Document details
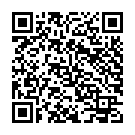
Abstract
Tracking algorithms provide a fundamental element of space domain awareness by producing up-to-date information on the state (e.g., position and velocity) of objects of interest, including operational satellites and trackable fragments of space debris. The information desired is typically an estimate of the state and an understanding of the uncertainty in this estimate. One way to obviate the need for advanced tracking algorithms is to attempt to overcome any lack of knowledge through the deployment and acquisition of an ever-increasing number of sensors to collect more data to process. Space domain awareness is--almost by definition--a realm in which it is impossible to dominate the problem through more information; instead, it becomes necessary to embrace uncertainty and advance the state of the art in an alternative manner. The need for advancements in tracking algorithms for functional, decommissioned, and defunct space objects is greatly exacerbated by the growing pressure being placed on the space domain by the presence of large satellite constellations and the growing interest in the cislunar orbital regime.
Approaches for tracking space objects are typically comprised of two phases: prediction and inference. The prediction phase leverages dynamics modeling to provide an estimated state and uncertainty prior to the incorporation of new measurement information acquired from ground-based or space-based sensors. The current state of the art focuses on prediction in the presence of nonlinear dynamics, and it has been shown that Gaussian mixture representations are better able to approximate the underlying probability distribution for space object tracking. The inference phase ingests the prediction and new measurement observations to produce an improved estimate and uncertainty of the state of the object. The focus of the current work is to develop and analyze a new approach for approximate Bayesian inference for space object tracking that leverages Gaussian mixture representations of the uncertainty.
In this work, the Bayesian update is transformed from a single-point solution to a multi-point (or even a continuous) solution through a homotopic continuation of Bayes' rule via manipulation of the measurement likelihood function. The resulting filtering approach is shown to be implementable as a recursion of partial updates that better approximates the true Bayesian posterior in the presence of nonlinear measurements. An adaptive