Document details
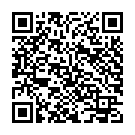
Abstract
The sustained increase in launch traffic, the deployment of large constellations, and the ever-growing population of space debris are putting significant strain on Space Situational Awareness (SSA) and Collision Avoidance (CA) activities. Current CA procedures involve high levels of interaction between different entities and human intervention. On the one hand, space surveillance and tracking is normally performed by entities other than the satellite operator. Coordination between operators for close approaches between active satellites is also paramount, as evidenced by the potential conjunction between ESA’s Aeolus and SpaceX’s Starlink 44 satellites on 2 September 2020. On the other hand, even if an automated initial screening is performed, the final decision for the potentially dangerous close approaches relies on human operators. A ‘business as usual’ strategy will not be sustainable as space becomes more congested, which has triggered different initiatives to increase automation like ESA’s Collision Risk Estimation and Automated Mitigation (CREAM) [1]. One of CREAM goals is to explore on-board CA capabilities, including autonomous decisions-making without the ground segment. However, several key technological advances are required to enable these applications.
Researchers from the ERC-funded COMPASS project at Politecnico di Milano have recently introduced the Manoeuvre Intelligence for Space Safety (MISS) software tool [2] for the analysis and design of Collision Avoidance Manoeuvres (CAMs). MISS relies on analytical and semi analytical methods, which leads to highly efficient and relatively simple algorithms. While these characteristics make MISS suitable for on-board use, past works have not dealt with the decision-making component needed for autonomous operations. In this paper, the synergies of MISS with machine learning techniques for the design of autonomous on-board CA algorithms is explored. A clear separation into two modules allows us to leverage the advantages of each approach: the decision-making module relies on machine learning, while CAM design is performed through the highly efficient semi analytical models. Particular attention is paid to the training of the decision-making algorithm and the challenges of obtaining historical CAM datasets that are large enough for this task, which requires the generation of synthetic ones. The future implementation of on-board CA capabilities would also require the establishment of federated SSA services feeding the spacecraft with information about nearby objects: although the design of such system falls out of the scope of this work, the basic inputs that would be required from it are identified. Finally, the application to a potential in-orbit demonstration mission is also discussed.
References
[1] Bastida Virgili B., Flohrer T., Krag H., Merz K., Lemmens S., “CREAM - ESA's Proposal for Collision Risk Estimation and Automated Mitigation,” First International Orbital Debris Conference (IOC), Sugarland (TX), US, 9-12 Dec. 2019. Paper 6031.
[2] Gonzalo J.L., Colombo C., Di Lizia P., “Introducing MISS, a new tool for collision avoidance analysis and design”, Journal of Space Safety Engineering, Vol 7, pp. 282-289, 2020. https://doi.org/10.1016/j.jsse.2020.07.010
Preview
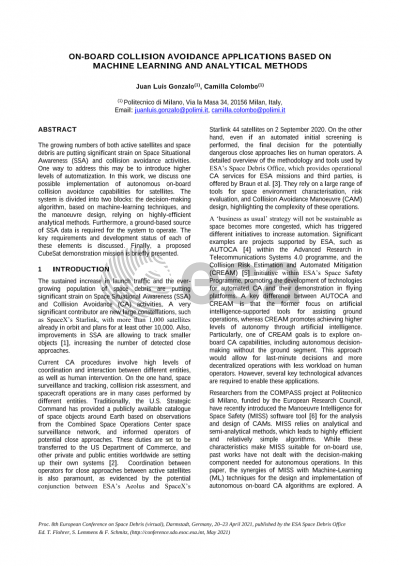
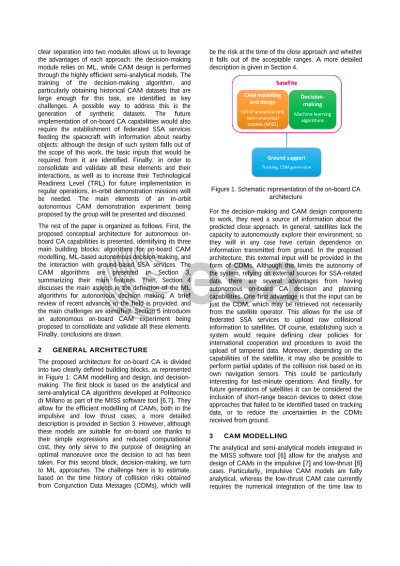
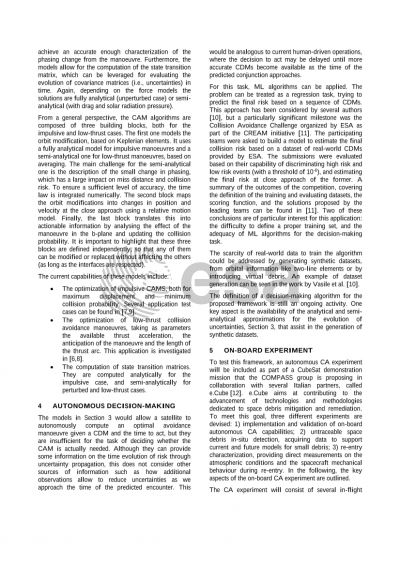
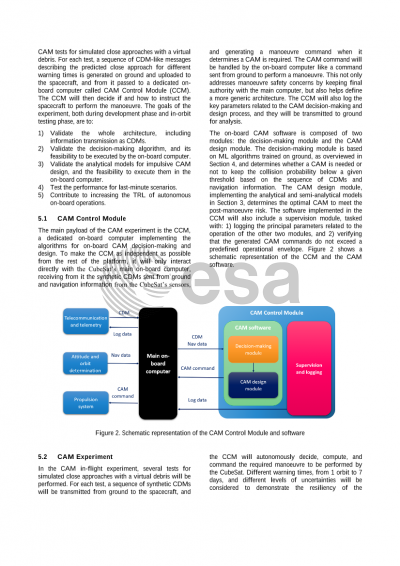
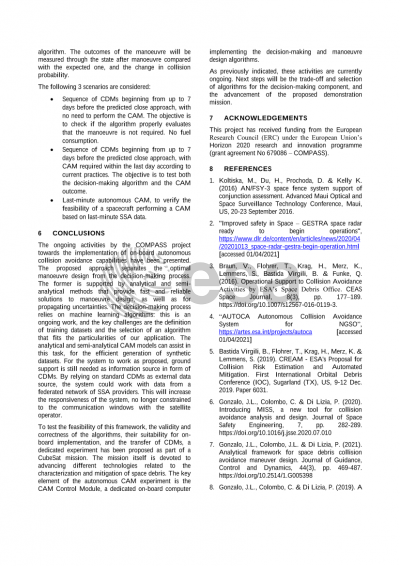
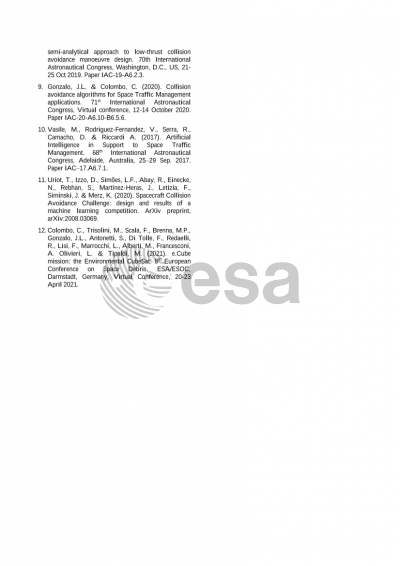