Document details
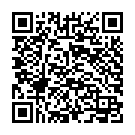
Abstract
Introduction:
Nearly six decades after the beginning of the space activities, the concerns for the uncontrolled re-entry of intact manmade objects is growing, gaining attention in the international scenario. Current estimations predict the uncontrolled re-entry of 1-2 large objects per week, with the possibility of impacting fragments on the Earth surface.
In order to deal with this issue, spacecraft-oriented software for the re-entry modelling and estimation of the survivability of generated fragments have been implemented, but the complexity of the aerothermal phenomena involved in the atmospheric re-entry process results in considerable costs in terms of computational time.
In this purpose, low-fidelity software, which use simplified spacecraft shape and atmospheric re-entry model partially based on re-entry observations, are used to provide faster but less accurate predictions during the last phase of the object lifetime. Motivated by the idea to improve these simplified models and following the successful ATV controlled re-entry observation campaign in 2008, many studies are now focusing on collecting break-up information by mean of in-situ observations.
Nevertheless, uncontrolled re-entries, especially in case of Highly Eccentric Orbits (HEO), for which minor uncertainties in the initial conditions for the atmospheric re-entry could led to relevant differences in the break-up evolution, are difficult to observe.
The proposed study uses machine-learning techniques which exploit the spacecraft-oriented results to model the break-up evolution for the uncontrolled re-entry of objects in HEO orbits, with the final purpose to use this information to simulate what possible observations could be obtained from ground networks.
