Document details
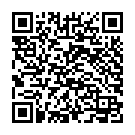
Abstract
A large number of resident space objects (RSO) is located in Earths vicinity. Today, 19206 RSOs, thereof 2027 are active satellites, are trackable. Nevertheless, the number will increase by thousands of additional satellites as the so-called mega constellations are established. This will lead to an increased risk of collisions with other RSOs. The RSOs can be detected using telescope and radar sensors. Detections of RSOs always come with inaccuracies due to measurement noise. Extrapolation methods for orbit determination in turn suffer from model inaccuracies, which lead to a decay of the orbit information over time. Frequent updates of the orbital states are needed to compensate for the degradation. Methods within the field of statistical orbit determination (SOD) are used to decrease the error of the detection and improve the latter.
One particular method is the usage of Kalman filters, where a dynamical model of the specific problem is compared against sensor measurements. In the following, a specific type of Kalman filter is investigated, the ensemble Kalman filter (EnKF). The EnKF uses a set of randomly chosen states based on a probability density function (ensemble) to approximate the uncertainty of the state vector. The ensemble is propagated and updated using the detection at the respective epoch. It is tested within a simulation environment and compared to the Unscented Kalman filter (UKF) to evaluate, whether it is possible to use the EnKF for orbit determination. Thereby, the number of ensembles and the number of detections is varied.
Preview
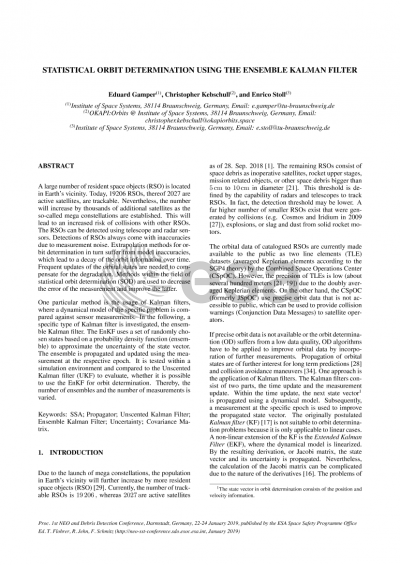
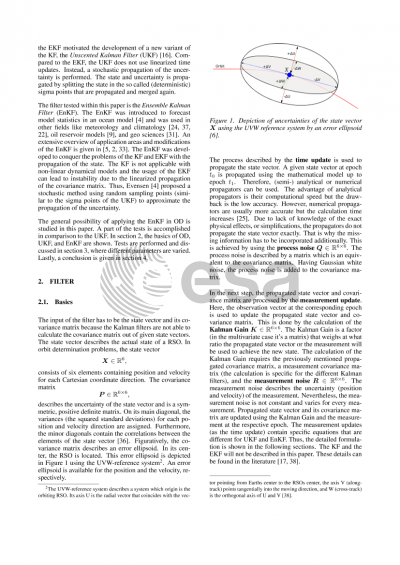
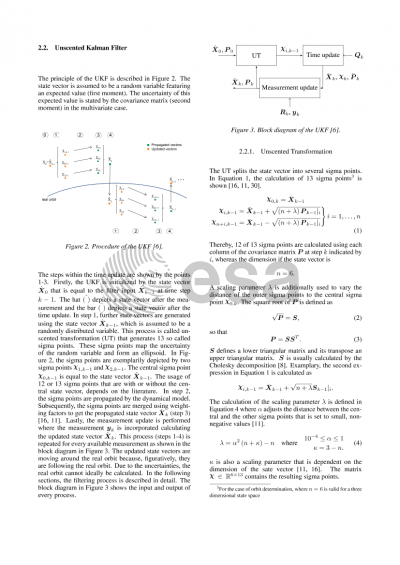
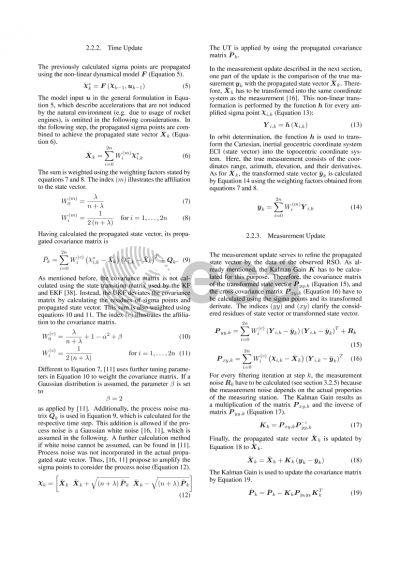
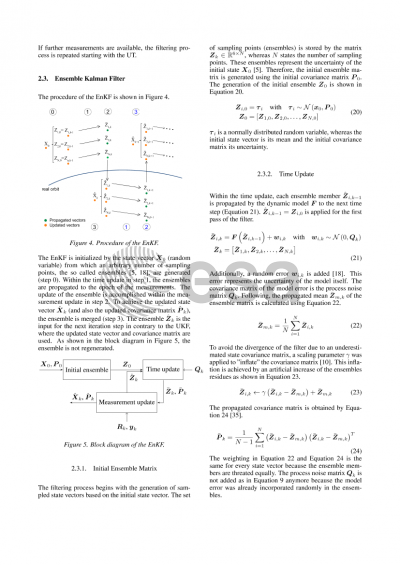
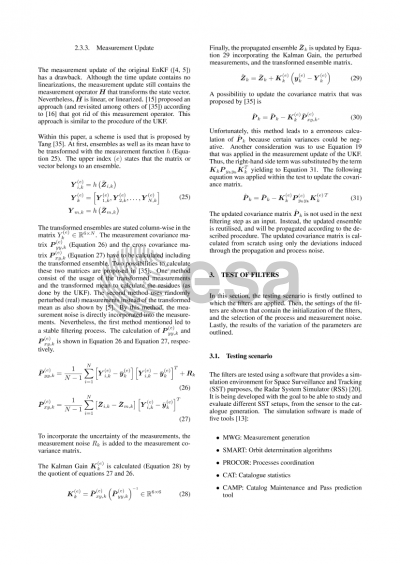
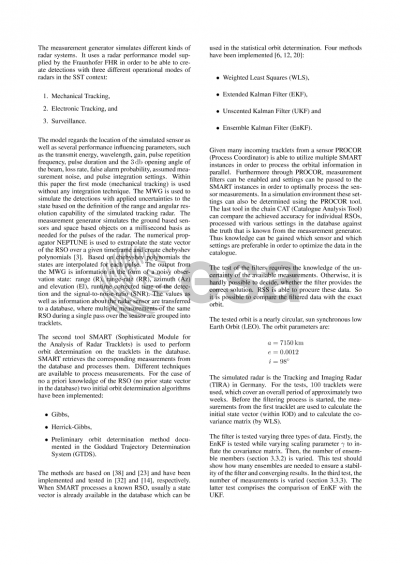
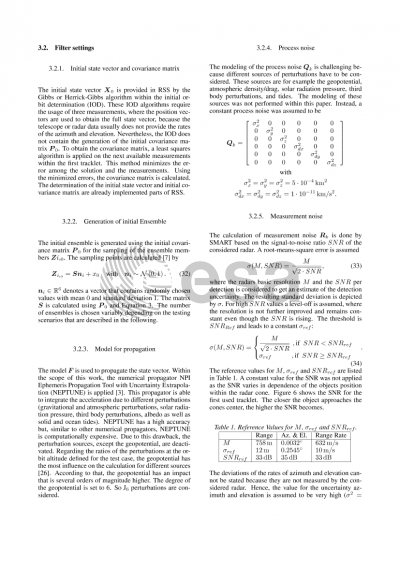
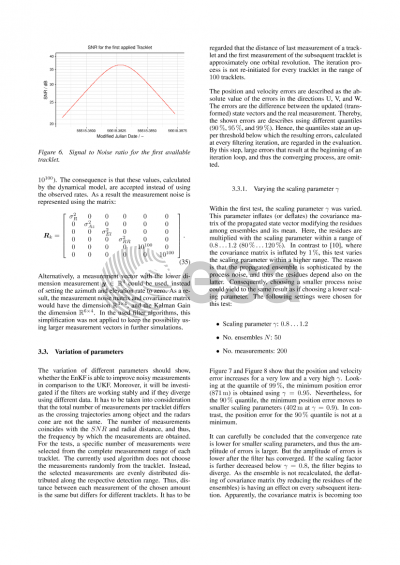
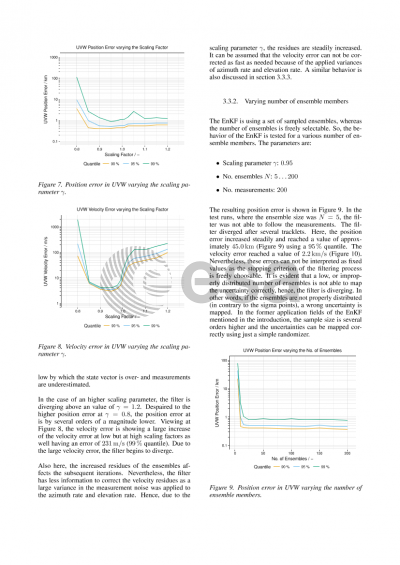
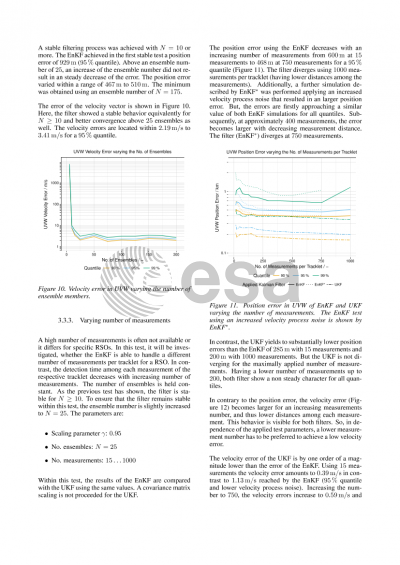
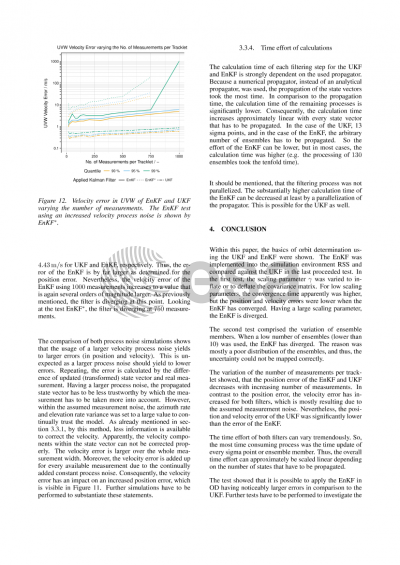
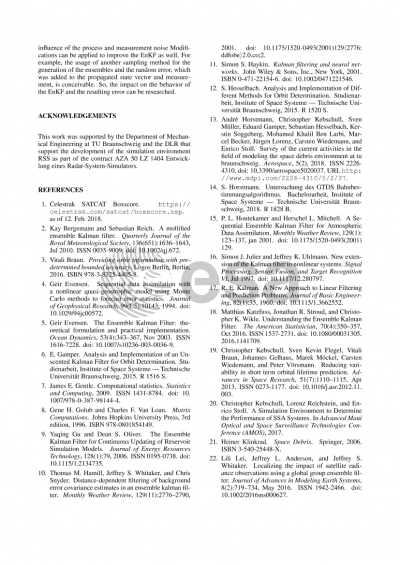
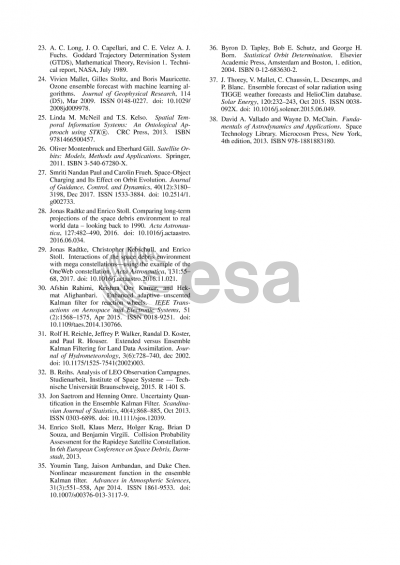