Document details
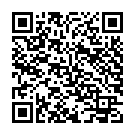
Abstract
This paper addresses the problem of automatically allocating collision avoidance manoeuvres, in the context of space traffic management, under uncertainty. The paper will present a robust Bayesian framework to predict the occurrence of a collision and allocate one or more collision avoidance manoeuvre. The robust Bayesian framework simulates the whole pipeline that starts from some prior distribution of the state of two or more objects, propagates the associated uncertainty, updates the state estimation with Bayesian inference using new observations and generates robust optimal manoeuvres. The manoeuvre is redefined as new observations are acquired and the metrics defining the occurrence of a collision are updated.
The term robust refers to the definition of manoeuvres that are guaranteed to avoid a collision under uncertainty in the prediction of the probability of collision. This uncertainty comes from nonlinearities in the dynamics, model uncertainty and uncertainty in the measurement likelihood and prior distribution. Through this robust Bayesian framework, we will show how to simulate the generation of CDMs (Conjunction Data Messages) and how to define robust manoeuvres in the case of uncertainty in the correctness of the CDMs. We will consider both the case in which a single manoeuvre is used to avoid a single collision and the case in which one or more manoeuvres need to be allocated to avoid subsequent collisions.
Building on previous work by the authors (see Sanchez and Vasile Acta Astronautica 2020) we will present an artificial intelligent agent that provides suggestions on whether to implement the manoeuvres and when. The agent is trained on a wide range of simulated scenarios and uses multiple indicators to infer the need to implement a collision avoidance manoeuvres, including the probability of collision and the associated uncertainty.
Preview
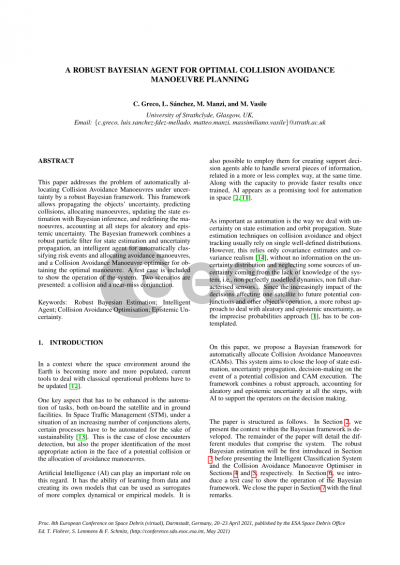
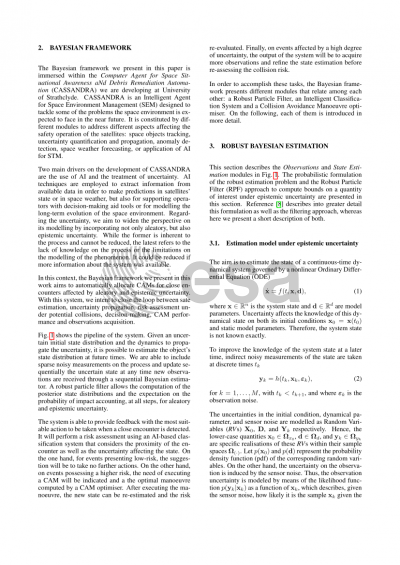
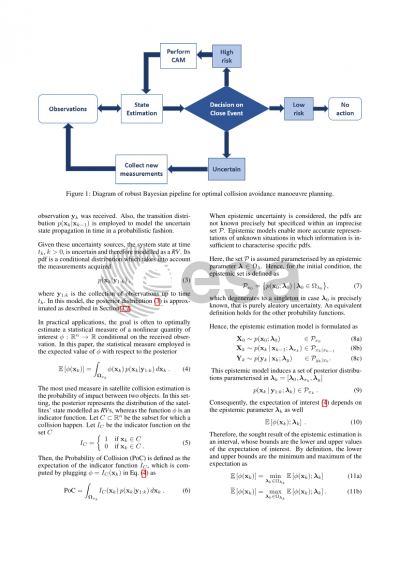
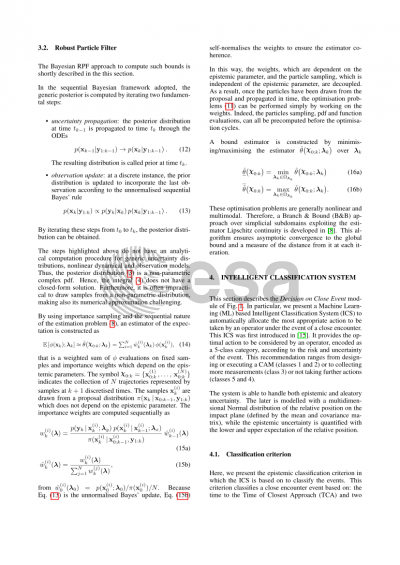
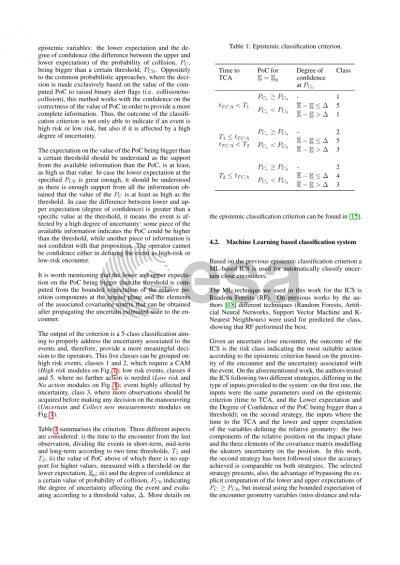
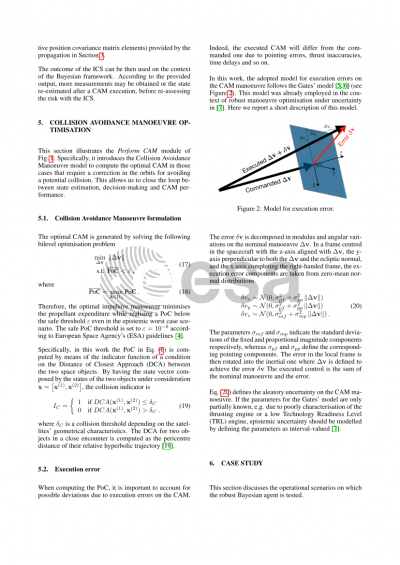
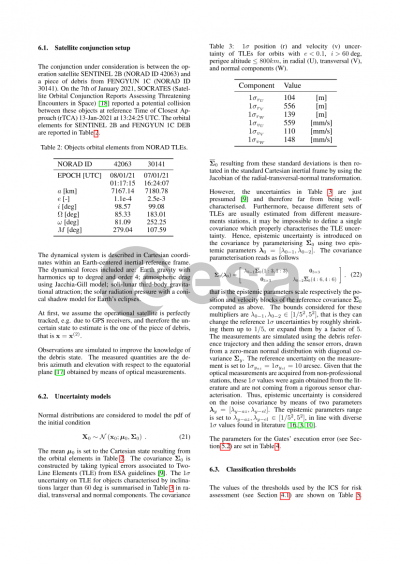
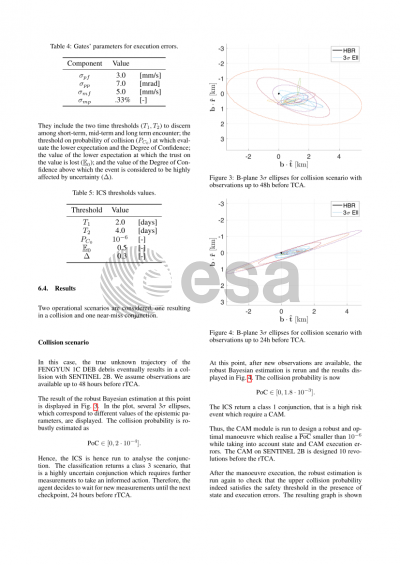
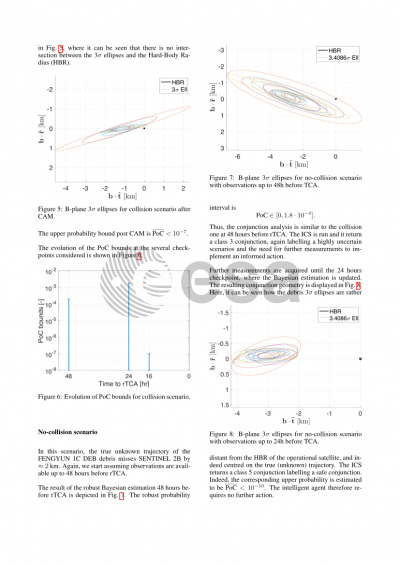
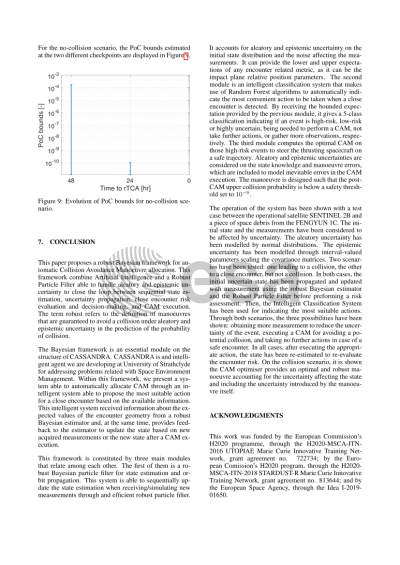
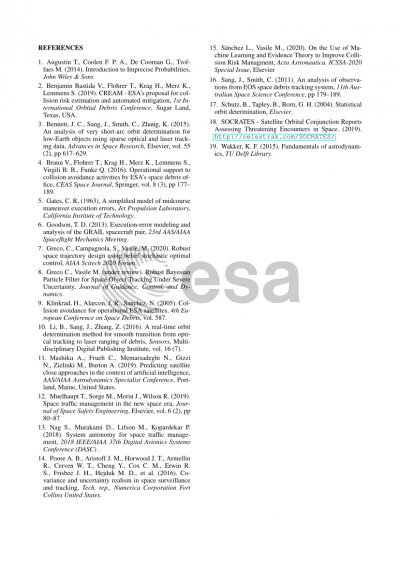