Methodology:
The methodology used for this work is described by the following steps:
A preliminary break-up scheme for the re-entering body is obtained observing the results of a first set of simulations. In this phase, the event which better characterizes the main relevant break-up, like for example the first tank separation, is identified.
Spacecraft-oriented software (SCARAB) is used to define a database, which contains the break-up information for a selected number of relevant configurations.
Several machine learning algorithms are then applied to define a model for the break-up scheme.
Once defined, the model is able to mimic the break-up altitude process of new input configurations within minutes.
Test case:
The test case used for this study is the ESA CLUSTER II spacecraft. ESA CLUSTER II mission, launched in August 2000, focus on understanding the solar wind-Earth’s magnetosphere interaction and it is composed by four identical spacecraft, which fly on HEO in tetrahedral formation.
Conclusion:
The proposed methodology provides an efficient approach to quickly estimate the break-up evolution of objects orbiting on HEO during the very last phase of uncontrolled re-entries, for which fast computations are crucial. These information can be then used to detect a re-entry in observations, during the predicted re-entry window of an object.
Preview
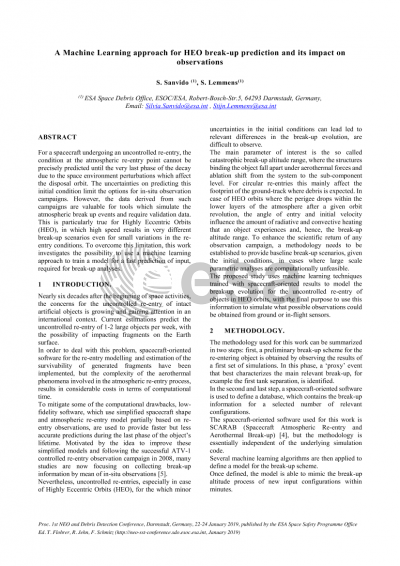
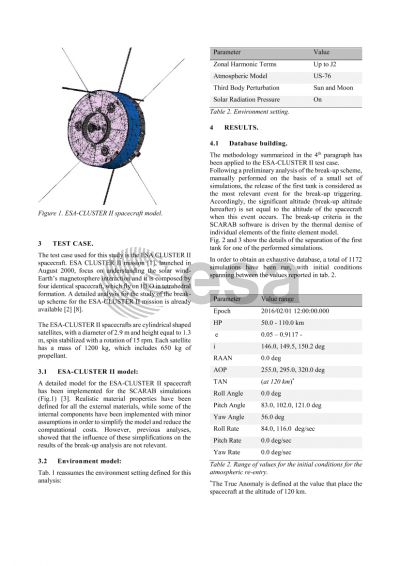
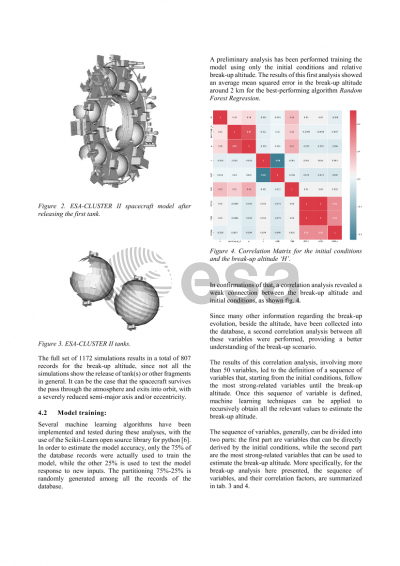
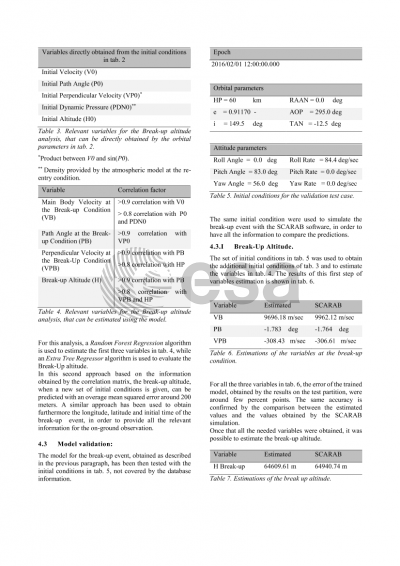
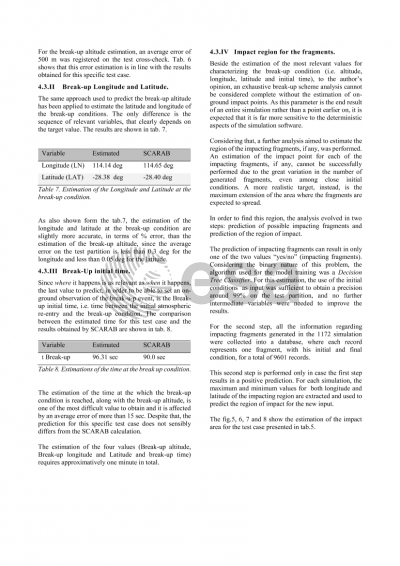
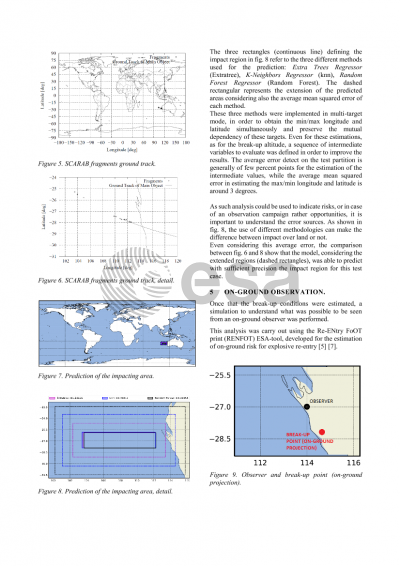
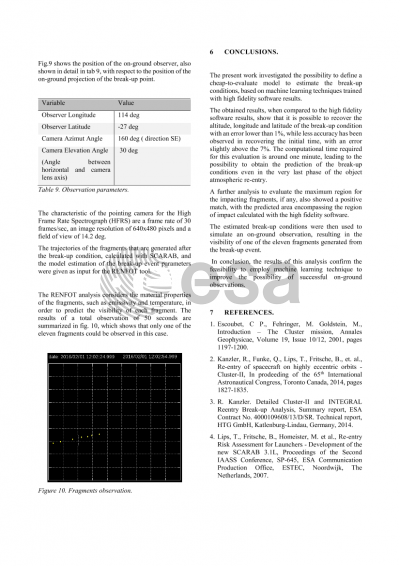
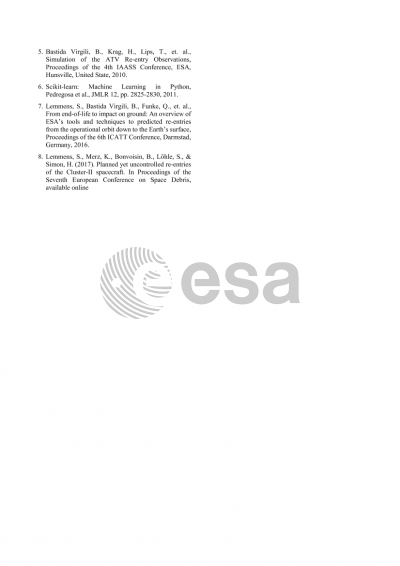