Document details
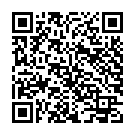
Abstract
One of the natural by-products of the radar measurements is the Radar Cross Section (RCS). It is given as a sequence in time and is a measure of the radar reflectivity of an object from the point of view of the radar installation. There is a lot of information about an object implicitly contained within the RCS sequence it generates. However, that information is somehow embedded and is not straightforward to extract. The evolution of the RCS differences contains information on the radar scattering characteristics of different viewing perspectives on the spacecraft. The characterization of these signals relies on the non-isotropic nature of the radar scattering from an object with respect to its attitude towards the line of sight of the radar.
The RCS sequences are exploited in two ways within the context of the project. Firstly, the attitude stability mode of the RSO is extracted, that is, classifying whether an object is stable, tumbling, etc. Then, based on the same information, a more fine-grained classification algorithm is developed, being able to associate each RCS sequence with the corresponding object from a corpus of known spacecraft. In both cases, at the core of each classifier, a Machine Learning (ML) algorithm is responsible for the characterization, with additional advanced pre-processing steps that increase the performance of the ML model.
The combination of both classifiers allows for the continuous tracking of the evolution of the attitude stability of a set of spacecraft using only passively obtained information from the radar installation. That is, given an RCS sequence, the knowledge of the owner RSO and its current and past attitude stability mode allows for a close following of the evolution of the stability of objects.
There is great value in this application for the RCS, as it is the minimum output of a radar installation. Currently object identification based on radar measurements relies on correlation of the orbital track detected by the radar and a library of continuously updated orbital tracks. This application would reduce the reliance of said track library and provide independent identification directly by the radar installation, with the added benefit of providing simultaneously the attitude stability of the identified object.
Different ML algorithms are trained with real data obtained with the Surveillance Radar (S3TSR), which is part of the Spanish Space Surveillance and Tracking (S3T) programme. The results obtained are systematically compared to measure the accuracy of the ML algorithms.
Preview
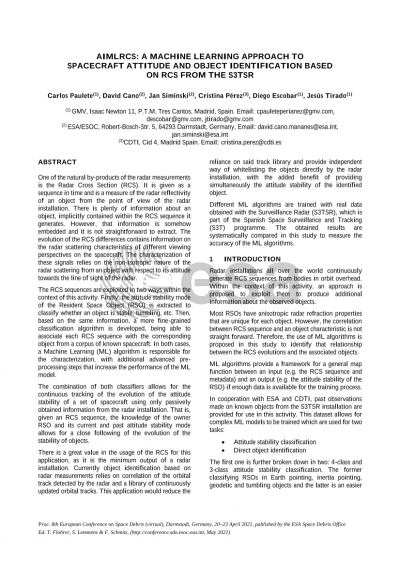
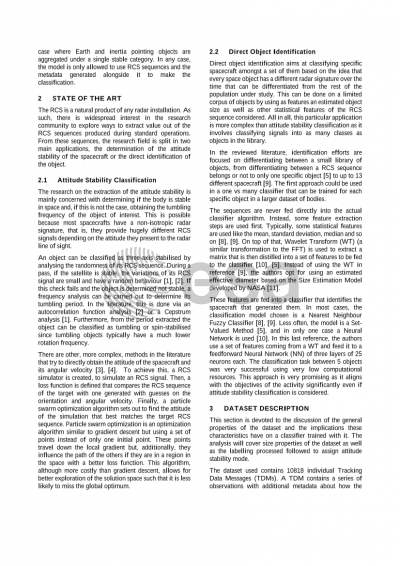
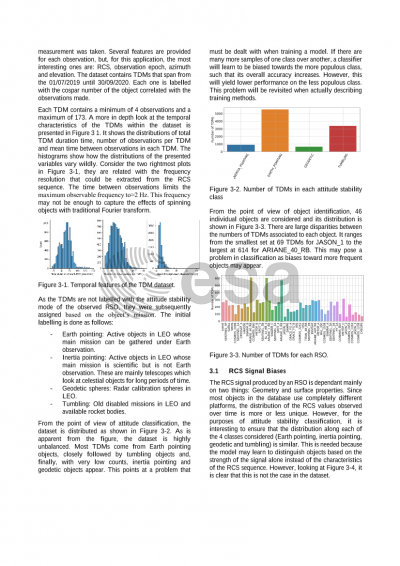
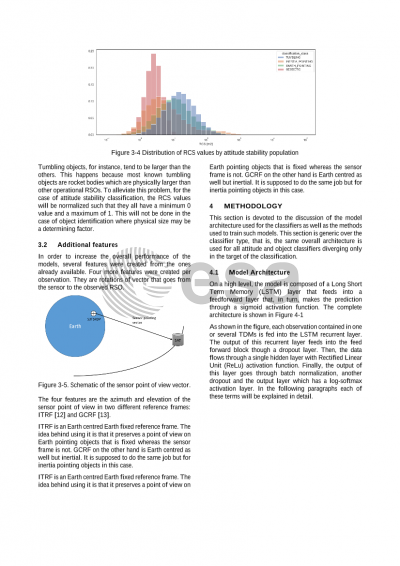
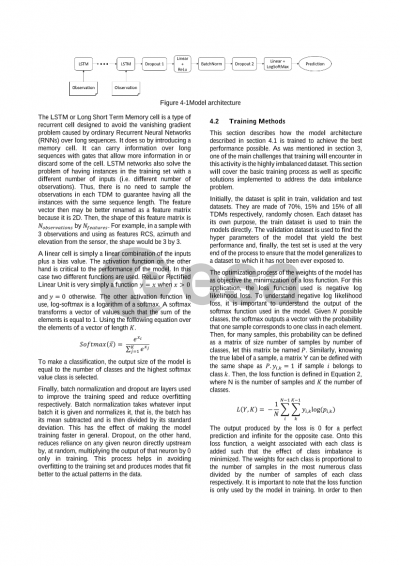
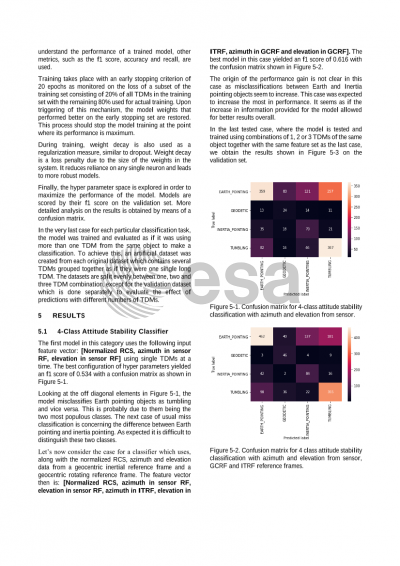
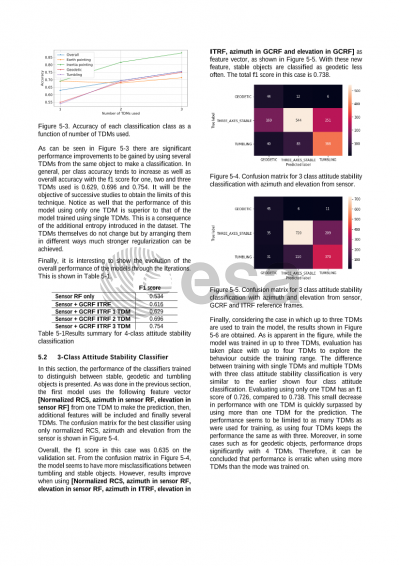
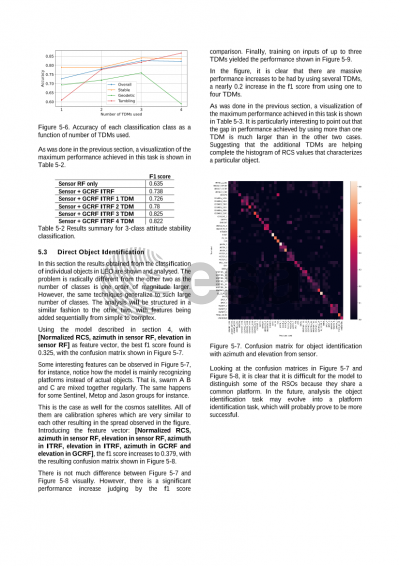
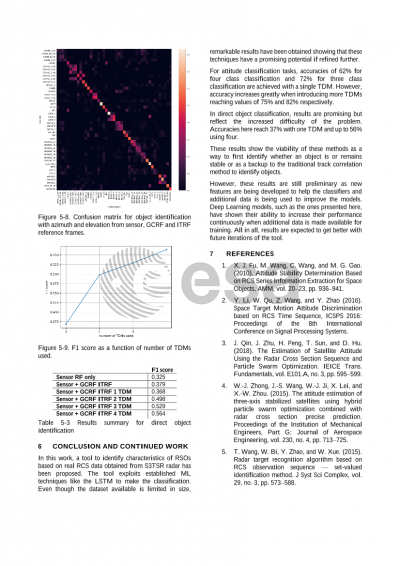
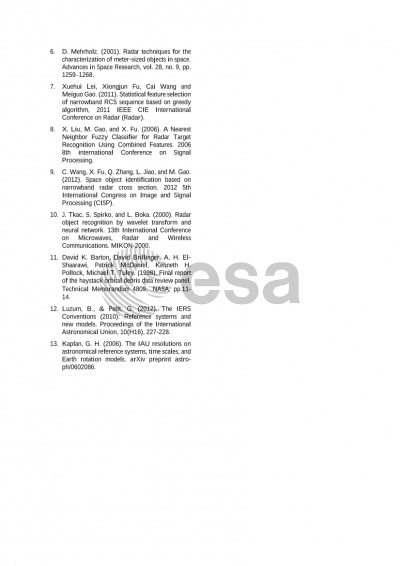