approach to approximate Bayesian inference is formulated by optimizing the multiple points used in the recursion of partial updates that minimizes the information divergence between the approximate solution and the true Bayesian posterior. The resulting method is applied to representative problems in near-Earth and cislunar space domain awareness through simulation studies. Results are presented that highlight the power of the method, especially in the presence of non-Gaussian uncertainties that can arise from long propagation periods and in the presence of extremely short-arc data. The method is shown to exhibit resiliency to algorithm failure and to produce superior tracking performance in these types of scenarios.
Preview
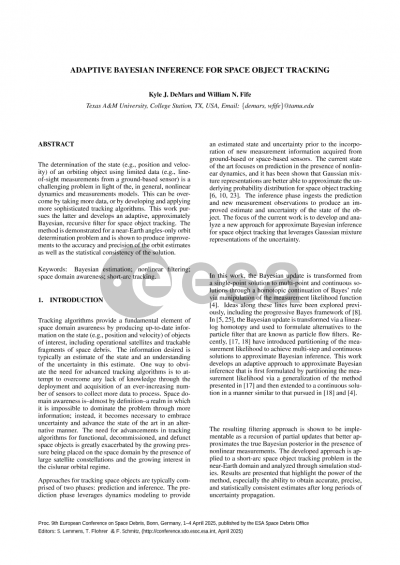
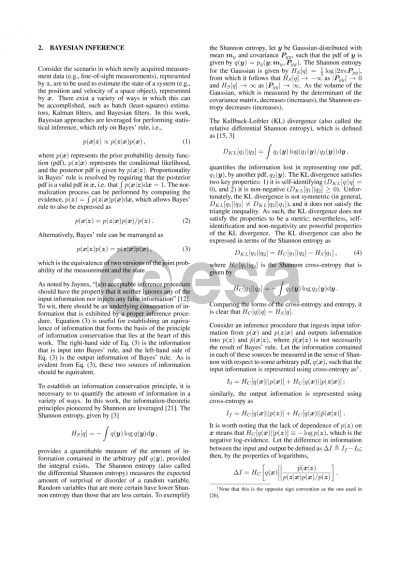
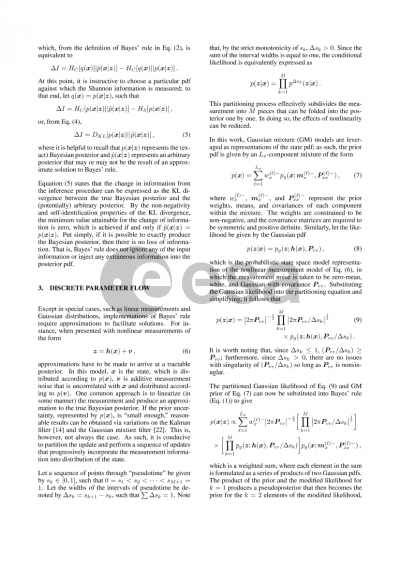
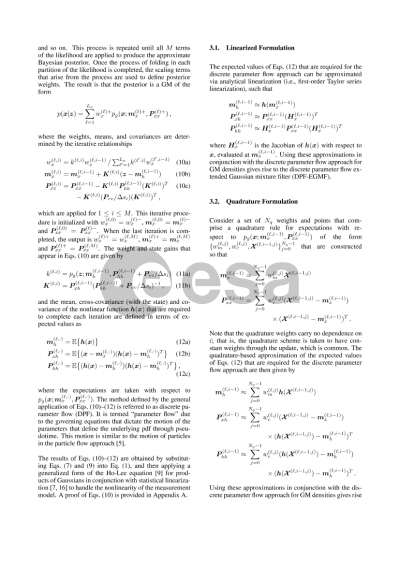
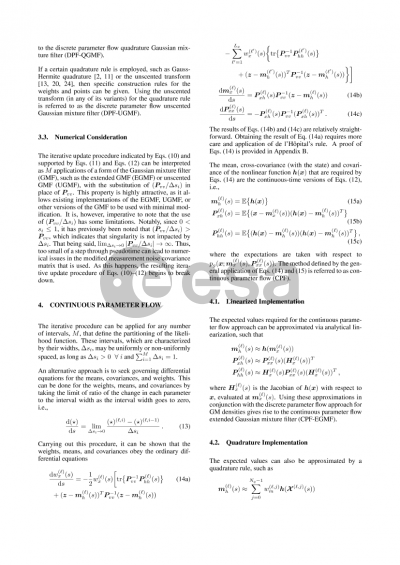
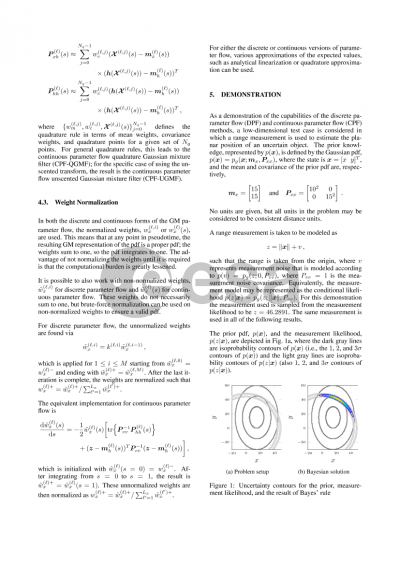
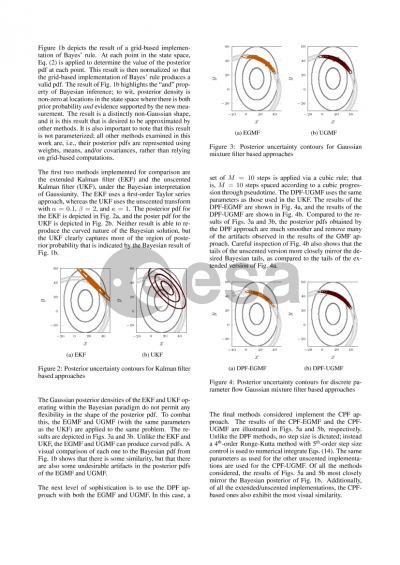
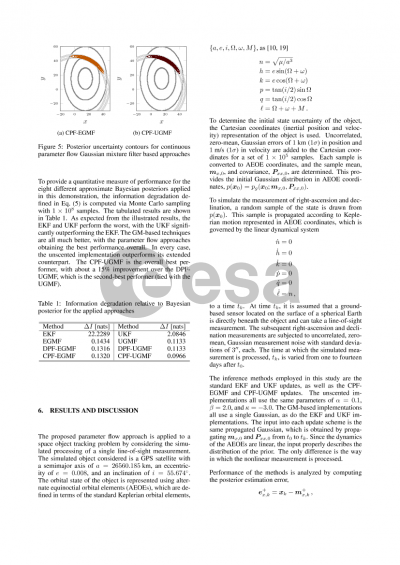
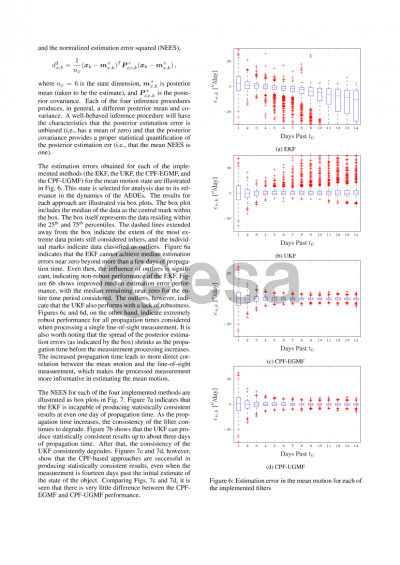
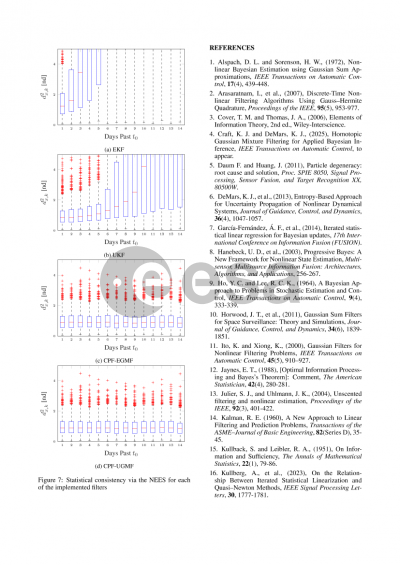
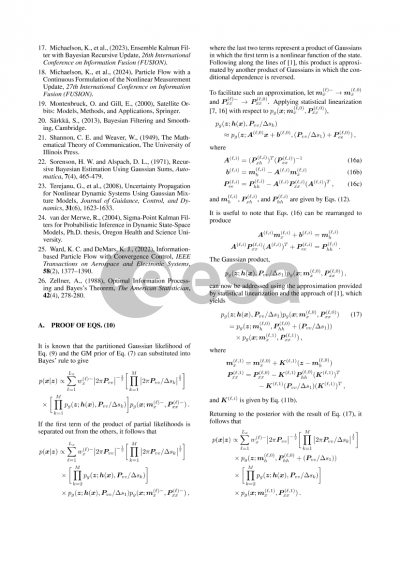
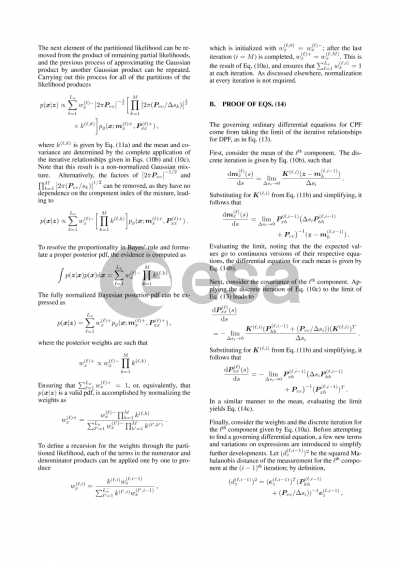
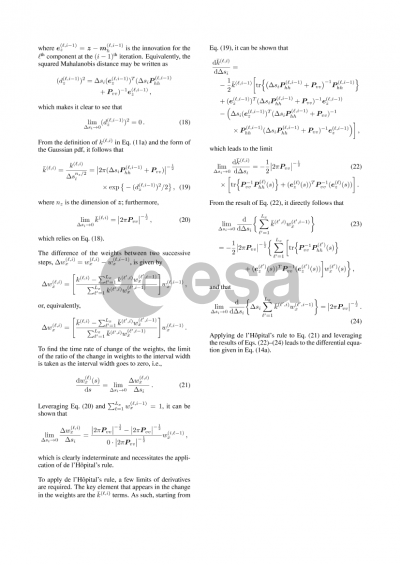