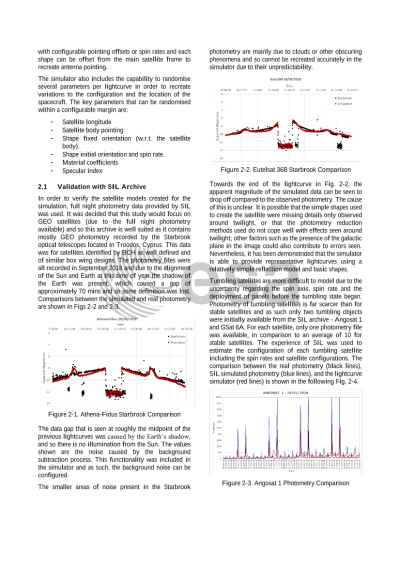
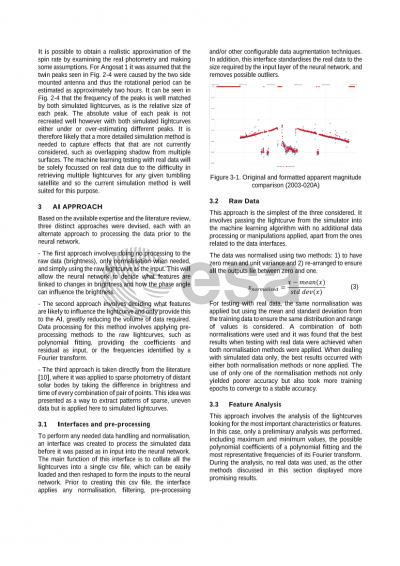
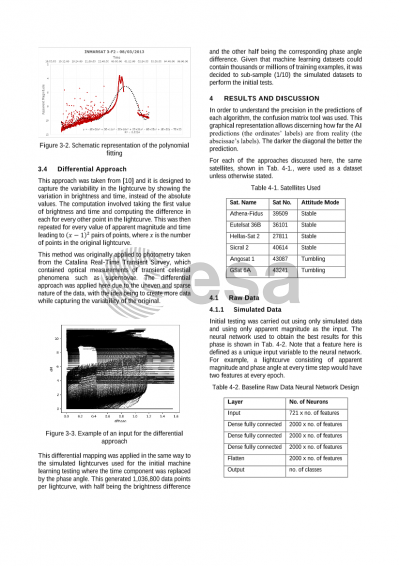
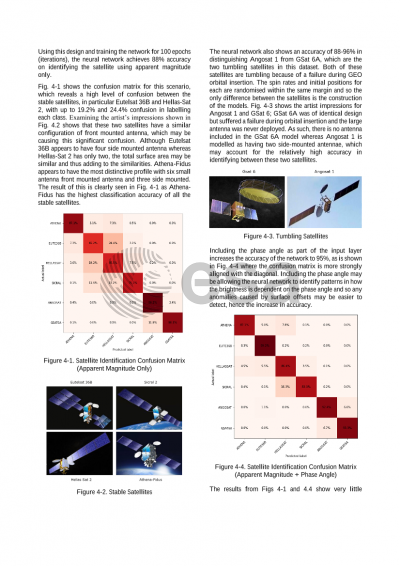
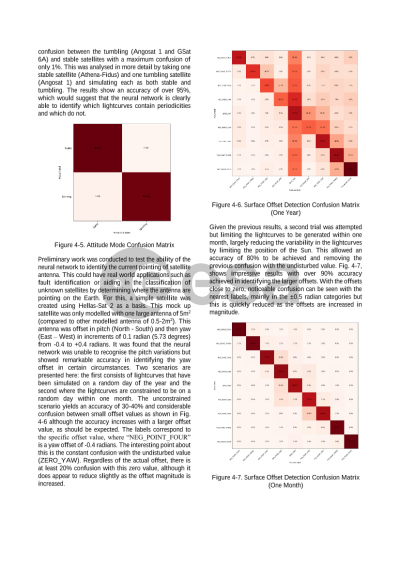
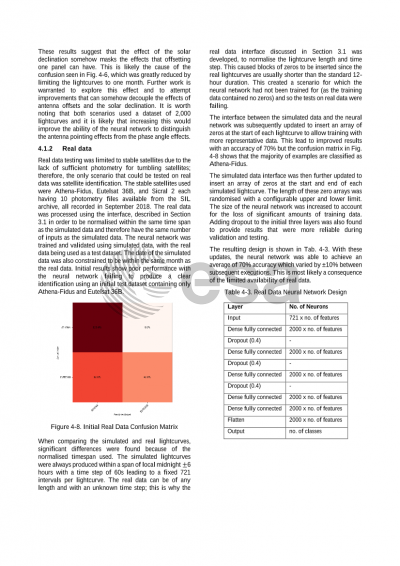
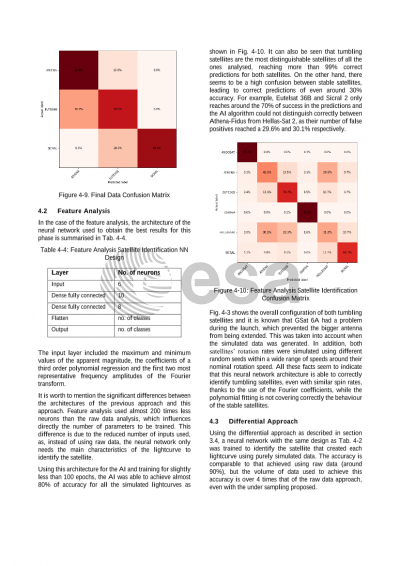
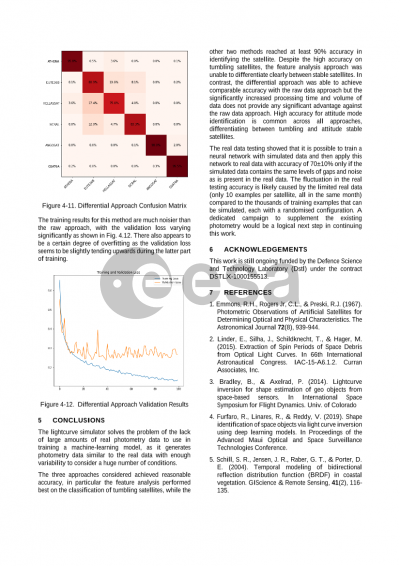
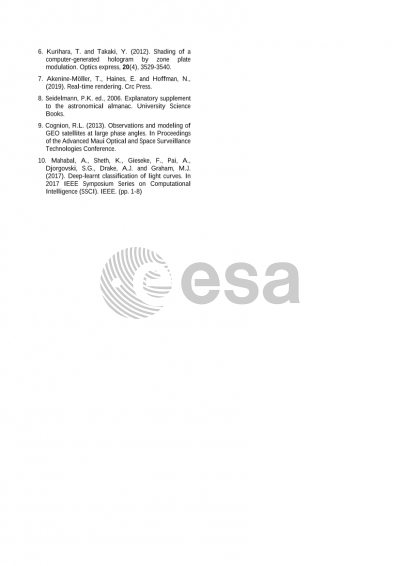