Document details
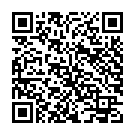
Abstract
Space surveillance can be defined as the ability to observe, detect, catalogue satellites maneuvers and map atypical behaviors. This project focuses on the prerequisite necessary to achieve the mapping.
Taking advantage of TLE (Two-Line Elements) open source data, available within space catalogues, a generic and automatic method to identify satellite maneuvers has been built.
This study has used TLE data for satellites from which maneuvers data were available (CRYOSAT 2, ENVISAT, HYLAS 2, JASON, JASON 2, JASON 3, SARAL, SENTINEL 3A, SENTINEL 3B, SPOT 2, SPOT 4, SPOT 5, TOPEX / POSEIDON).
The maneuvers information’s have only been used to verify later results. Indeed, to guarantee the genericity and automaticity characteristics, the method has to be developed without any maneuver supervision knowledge. Moreover, as this project, is within a big data environment, everything shall be automatic.
It is within this context that the potential of different options was explored, from the historical MWCF algorithm, passing by different types of clustering techniques DBSCAN then OPTIC. Even if these techniques have presented encouraging results, they did not allow to answer the expected: a generic and automatic method. Indeed, they have always some parameters to be manually scaled.
Moreover, while looking at the temporal evolution of the semi-major axis (derived from the mean motion) a clear coherence of its inflexion with the maneuvers could be observed. Detecting maneuvers could then be simplified as detecting certain inflexion points of the curve.
In this view, a new methodology has been developed, that embodies a cross-fertilisation between physics and data mining.
