Document details
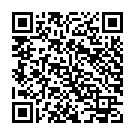
Abstract
Orbit prediction, an essential aspect of collision avoidance is reliant upon data retrieved from near earth observatories and processed through pipelines, sometimes, to generate visualizations, such as that presented by ASTRIA (https://astria.tacc.utexas.edu/AstriaGraph/). While such data pipelines often treat retrieved data equally, there is a great deal of variation in the quality of the retrieved data which impacts the orbit calculations in various ways. We analyze six years of nightly data downloads (2018-2023) retrieved from the Joint Space Operations Command (JSpOC), and characterize it with respect to various characteristics including its age (time since retrieval), frequency of observations for individual objects, changes to observed objects, data format changes, and object count in each download.
While the data visualization functions reliably, with a consistently growing number of tracked objects, a closer picture reveals several points where the retrieval-processing-visualization pipeline could be optimized and the details fine-tuned based on the data quality analysis. While the number of objects tracked has increased over time, this increase is not evenly distributed. While new objects are added, old ones cease to be reported. While the nightly download reports all tracked objects, the frequency of observation varies–some tracked objects have been reported only a few hours before (perhaps, indicating that these are observed multiple times a day), other objects are not observed daily and some are observed up to once every thirty days. This indicates that orbit calculations based on fewer observations could be less reliable than those based on daily tracks. Such disparities could be included in the orbital visualisations as well. Furthermore, this disparity also presents opportunities for optimizing the data retrieval pipeline to capture more observations that could potentially be missed due to the daily retrieval cycle.
We also assess the impact of data quality issues on integrating data longitudinally over longer time periods as well as data from multiple sources to enhance the cohesion and reliability of orbit prediction and data visualizations.
Preview
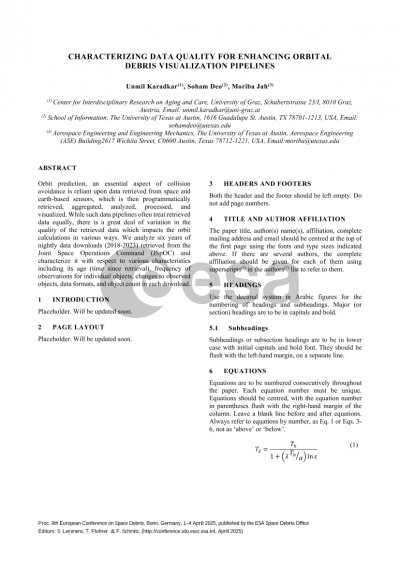
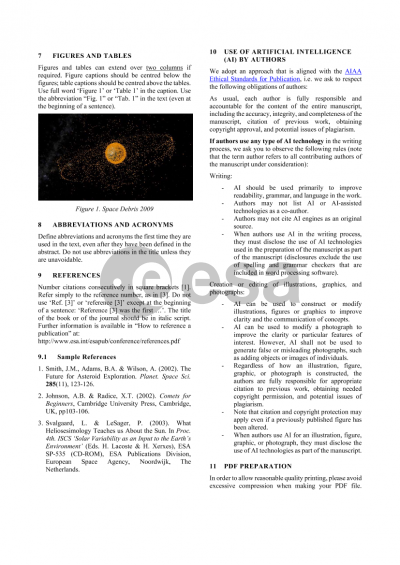
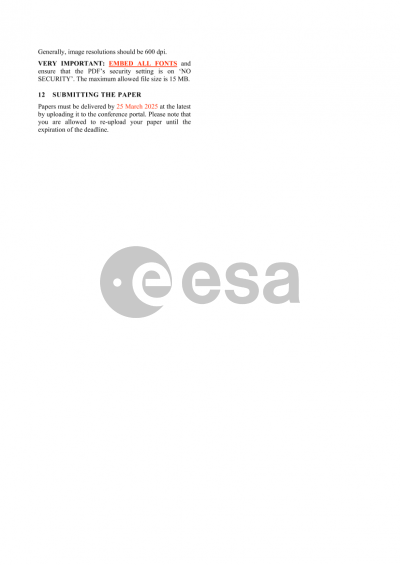