Document details
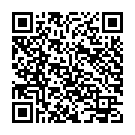
Abstract
In order to keep a safe access to space in the coming years, it will be necessary to remove space debris such as non-functional spacecraft or abandoned launch vehicle stages from Low Earth Orbit (LEO) region.
To make space debris removal missions more commercially viable, planning is required on how to remove multiple pieces of space debris in one mission. This approach can reduce the overall launch cost and help remove space objects efficiently.
This paper addresses the mission planning problem to enable a single spacecraft to efficiently rendezvous and safely deorbit multiple space debris. The problem mixes combinatorial optimization to select the debris among a list of candidates and functional optimization to determine the minimum-fuel and/or minimum-time orbital manoeuvres to transfer the Servicer satellite to the target debris orbit and bring it back to a disposal orbit. Finding the optimal solution is challenging because of the large number of candidate space objects to be considered and the variety of their orbital parameters, as well as the technical/operational constraints to be incorporated in the optimization problem (e.g. propulsion system duty cycle, visibility, etc.).
In this study, a two-step strategy has been proposed to tackle the above problem. First, the Machine Learning method has been used to train an Artificial Neural Network (ANN) to quickly estimate the cost of transfer and disposal of a debris object. This approach allows the evaluation of delta-V and time-of-flight of many potential debris at a modest computational cost. Then, the full sequence of debris, together with the individual trajectories, are optimised using Fujitsu’s Quantum Inspired Optimisation Services (QIOS). To this purpose, the problem is formulated as a quadratic unconstrained binary optimisation (QUBO) problem in order to be handled by Fujitsu’s quantum-inspired Digital Annealer technology, which has been built to efficiently solve complex combinatorial optimization problems.
To show the effectiveness of the proposed approach, a realistic debris removal scenario has been defined in collaboration with Astroscale, a worldleading debris removal company, through the capabilities of Astroscale’s commercial end-of-life servicer that is designed to remove multiple failed satellites from orbit.
This research has been carried out as part of the UK Space Agency grant “Advancing Research into Space Surveillance and Tracking: to assess how to make space debris removal missions more commercially viable”. The project leverages ANN-based trajectory design algorithms developed by the University of Glasgow (UoG) and Fujitsu’s Digital Annealer and Quantum Inspired Optimisation Services to solve the optimisation problems associated with ADR mission planning design. Amazon Web Services provides Cloud and AI/ML tools and services to support the project with access to open data sets. Astroscale, the world leader in debris removal technologies, is providing the end-use case as a representative user of multi-target mission optimisation.
The goal of the project is to deploy and combine, for the first time, existing and working technologies, providing excellent commercial and/or scientific opportunities. The development of such innovative project, being driven by the unique combination of the consortium’s technologies and knowhow, can be beneficial for private and public space organizations delivering space debris removal technologies (reduced mission time and fuel usage, allowing either lower launch mass or more objects captured).
