Document details
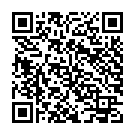
Abstract
As space activity grows exponentially, the orbital environment is becoming increasingly congested with active satellites, inactive spacecraft, and debris of varied sizes and shapes. It has become evident that, in addition to precise monitoring of the debris population, active space debris removal is essential to ensure long-term access to Earth's orbits, requiring detailed knowledge of each object's orbit, shape, and rotational state.
Despite the significant efforts by space surveillance networks to monitor and track a growing number of objects, the available data often consists only of positional information, leaving critical gaps in understanding the physical and rotational characteristics of defunct satellites and space debris. To bridge this knowledge gap, we are leveraging data from the VST/OmegaCAM archive, a unique dataset of over 400,000 high-precision observations spanning 12 years. While VST was designed for deep-sky surveys, space debris cross its field of view, leaving detectable traces in the images. The instrument's exceptional sensitivity allows us to detect objects as small as 5 cm in low-Earth orbit (LEO) and 30 cm in geostationary orbit (GEO). Processing this extensive archive requires advanced image analysis, for which we have developed a novel streak-detection method that combines a convolutional neural network with a Hough transform layer. We present our training dataset, algorithm design, and streak-detection workflow and discuss the detections realised with our algorithm on a set of OmegaCAM images. We discuss the performance of the algorithm in terms of completeness, sensitivity and precision.
After detection, each streak is correlated with the catalogue of known orbital objects, and photometric reduction is applied to extract light curves, providing insights into the object's attitude and shape. The intensity profile of a streak is retrieved by placing a series of rectangular apertures along the streak and measuring the flux received from the target in each aperture. The retrieved flux is calibrated against photometric reference stars from GAIA DR2 with magnitude conversion and error rejection processes. This approach yields high-precision absolute magnitudes with errors of a few hundredths of a magnitude, enabling detailed characterization of non-resolved objects. Fourier analysis is applied to retrieve, when relevant, rotation rates of objects, providing valuable information about the operational status of the detected objects and potential retrieval possibilities.
