Document details
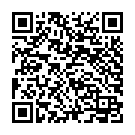
Abstract
The space objects population is expected to continue its rapid growth due to many factors such as the mega-constellations and a wider accessibility to the space, but also from fragmentation events, whose occurrences are increasing. In such situation, Space Situational Awareness (SSA) products and services are of the utmost relevance to maintain the safety and sustainability of the spacecraft operations. Most SSA products rely in the knowledge of both the state of the Resident Space Object (RSO) and its associated uncertainty, or in other words, the Probability Density Function (PDF) that describes the state of the object. For many SSA products such as collision risk analysis or catalogue maintenance, the uncertainty of the state is represented as a covariance matrix, retaining only up to the second order moment of the state PDF. This choice is generally based on a trade-off between accuracy and computational efficiency. Under this assumption, it is necessary to consider the different sources of uncertainty that are present in the space environment in order to improve the covariance realism. For these reasons, it is necessary to develop efficient and accurate methodologies to characterize the PDF of the state attending to the different sources of uncertainty, which is known as Uncertainty Quantification (UQ).
In this work, we describe a covariance propagation and determination methodology based on linear theory that allows to analyze the effect of time-correlated errors on the covariance evolution. Linear covariance propagation theory can consider the impact of the uncertainty of dynamical parameters on the evolution of the state by means of the so-called sensitivity matrix, resulting from the integration of the variational equations, and the consider parameter theory. However, the correlation between parameters is not generally considered during linear propagation. In that line, we present here a method to compute efficiently the effect of a series of uncertain time-correlated parameters on the covariance. First, an auto-regressive function of order 1 is used to model the time correlation in the sequence of parameters, controlling the correlation strength by means of the power of the noise and the time-scale of the correlation. Second, the properties of the variational equations are exploited in order to compute the sensitivity matrix, in this case, of a series of multiple uncertain parameters of the same kind (e.g. atmospheric drag errors) from the case where a single constant uncertain parameter is considered. This formulation allows to analyze the