Preview
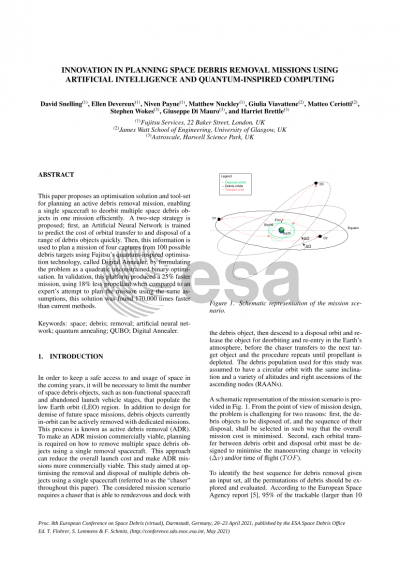
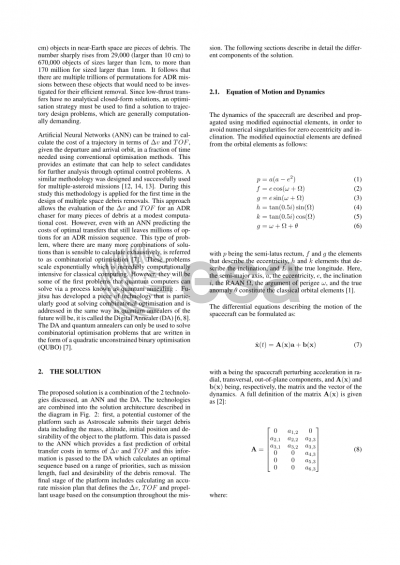
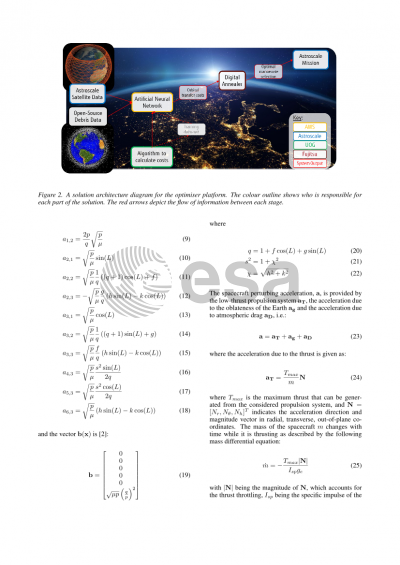
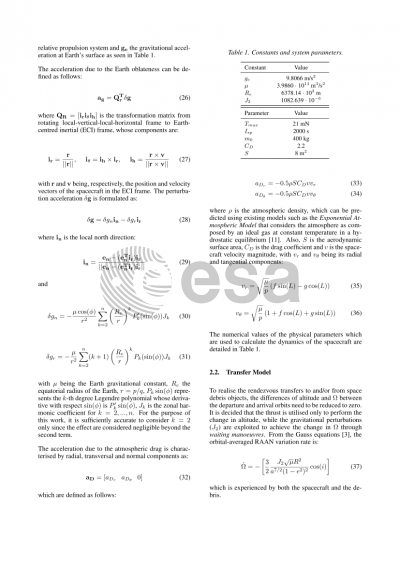
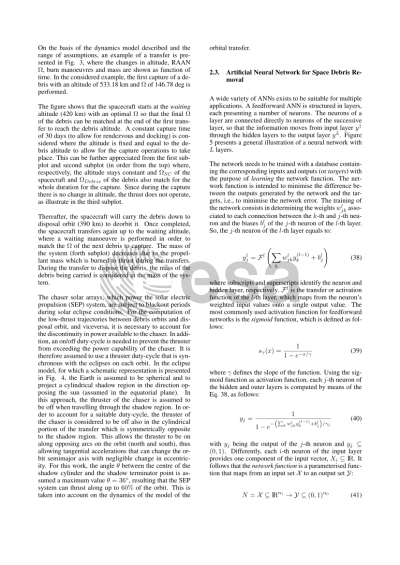