It first isolates all inflexion points within the semi-major axis time evolution. Then, following the aspect of the curve different types of filters are applied to remove the noise and keep only what is expected to be maneuvers. Within the set of satellites of this study, it has been observed that only up to 3% of the temporal evolution of the semi-major axis are maneuvers. To secure the detection it has been double to 6% and this was used as an hypothesis to calibrate filters to the correct level.
With a 5 days lag authorized between the real maneuver and their detection, very good results were obtained for most of the satellite. Except HYLAS 2 and TOPEX / POSEIDON, results of the maneuvers detection up to February 2020 (end of our extracted data) are: F1 score 81% (51 to 94%), recall 88% (74 to 100%), precision 77% (34 to 96%) and error rate 0.6% (0.09 to 1.47%). This is a sufficient level to consider the method robust enough to fulfil our objective. Let’s mentioned that some maneuvers were grouped together in the calculation as they were within the same 5-days detection window. It shall also be mentioned that the method required no parameter at all.
Regarding the two other satellites, the method has been completed by a mechanism to automatically disable analyses when the level of noise is too important to obtain robust results for the all life of the satellite (HYLAS 2) or for portions (TOPEX / POSEIDON).
Preview
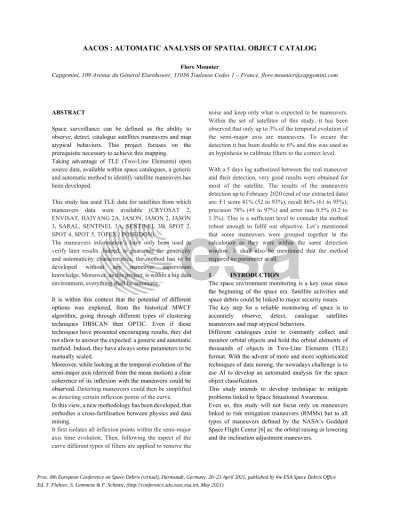
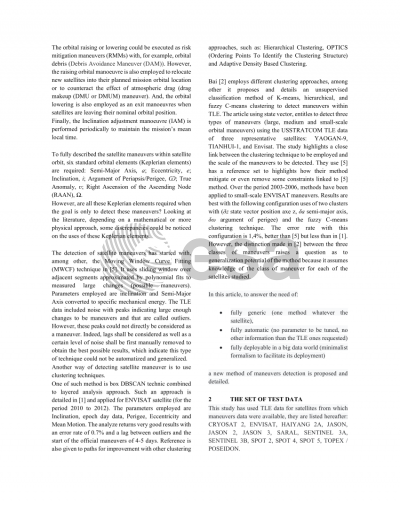
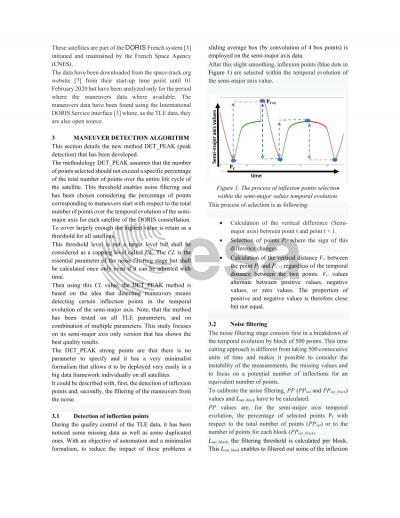
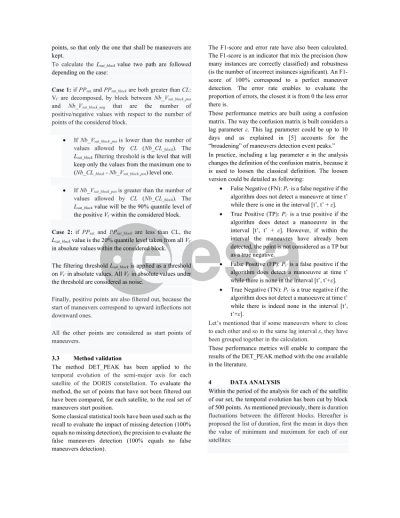
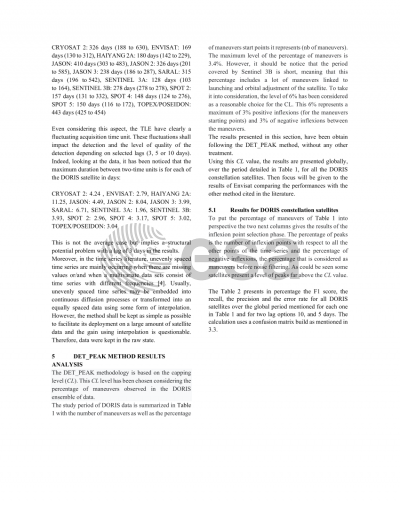
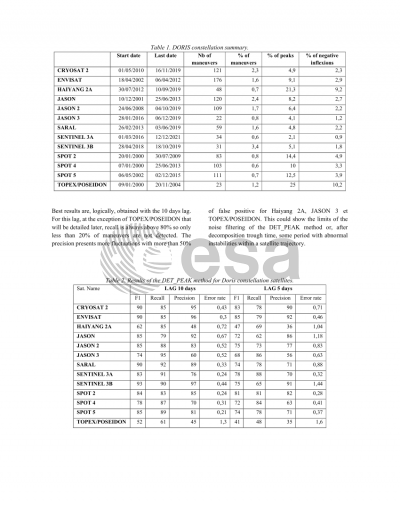
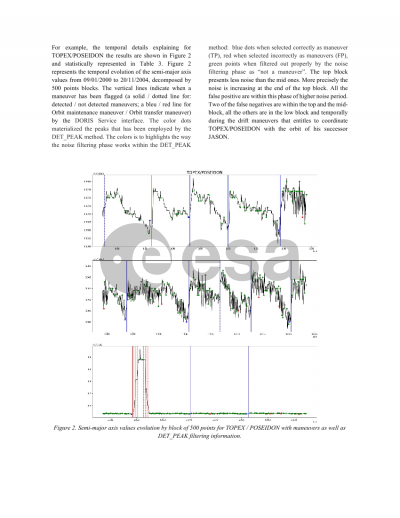
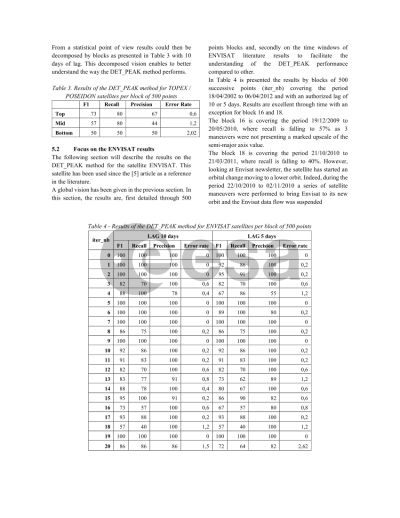
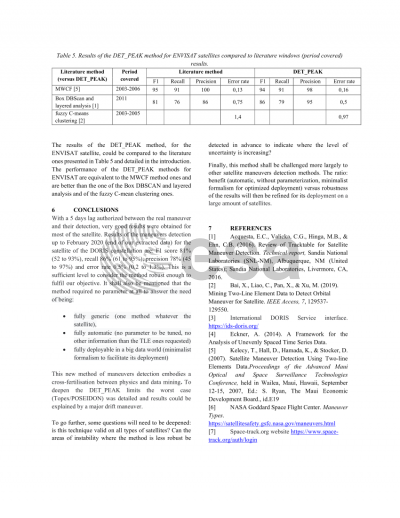