All data processed through our detection and reduction pipeline will be made available in an open-access data repository, offering the community a valuable resource for further investigation into the orbital debris population. Beyond supporting active debris removal efforts and enhancing knowledge about individual debris objects, this work will also enable deeper insights into key aspects such as the increasing interference with astronomical observations and a more accurate understanding of the size-frequency distribution of orbital debris.
Preview
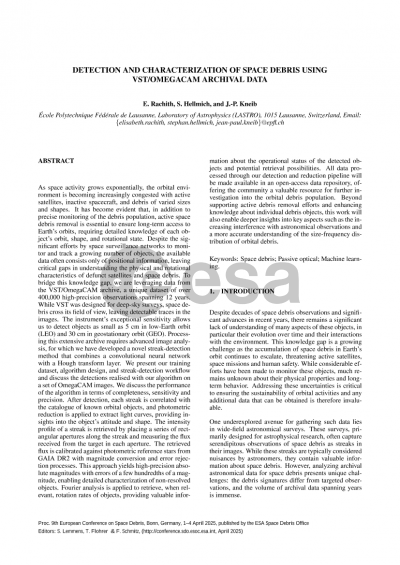
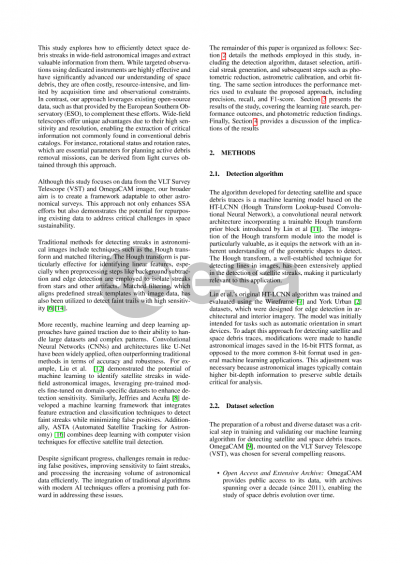
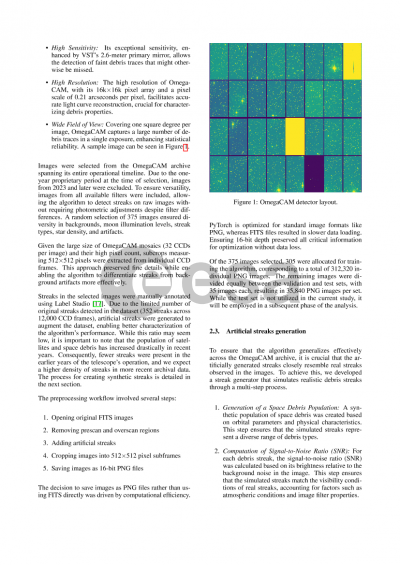
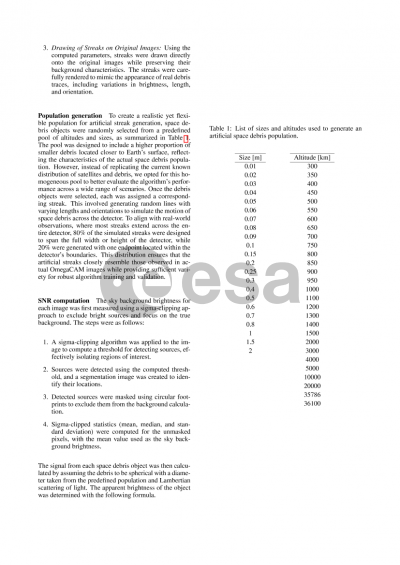
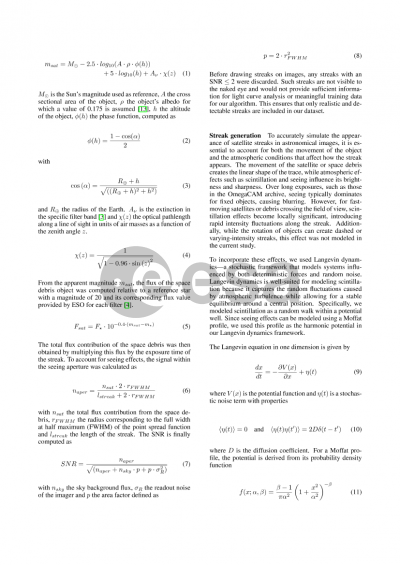
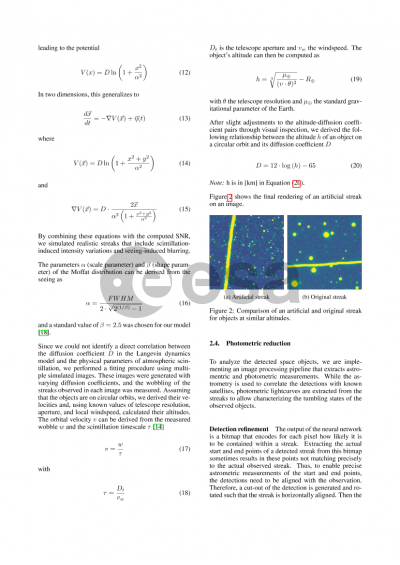
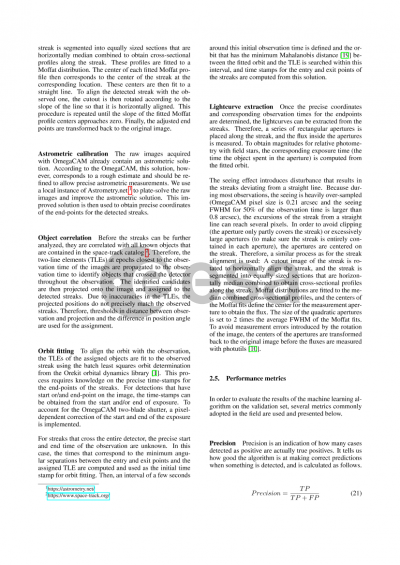
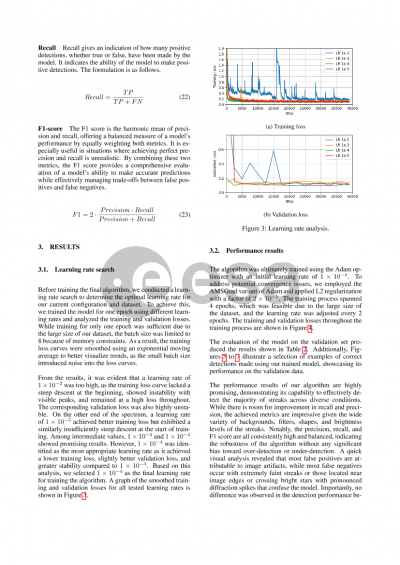
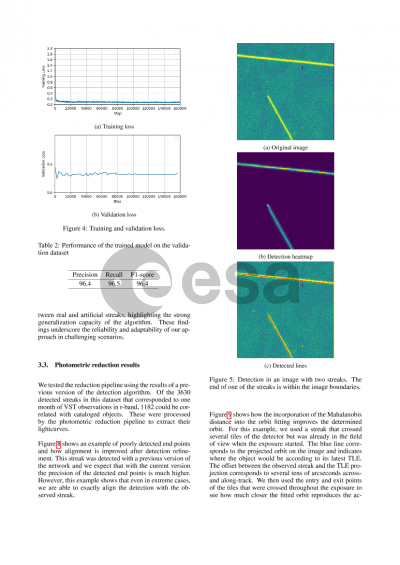
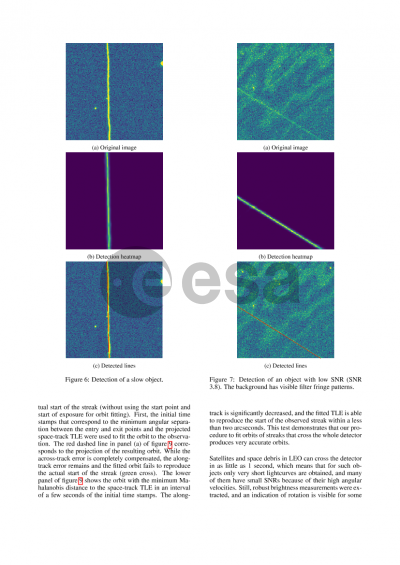
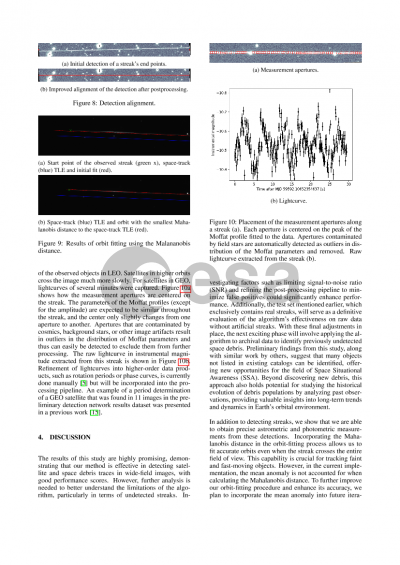
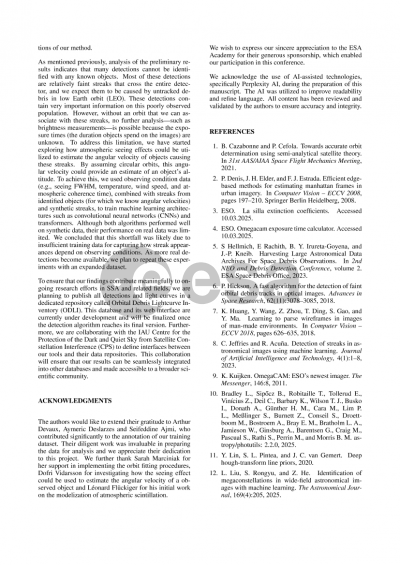
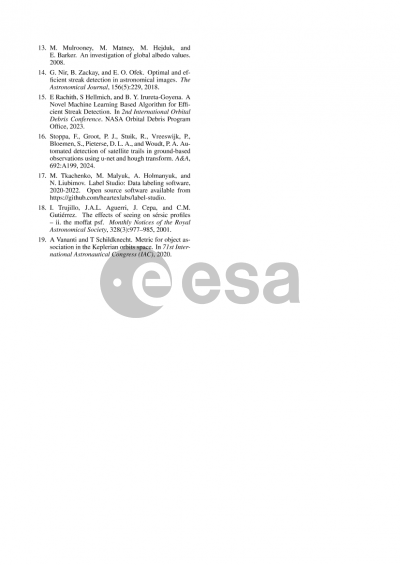