Document details
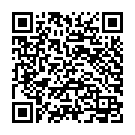
Abstract
In recent years low-thrust propulsion has become a credible alternative to standard chemical propulsion for many different applications, ranging from orbital transfers to routine operations of small LEO satellites. This has led to an increase in the frequency of manoeuvres as well as their duration for missions employing such devices. Hence, it becomes necessary to have accurate and fast methods to improve the uncertainty realism of the propagated orbits of manoeuvrable satellites with low-thrust propulsion within a space objects catalogue. Having more realistic uncertainties can benefit the measurement correlation process and allows a more accurate estimation of the collision probability in case of conjunction events, which could avoid unnecessary collision avoidance manoeuvres or fatidic collisions.
In light of these considerations, it becomes a pressing issue to accurately quantify state uncertainties under the effect of low-thrust noise in a timely manner. The most general solution to this problem can be provided by a full solution of a high dimensional and nonlinear partial differential equation, the Fokker-Plank equation, or by carrying out Monte Carlo simulations. However, both methods have a considerable computational cost, owing to the entire statistical description of a trajectory. To solve this issue, many different techniques for orbital uncertainty propagation have been developed in recent years. Probabilistic methods infer statistical properties from the propagation of a selected number of samples. On the other hand, dynamics-based procedures rely on the knowledge of the system dynamics to propagate statistical moments.
This work proposes an analysis of several uncertainty propagation methods. The presented approaches belong to different families of methods (i.e., dynamics-based and probabilistic).
The proposed dynamics-based methods rely on carefully mixing differential algebra (DA) with Gaussian Mixture Models in different ways. First, by propagating uncertainties through a DA scheme while leveraging an adaptive Gaussian Mixture to describe the distributions. Secondly, by extending the DA propagation with an expansion of the embedded covariance inflation linked to the process noise.
On the other hand, probabilistic methods consider stochastic dynamic models that allow us to include the thrust uncertainty in a seamlessly manner. A time-dependent uncertainty model for both thrust acceleration and pointing is proposed. The integration of the resulting stochastic differential equations is done using a stochastic Runge-Kutta, compatible with the thrust uncertainty model. The uncertainty propagation, in turn, is performed using Unscented Transformed, cubature schemes and Kernel Density Estimator methods.