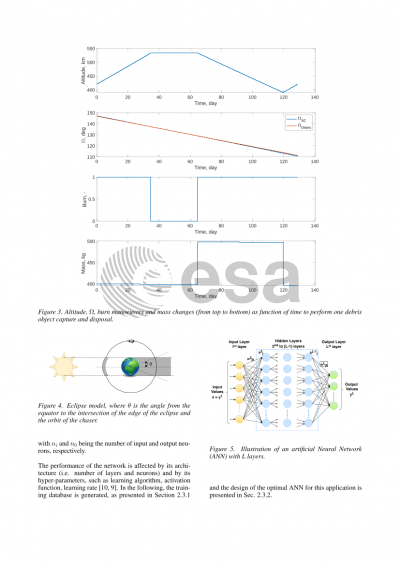
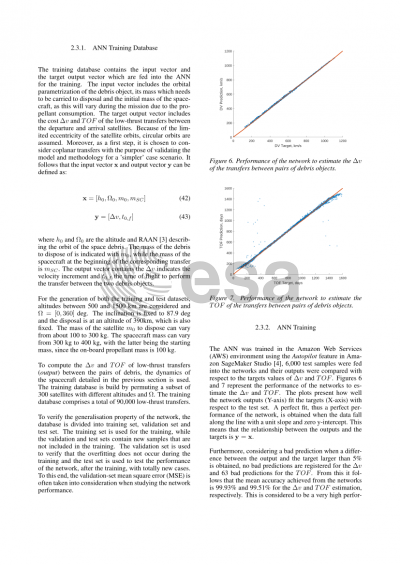
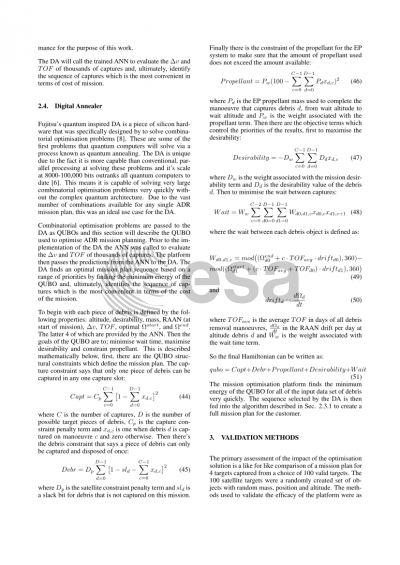
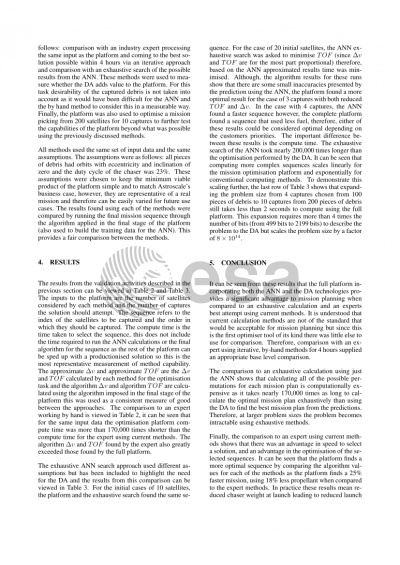
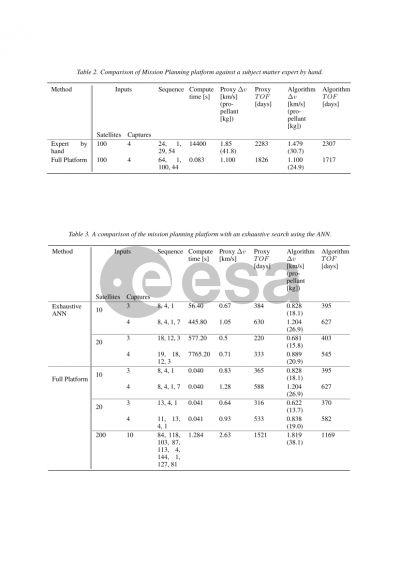
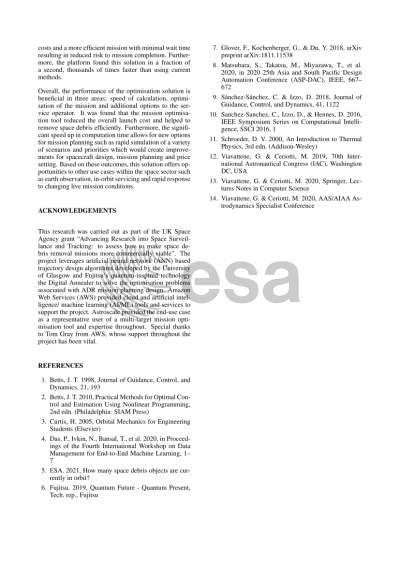