impact of stochastic perturbations on the covariance evolution without requiring the integration of stochastic equations of motion, and allowing to derive different perturbation models ranging from Gaussian noise up to a constant perturbation depending on the selected correlation time-scale. The work presented here describes in first place the proposed formulation. Then, the performance and accuracy of the method is compared against Monte Carlo based analysis with stochastic dynamics. Finally, the methodology applied to scenarios of covariance propagation and determination applicable to space debris activities, namely the atmospheric density uncertainty or manoeuvre uncertainty sources such as the manoeuvre modulus or thruster pointing errors.
Preview
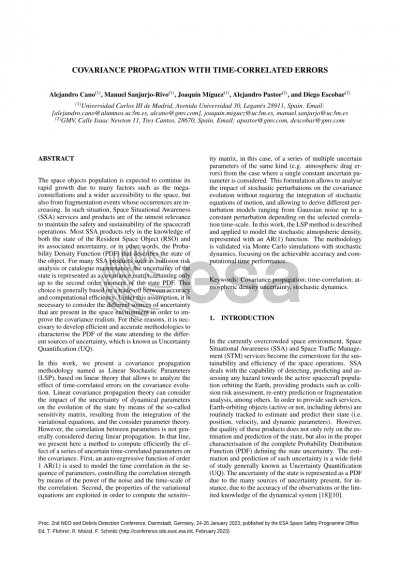
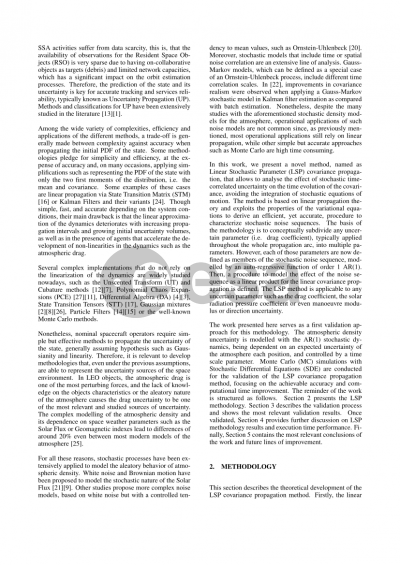
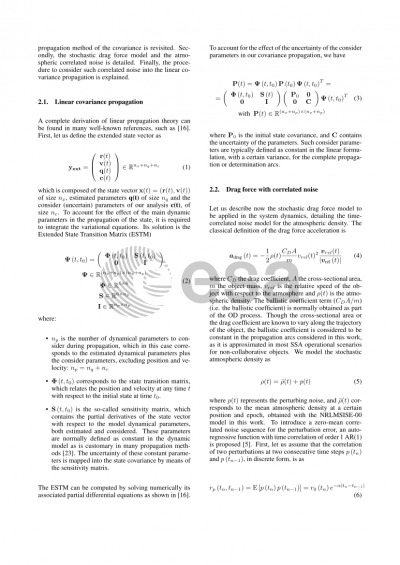
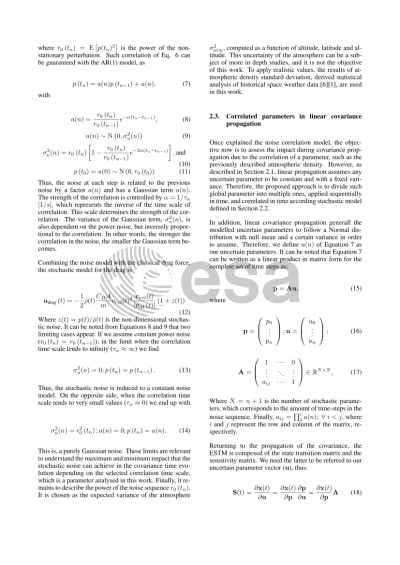
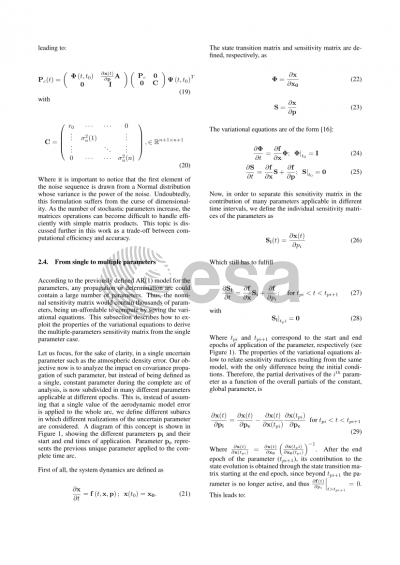
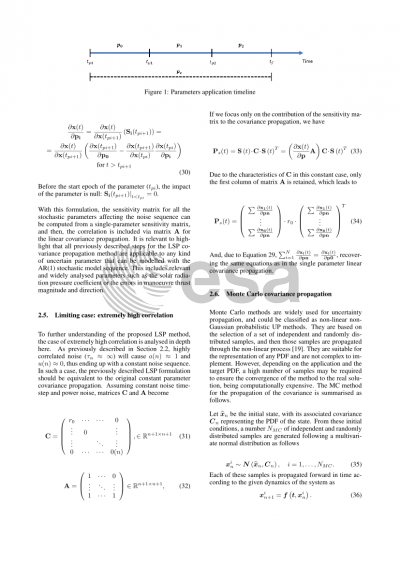
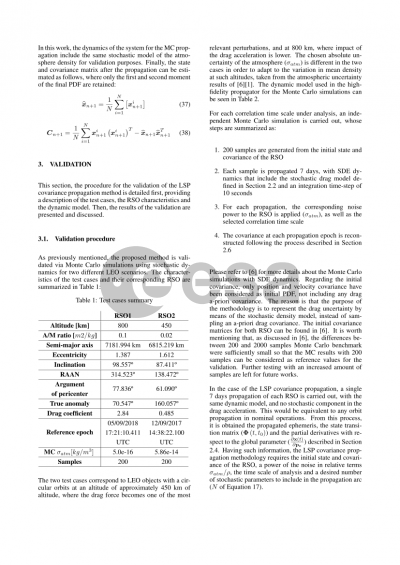
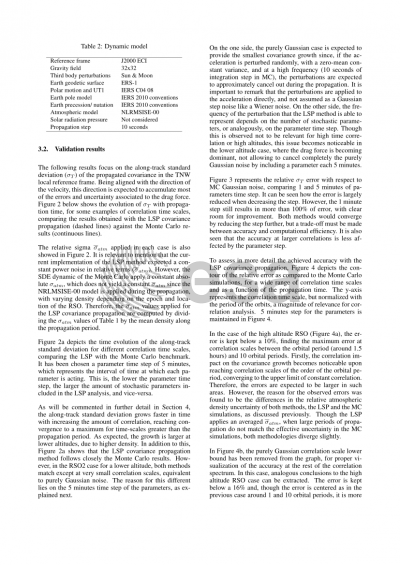
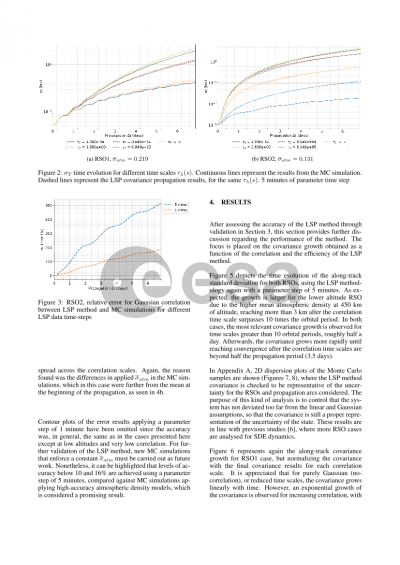
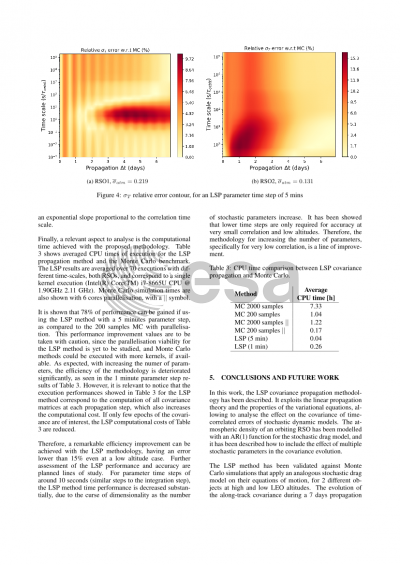
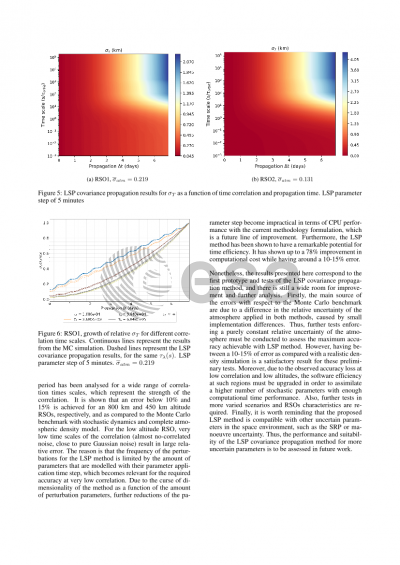
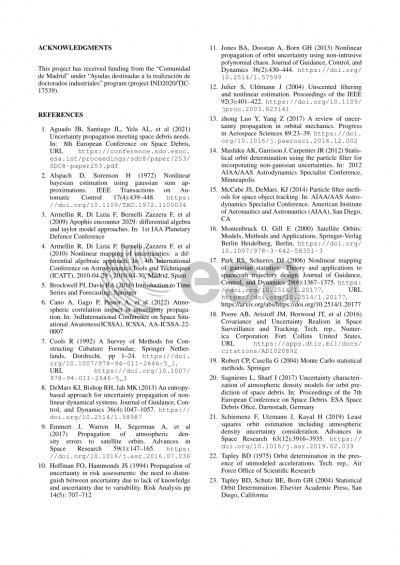
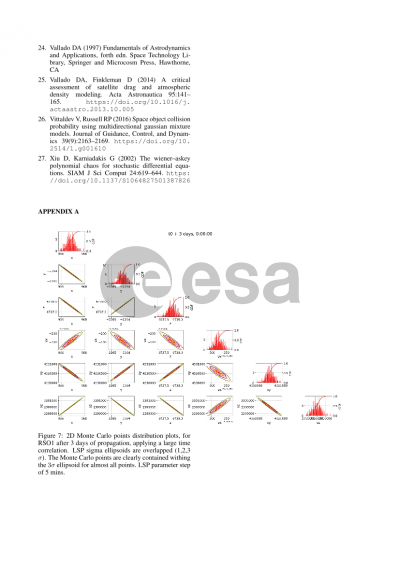
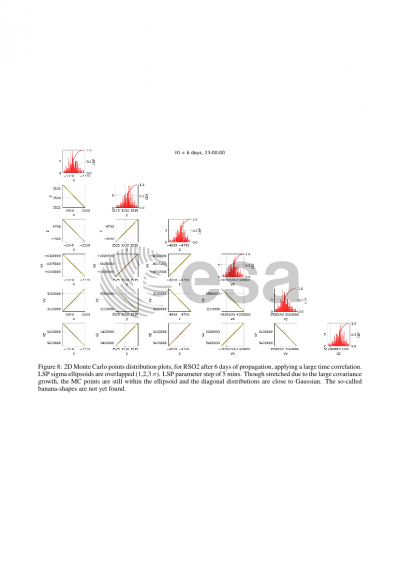