The results of the application of the presented methods to different scenarios are presented in this work. To test applicability across a wide spectrum of missions, the scenarios analyzed include orbit maintenance and Electric Orbit Raising scenarios in different orbital regimes.
The implemented techniques and scenarios have been developed in the framework of the ESA-funded ELECTROCAM contract, awarded to the consortium formed by GMV, Politecnico di Milano, and Universidad Carlos III de Madrid.
Preview
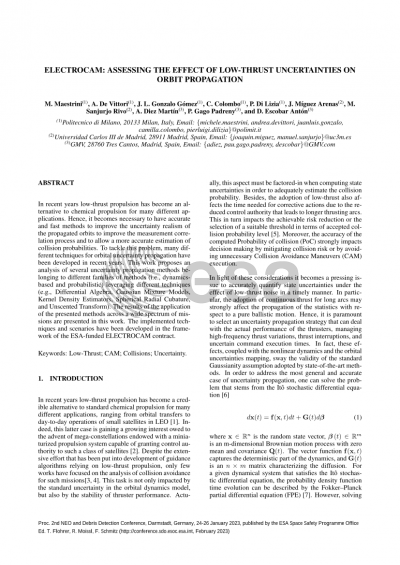
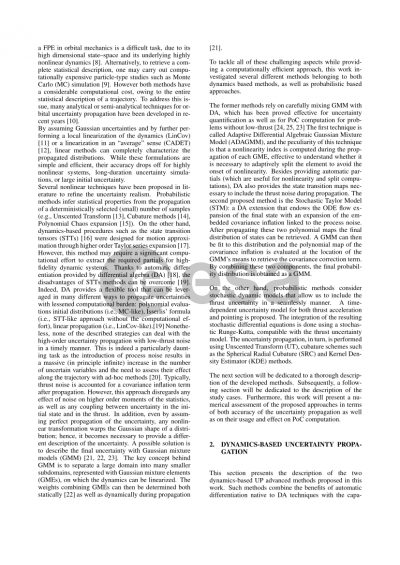
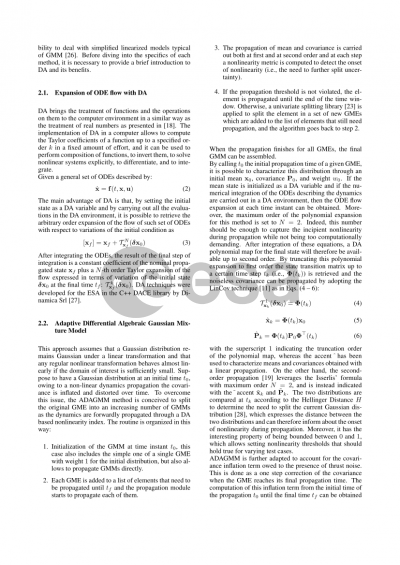
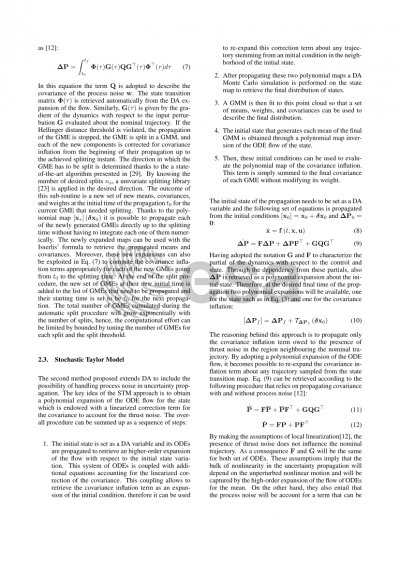
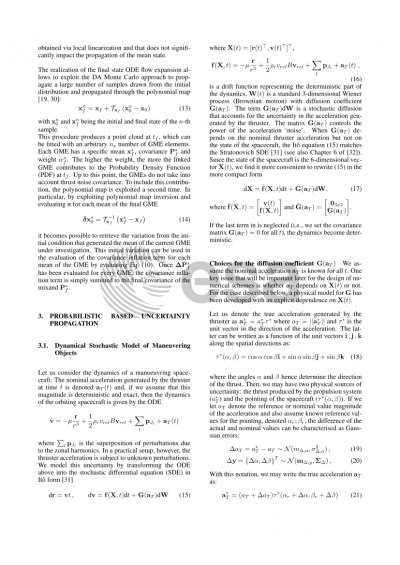
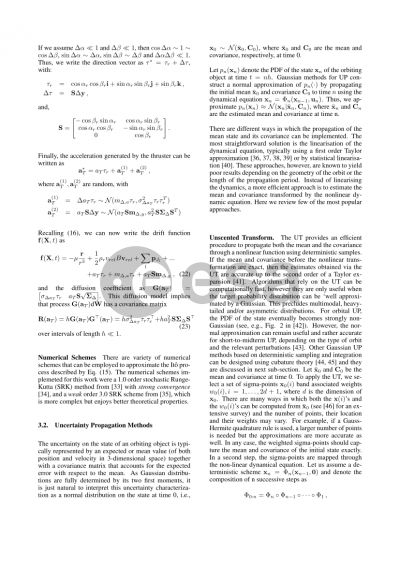
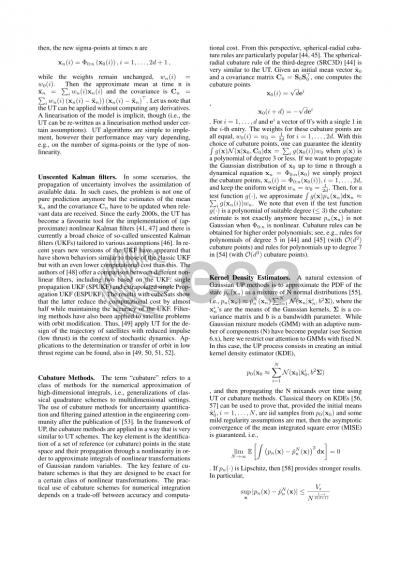
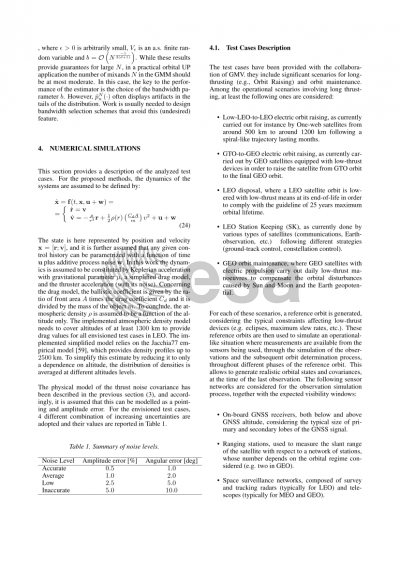
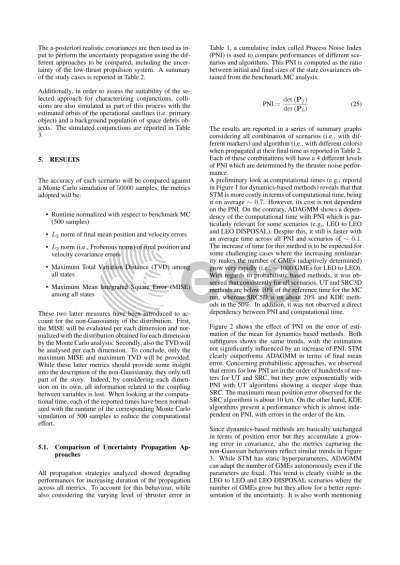
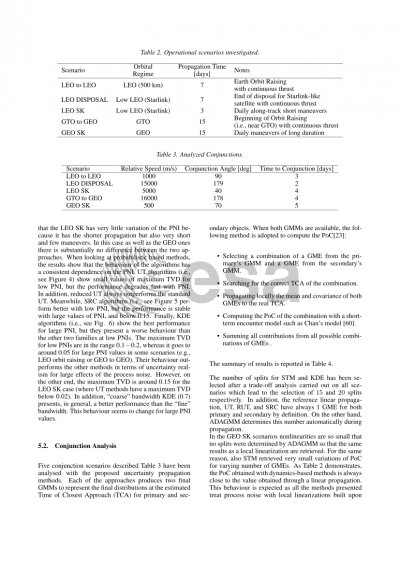
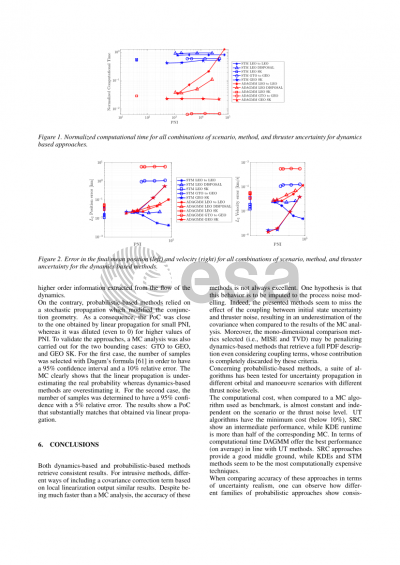
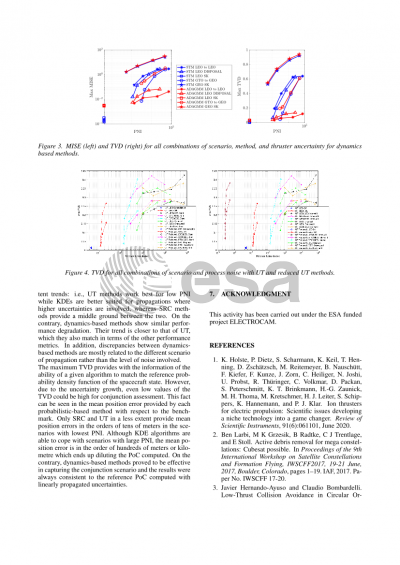
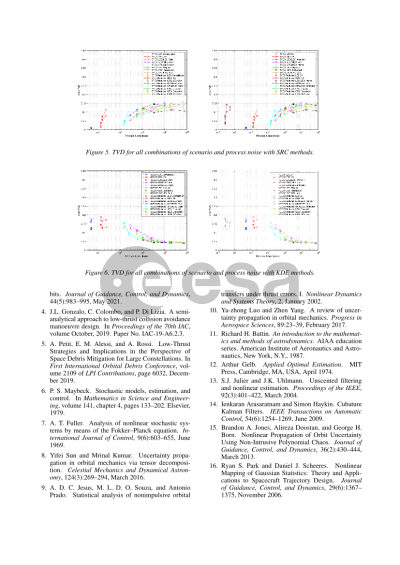
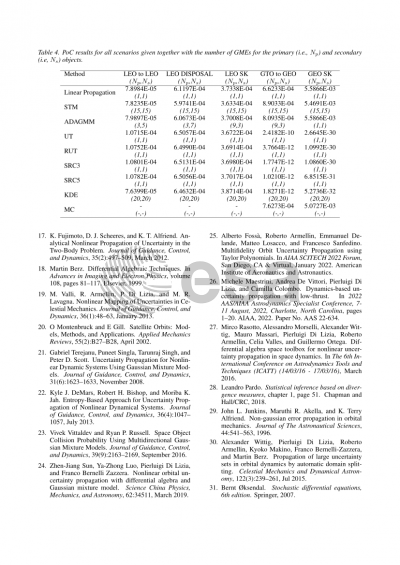
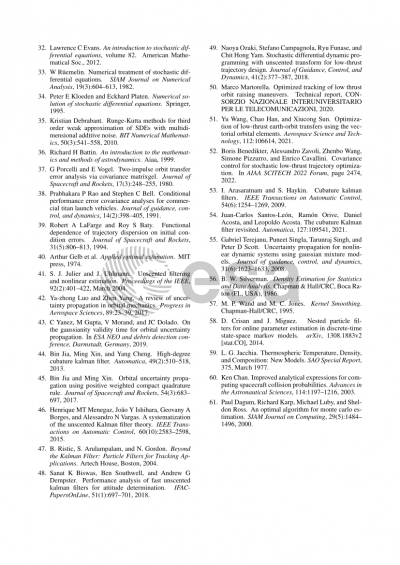